New Data Shows Why AI Governance Must Start Before You Build Anything
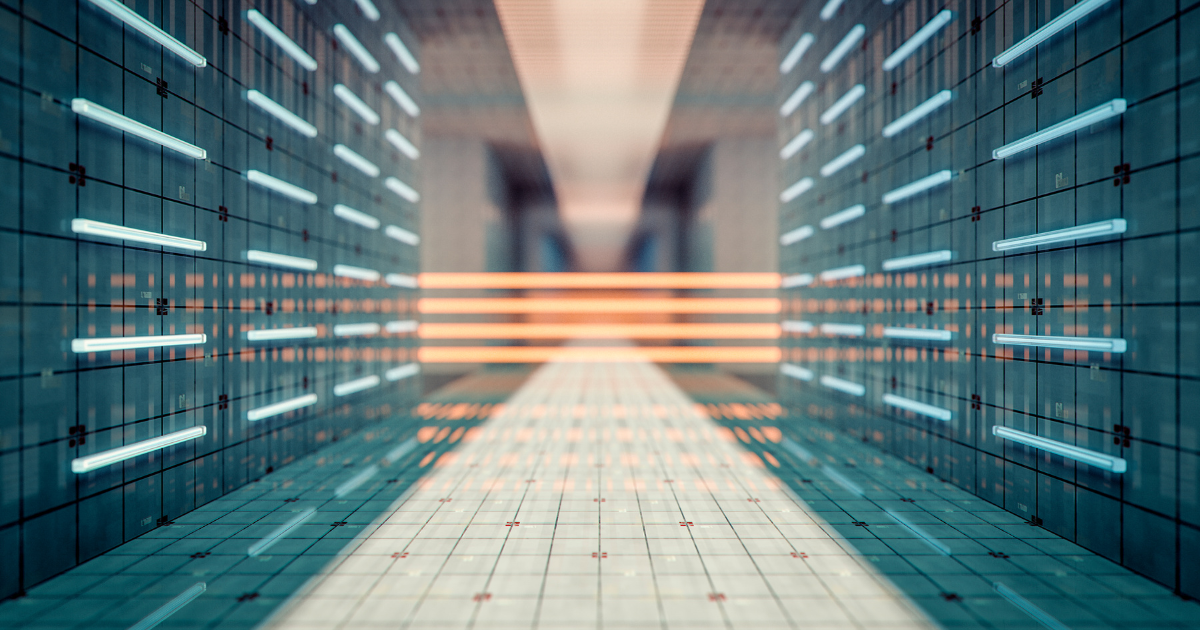
By the time your generative AI model is ready for production, it might already be too late to govern it properly
A large wave of new enterprise AI projects is on its way. More than 80% of organizations in North America have at least 51 use cases in the pipeline, a survey published this week by Corinium and ModelOp has found.
The extent to which those use cases deliver value, however, is heavily influenced by time to market. Our data suggests that, despite massive enthusiasm and multi-million-dollar investments, roadblocks during production are hampering ROI.
For the majority – 56% – a generative AI initiative takes anywhere from six to 18 months to launch. There are numerous reasons for delays, but perhaps the most misunderstood is poor AI governance. This is because too many leaders still view governance as a speed bump when in fact, if done right, it can drastically reduce time to market.
Skip McCormick, CTO of Cornerstone Technologies, says it is a reluctance to start governance early on, rather than the process itself, that causes delays.
“Many teams develop AI solutions independently and only consider governance too late in the process,” he says. “Someone builds a great model, and when they’re ready to put it into production, they suddenly realize it has to comply with model risk management and governance.”
When implemented early, AI governance can streamline model delivery, ensure regulatory compliance, and provide clarity on value creation. Yet only 23% of enterprises have standardized AI intake and management processes, and 36% still use manual processes like spreadsheets and email to manage proposals.
Doing things manually is “like herding cats,” says Jim Olsen., CTO of ModelOp: “Enterprises need to embrace AI lifecycle automation if they want to guarantee policies will be enforced when dealing with hundreds or thousands of AI use cases.”
Here are some key takeaways from our report on how to reduce risk while accelerating time-to-value:
1. Standardize Use Case Intake
Create a central, consistent process for evaluating and approving AI use cases. Avoid reliance on disparate tools and manual workflows. Centralization reduces confusion, speeds up intake, and sets the stage for scalable governance.
2. Automate Documentation and Review
Governance doesn’t have to slow your data science team down. With AI lifecycle automation, documentation can be streamlined without forcing data scientists to become compliance officers.
“It’s hard to get data scientists to stop doing data science and document their models,” says McCormick. “If you don’t capture that information while it’s fresh in their minds, it becomes nearly impossible to get later.”
3. Build a Systematic Model Inventory
Without visibility into what models you’re building, where they’re deployed, and how they perform, you can’t govern effectively. A model inventory is the foundation for traceability, interpretability, and assurance.
4. Enforce Enterprise-Level Assurance
Only 14% of organizations are performing AI assurance at the enterprise level. This is a missed opportunity; enterprise-wide enforcement ensures consistency, accountability, and alignment.
Our report, AI’s Time-to-Market Quagmire: Why Enterprises Struggle to Scale AI Innovation, contains industry benchmarking data from 100 senior AI and data leaders. It highlights key obstacles to adoption and the emerging role of innovation leaders in AI governance. You can read it here.