Ensuring Cloud Migration Leads to AI and Analytics Success
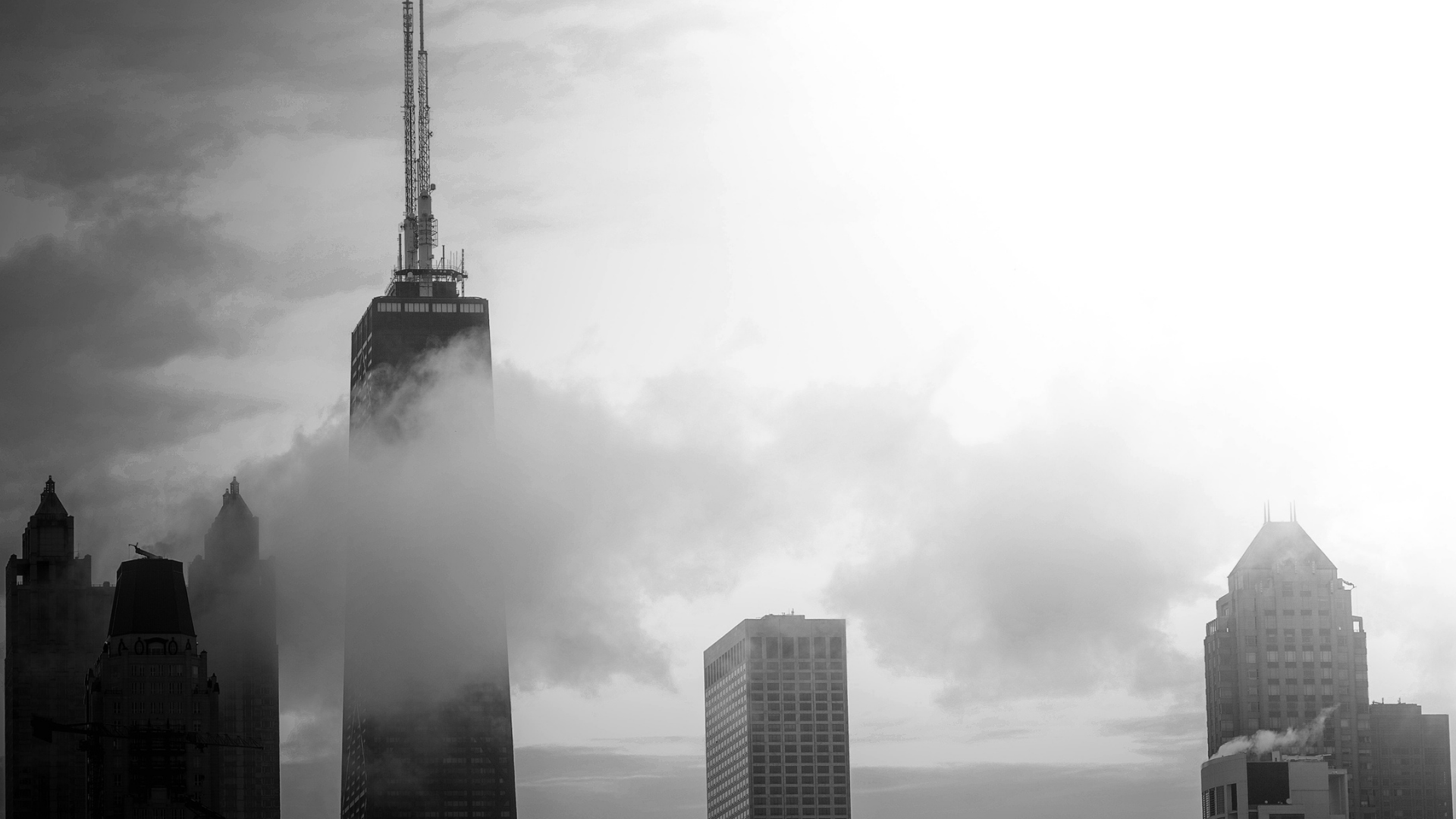
AI and analytics teams benefit most from the cloud. But data leaders must overcome three core challenges to deliver cloud migrations that set their enterprises up for AI success
Migrating data to the cloud supports AI and analytics innovation in two ways. It breaks down data silos to give data scientists and algorithms easier access to data. Plus, it gives AI teams access to environments that facilitate quicker experimentation with new technologies.
Our research shows that accelerating AI adoption in this way is one of the primary reasons that enterprises embrace the cloud. Of the 100 data and analytics leaders we surveyed, 41% cite ‘enabling AI and advanced analytics’ as a key benefit of the exercise.
