Establishing a Standard for AI Model Reliability at McAfee: Celeste Fralick PhD
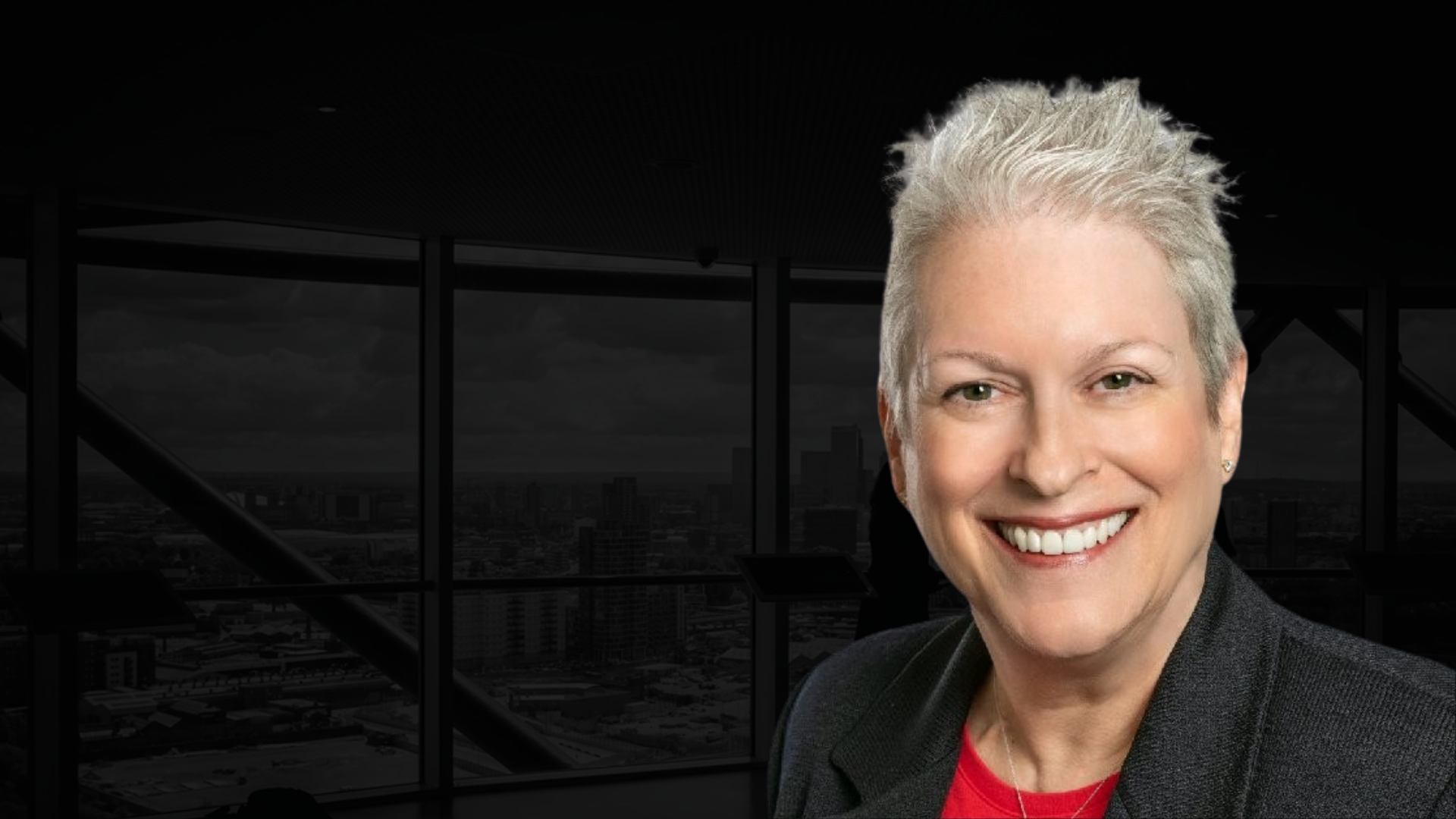
Celeste Fralick PhD, Chief Data Scientist at McAfee, talks about building the anti-virus company’s AIOps function and her ambition to establish an industry standard for predicting how long models will last ‘in the field’
All AI models have the potential to ‘drift’ or degrade over time. But AI systems designed to fight fraud or hackers are unique, in that there are human adversaries out in the world working to subvert them and evade detection around the clock.
