Prescriptive Analytics and the Rise of Advanced AI
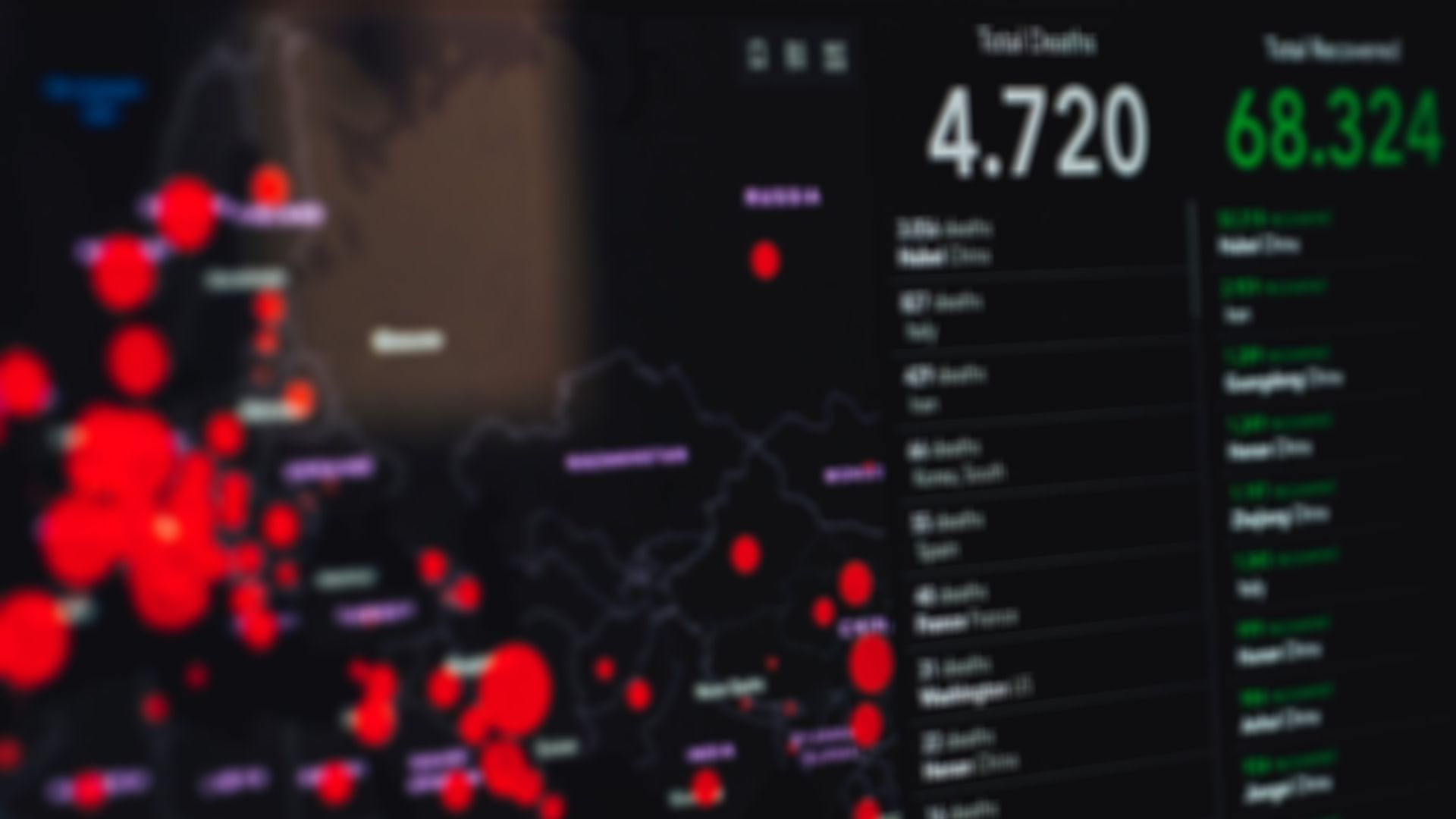
Reinforcement learning, multi-agent systems and other advanced AI models will help organizations transition from predictive to prescriptive analytics in 2021
Global AI maturity has skyrocketed over the past 24 months. While Gartner reported that just 4% of organizations had deployed AI capabilities going into 2018, IDC research suggests that 75% will have by 2022.