“[In the cloud,] the team that would like to try something out can do it essentially in a few minutes, as opposed to going through the whole procurement process”
Dr Vladimir Bacvanski, Principal Architect, Strategic Architecture, PayPal
“Cloud providers give enterprises access to modern data management tools that accommodate unstructured and open source technologies,” explains Ashish Verma, Deloitte Consulting’s Managing Director and Data Modernization and Analytics Leader. “Without these capabilities, it’s more difficult for enterprises to operationalize and scale AI tools effectively.”
PayPal Principal Architect, Strategic Architecture Dr Vladimir Bacvanski agrees: “If you are limited to what you can do in your data center, you would need to have a process in which you identify the AI vendor and then you make sure that this vendor's solution will work in your ecosystem.”
“There will be some time where you need to install and put everything in place, and all of that can take significant amounts of time,” he adds. “During that time, your team would like to experiment and try out the new technology. They are essentially paralyzed.”
Accelerating Analytics Innovation in the Cloud
One of the most basic ways enterprises can use cloud migration as a springboard for advanced analytics and AI capabilities is by creating a single place where they can store data from a wide variety of sources.
“This is the low hanging fruit,” says Dr Bacvanski. “This could even be built in-house. So, you have the ability to play with large volumes of data. You keep buckets of files and you essentially have a place where you can put a variety of data sources that can be then used by the AI systems.”
AI leaders have several options when deciding how to do this. For example, our research suggests that 24% or enterprises currently store data or run apps in the public cloud, 43% do so in a private cloud and 47% have adopted a multi-cloud strategy.
“Without [cloud] capabilities, it’s more difficult for enterprises to operationalize and scale AI tools effectively”
Ashish Verma,Managing Director and Data Modernization and Analytics Leader, Deloitte
A public cloud is hosted by a third-party provider that maintains compute resources their customers then share access to via the internet. This allows enterprises to generate cost savings by only paying for what they use.
Private clouds may be built in-house or maintained by a third-party. They provide enterprises with exclusive access to cloud resources and enable them to realize some of the benefits of the cloud, while remaining ‘in control’ of their data and IT environments.
Hybrid clouds connect a private cloud to public cloud infrastructure so workloads can be orchestrated across both environments, while enterprises with a multi-cloud strategy will use a combination of different clouds to achieve their goals.
“A question I often get asked is, ‘Do organizations often have one cloud or multiple clouds?’” Verma says. “In reality, enterprises have multiple clouds. A key consideration in developing and executing a modern cloud strategy is to make sure that interoperability and interaction between one cloud and another is paramount.”
Many cloud providers also enable faster innovation by allowing data science teams to experiment with new technologies in cloud-based development environments. This is particularly useful for newcomers to the field who may struggle to set or operate these systems smoothly alone.
But of course, it’s still usually necessary to develop these capabilities in-house to deploy more complex AI models at scale.
Three Cloud Migration Barriers to Overcome
Despite the various options available and the surge in demand for cloud services we saw in 2020, few businesses have fully embraced the technology. In fact, just 4% of enterprises currently have more than 75% of their data stored in the cloud.
Our research shows that ‘securing executive buy-in’, ‘understanding what data the enterprise has’ and ‘ensuring data privacy and security’ emerged as the top three barriers to cloud migration today.
Securing executive buy-in is a significant obstacle. Just 1% of our survey respondents have ‘completely dealt with’ this challenge, while 78% are finding it at least ‘quite challenging’ to get executives on-side.
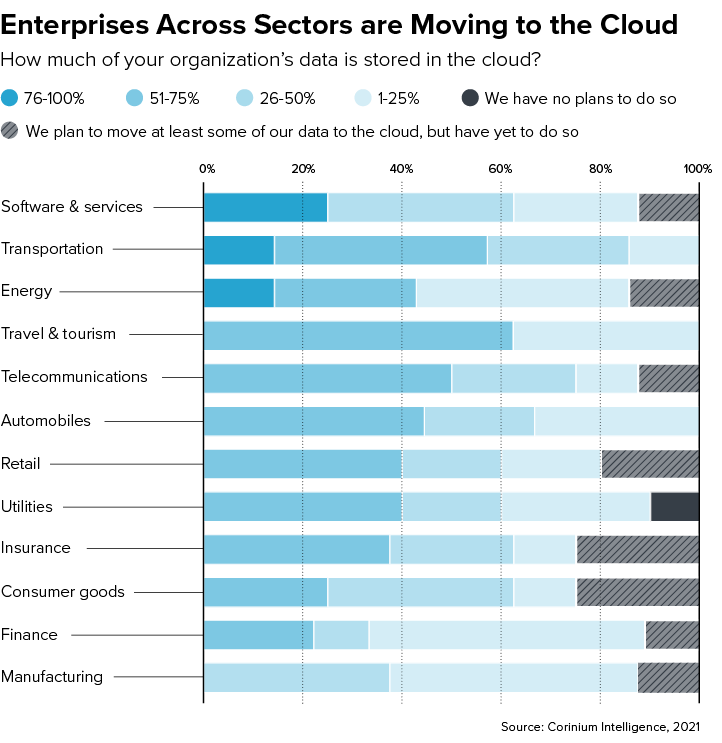
“The way to get buy-in from different members or an enterprise is, understand what their pain points are for their projects,” recommends Dr Besa Bauta, CDO at MercyFirst. “The key is understanding the pain points of the different divisions and partnering with them.”