The sheer volume of malware in the world today means AI systems are essential in the fight against hackers. But it’s not enough for companies in this sector to just be using AI. They also need mature AIOps functions to monitor models constantly for potential performance issues and guard against adversarial AI attacks.
To discover how anti-virus giant McAfee is approaching this challenge, we caught up with the company’s Chief Data Scientist, Celeste Fralick PhD, ahead of her appearance at Corinium’s 2021 CDAO Fall events.
“Malware is extremely prevalent,” Dr Fralick notes. “As a data scientist, the only way you can take care of it is not just using the rules that most malware companies utilized historically. Everybody has to use some kind of machine learning, deep learning or AI.”
McAfee deals with around 500,000-800,000 cyberattacks every day. As Dr Fralick says, the company’s AI function provides a vital line of defense for enterprise customers and consumers alike.
AIOps and the Fight Against Adversarial AI
Dr Fralick defines the process of developing a model and gathering the baseline data to train it as DevOps. Meanwhile, she views the practice of implementing AI models into a product and managing them in production as being part of MLOps. For her, AIOps refers to all these things at once.
To illustrate just one of the AIOps challenges the constantly shifting cyber threat landscape creates for McAfee, Dr Fralick highlights what she calls the ‘malware labels’ problem.
“One of the challenges that we have in malware, which I don't typically see in too many other businesses, is label changes,” she says. “Your label [for a given virus] will go from, say, ‘benign’ to ‘malware’ to ‘benign’, and maybe back to ‘malware’ again.”
Monitoring AI models for things including changes in the label definitions (i.e., ‘concept drift’), changes in the volume of data feeding into them and signs of ‘data decay’ is vital for ensuring systems perform as intended over time. Creating automated processes to keep track of these things has been a priority for Dr Fralick in recent years.
“The most important thing that you can do, and this is what we've been focused on, is ensure that you have a complete feedback loop,” she notes. “It is critical to ensure those monitors are in place and to ensure that we have thresholds in place that warn you when something is awry.”
“Those are the types of things you want to monitor, as well as bias, explainability and implementing software to prevent adversarial machine learning attacks, which is really model hacking,” she concludes. “[That's] where your adversary can come in and not know what your model is, not know what your features are, and change them.”
Establishing an Industry Standard for Model Decay
Having automated many of the model monitoring processes necessary to ensure the ongoing trustworthiness of McAfee’s AI models successfully, Dr Fralick now has her sights set on a more ambitious goal.
“I was part of the Task Group that developed ISO 24028, which is an AI trustworthiness standard,” she says. “They speak of robustness, resilience and trustworthiness, and that's what you hear a lot throughout the industry.
“But now we need to go to the next level, after we established that trustworthiness standard, and ask ourselves, ‘How long will our model last in the field?’ If we can't predict that, then we are about where the hardware sector was in the 1970s.”
To provide McAfee with an accurate way to predict how long its AI models will last in the field, Dr Fralick is developing a formula to analyze average model decay times across different industries and companies. She calls this new standard ‘Mean Time to Decay’, or MTTD.
“Right now, I’m in the middle of gathering some of our KPIs and popping them into the mathematical model of ‘Mean Time to Decay’,” Dr Fralick says. “I have shown many presentations over the last year about how monitors will drive that calculation and sharing some proposed mathematical calculations.”
Creating this calculation for AI reliability at McAfee will be a key focus for Dr Fralick in 2022. If she and others can establish industry standards for predicting AI model lifespans, this will mark a clear step forward for the maturity of AI use in industry today.
Celeste Fralick PhD will speak at Corinium’s 2021 CDAO Fall summit. Click here for more information or click here to reserve your place.