Dr. Sizwe Gwala: Data Fabric and Mesh, Pioneers for AI-driven Data Management
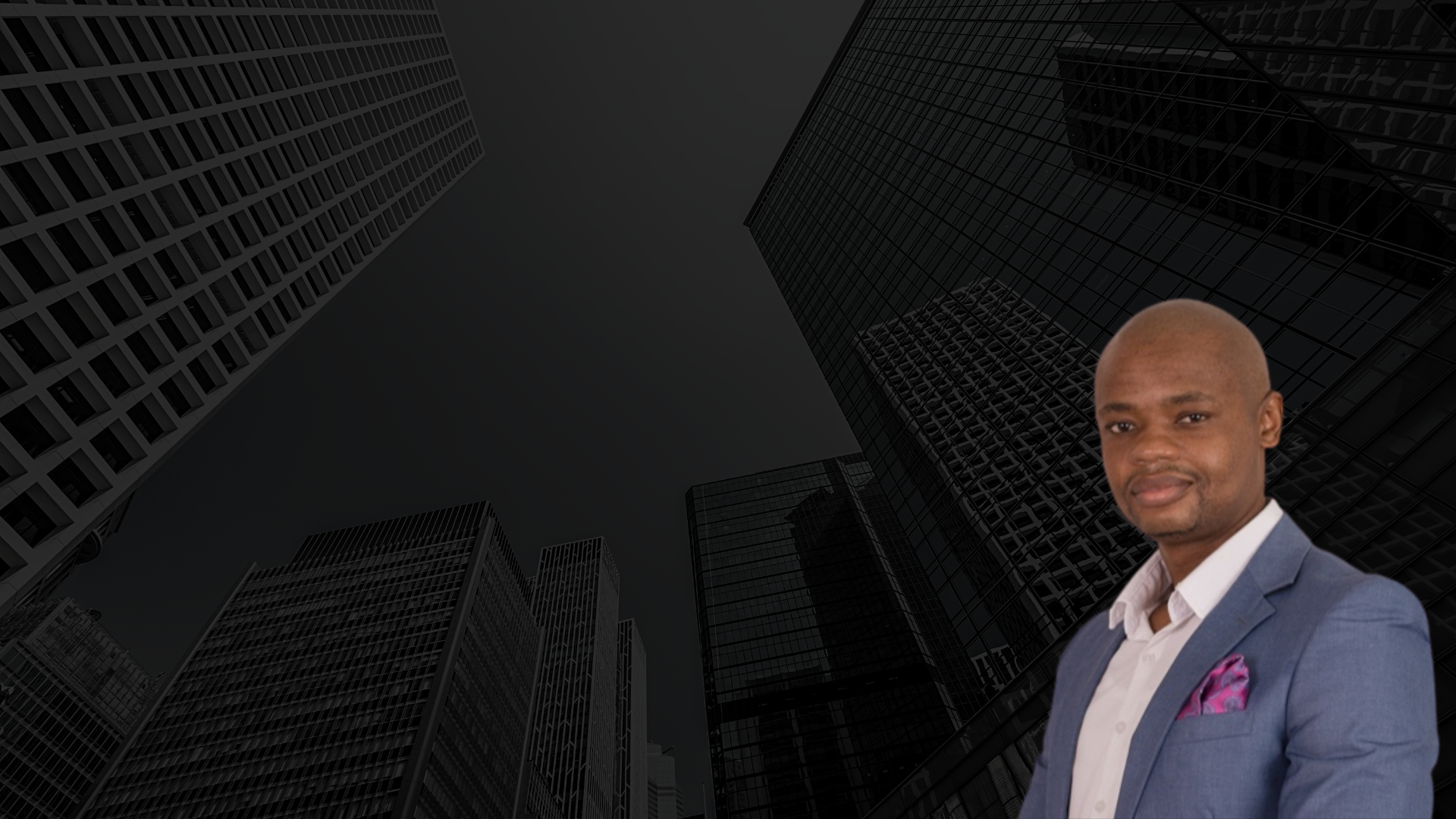
This guest blog from Absa Group Compliance's Head of Data Governance Dr. Sizwe Gwala discusses how the next generation of data management practices are enabling improved data capabilities, operational efficiency and access
Artificial intelligence (AI) and machine learning (ML) are modern-day technologies fueling digital transformation in in data-driven businesses. Their deployment is dependent on business’ flexibility and ability to continuously adapt to emerging data management (DM) practices, in handling and managing their ‘crown jewels’.
The past few years have seen the DM discipline transitioning from traditional databases, to warehouses, and then data lakes, all guided by business maturity and the complexity of a problem solutioned for.
Data fabric and data mesh are the logical next steps in DM practices, with fabrics being technology-centric, and meshes largely focusing on institutional changes. Although distinct in most parts, these practices are interrelated and often complementary when adequately implemented and driven by skilled practitioners.
Centralization Through a Data Fabric
A data fabric is a metadata-driven approach connecting a variety of sources and applications in a united and self-service manner. It is an all-encompassing, integrated architectural layer which serves to accelerate data inference through automated processes, while also providing real-time insights. Data fabric architectures operates around the notion of loosely coupling data in platforms with required applications.
An example is a multi-cloud environment where one cloud provider manages data ingestion, and another oversees data transformation and consumption. There might further be a third service provider responsible for analytical services. With a fabric overlayed virtually over various data repositories, AI-driven technologies can be seamlessly deployed thereby enabling businesses to leverage human and machine capabilities for centralized data access, and to discover unique, business-relevant relationships across integrated data points and downstream consumers.
Decentralization Through a Data Mesh
Data meshes are, in turn, largely decentralized and encourage dispersed business teams to manage data as they deem fit, albeit with some common governance controls. The rationale behind a mesh is to solution for synchronization issues between databases, data lakes and warehouses by filtering their views in line with commonly shared data elements, across users instead of hardcoding them for transformation.
A data mesh approaches DM from a people-and process-centric view while also handling data as a product, each with an owner who could be part of a cross-functional team of data engineers. In a typical mesh, data is maintained on the same format as the source, and then adopted by domain-specific teams to localize it into a data product as they deem fit.
Enabling for AI-driven Data Management
AI has the potential to transform nearly all key business practices, including how people work, communicate, travel, and govern data. However, taking full advantage of this technology requires enterprises to adopt modern-data architectural approaches such as a data fabric and a mesh. With AI-driven DM integrated within a modern architecture, businesses are better placed to derive the following benefits:
- Improved data access and repository
- Improved operational efficiency
- Improved data query performance and accuracy
- Automated data profiling, analysis, and modeling
- Accelerated data scientist productivity
Key Takeaway
AI and ML are modern day technologies fueling digital transformation in in data-driven businesses. Their deployment is dependent on business’ flexibility and ability to continuously adapt to emerging DM practices such as date fabrics and data meshes.
Fabric is an all-encompassing, integrated architectural layer which serves to accelerate data inference through automated processes, while equally providing real-time insights. And a mesh is decentralized and encourages dispersed business teams to manage data as they deem fit, albeit with some common governance provisions.
With AI-driven data management integrated within a modern architecture, businesses will be better placed to derive various benefits including inter alia; improved data access, operational efficiency and automated data profiling, analysis, and modeling.