Concerns around how secure it is to store information in the cloud may be another factor that’s causing executives to hesitate on cloud migration. After all, 56% of our survey respondents state that ‘ensuring data privacy and security’ in the cloud is at least ‘quite challenging’.
“Executives can be hesitant to put sensitive data in the cloud for myriad reasons, including potential security concerns,” Verma says. “Hacks can erode public trust in an organization and cause serious reputational damage.”
To move data safely and securely into the cloud, data leaders must do their due diligence to ensure that a given cloud provider can be trusted to keep their data secure. Showing other executives there will be rigorous security measures in place will go a long way toward getting them ‘on side’.
Analytics Success Depends on Good Data Management
Besides the challenges of securing executive buy-in and ensuring data in the cloud is secure, 63% of our survey respondents say ‘understanding what data the enterprise has and where it’s kept’ is at least quite challenging within their organizations.
This highlights how tough data integration can be, especially for enterprises with many business units or companies under their umbrellas.
Historically, data leaders have attempted to address this challenge by creating centralized data lakes to acts as ‘single sources of truth’ for their organizations. But in recent years, we have seen a decline in this practice.
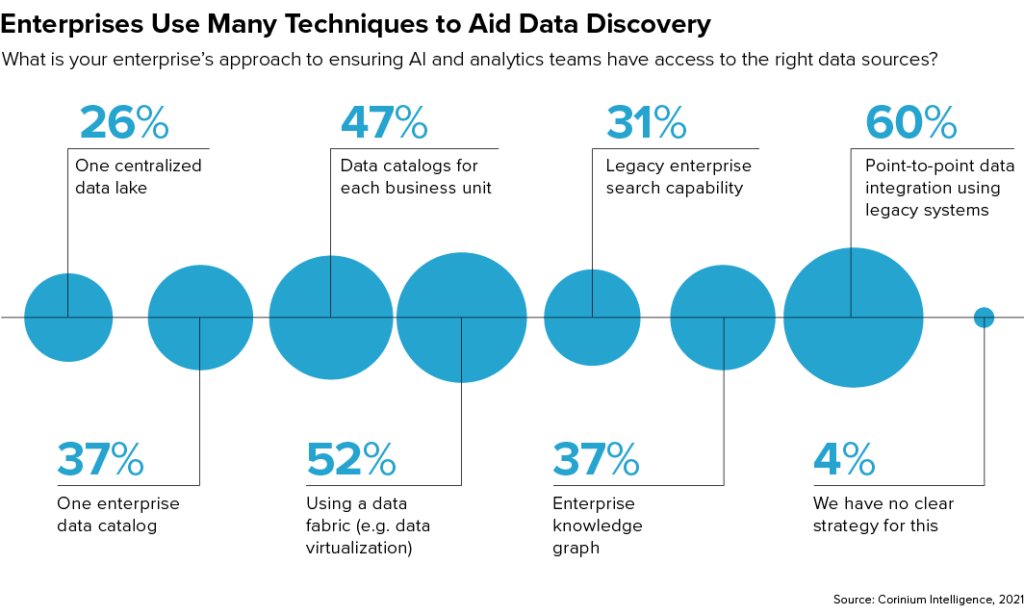
Our research shows that just 26% of enterprises are currently pooling data in a centralized lake. Meanwhile, 37% have an enterprise data catalog, 47% have data catalogs for each of their business units and 52% are using data virtualization to integrate data from multiple sources.
Enterprises that opt for a ‘lift and shift’ approach to cloud migration can sidestep this data discovery challenge by focusing just on replicating certain on-premises data stores in the cloud.
Yet, those that wish to use their cloud migrations as springboards for future AI initiatives must take steps to engineer a data infrastructure that arms their data scientists with the data and tools they need to succeed.
This is an excerpt from our Driving Business Value with AI in the Data Cloud research report. For even more insights about how enterprises are using cloud migration to unlock the potential of AI-led innovation, click here now.