Our research shows that this transformation is well underway. When we interviewed 104 C-level data and analytics leaders in April 2020, two thirds said they have deployed AI within their organizations. In fact, 25% reported having a fully unified, enterprise standard approach to scaling AI in place.
Today, simple AI capabilities are becoming commonplace in companies across the US. Using AI to predict future events is no longer ‘cutting-edge’. Instead, the world’s most forward-thinking AI leaders are developing advanced AI tools that prescribe specific actions to improve organizational outcomes.
“That’s where the buzz is with AI,” says Lockheed Martin Space CDAO Tobin Thomas. “The potential there is huge. So, a lot of the investment that we’re doing is within that base – reinforcement learning and unsupervised learning.”
Johns Hopkins Healthcare Senior Director of Healthcare Economics and Data Science Romy Hussain is also leaning into these ‘next generation’ technologies. She predicts her organization will have deployed prescriptive AI-powered tools within 12 months.
“I’m really excited by reinforcement learning,” she says. “The possibilities there are really endless – not just to predict something like an outcome but also to recommend what we should do about it.”
Advanced AI techniques like these look set to usher in a new era of digital transformation and hyper personalization in the coming months and years.
Creating Better Processes with Reinforcement Learning
Reinforcement learning is a branch of machine learning that uses simulated ‘rewards’ to teach AI systems to achieve desired outcomes in uncertain circumstances through trial and error. The technique received widespread coverage in 2017, when Deep Mind Technologies’ AlphaGo software beat Ka Jie, the world’s top ranked Go player at the time, in a three-game match.
“If we add [items] to [a shopping] cart, the online retailers present us with options automatically,” explains Foz Saeed, Director of Marketing Analytics and Insights at biotech firm Thermo Fisher Scientific. “The discovery of which options you present is algorithmic and belongs to the predictive side. The automation of it belongs to the prescriptive side.”
“There are a ton of applications, certainly in the healthcare field, but also in the wider world,” adds Hussain. “For example, a lot of ride sharing app reinforcement is used to pick out optimal routes from A to B.”
At Johns Hopkins Medicine, Hussain is using reinforcement learning to build prescriptive capabilities into her patient readmissions models to provide care recommendations for different patient segments.
“In the data science world, we call it ‘policy creation’,” she says. “It’s, ‘What are the policies by which, if we followed them, the adverse or predicted outcome could be avoided?’”
The beauty of reinforcement learning is that it can find patterns in data that humans miss. But because it depends on trial and error, it’s only an efficient solution to certain business challenges.
Reinforcement learning should be considered when it’s possible to define a concrete ‘reward’ function to reinforce the algorithm’s good choices. It’s best suited to situations where there’s plenty of time available to run the machine until it arrives at a good solution.
Using Multi-Agent Systems for Policy Planning
Multi-agent systems are another AI tool that looks set to shape policy development at Johns Hopkins in the future.
They allow data scientists to design virtual worlds, populate them with objects and explore how applying different properties to those objects affects the behavior of the system.
“The very famous example is the COVID-19 model,” Hussain explains. “They have a multi-agent system that created different clinical typologies around facial coverings, where people are going, what they’re doing, etc.”
“The interesting thing is, you can introduce different stimuli and see how that affects the outcome,” she continues. “It’s really great for exploring the ramifications of different policies.”
While this is not technically a prescriptive technology, multi-agent systems make it possible to model scenarios that are more complex than simpler machine learning techniques. As such, its applications stretch far beyond stopping the spread of infectious diseases.
For example, Hussain is helping Johns Hopkins’ health insurance division use multi-agent systems to model how healthcare providers are likely to react to changes in reimbursement policies for certain types of procedure.
While it’s still a very nascent technology, companies including IBM, car manufacturer Daimler Chrysler and the US Ministry of Defense have already successfully implemented multi-agent systems.
When to Explore Advanced AI Technologies
There is huge pressure on data leaders to invest in AI technologies. But doing so without a clear objective or the right infrastructure in place is risky.
At the same time, these technologies are very resource intensive. From a business perspective, simpler solutions will often be preferable to more complex AI technologies when they are available.
“Maslow’s Hammer says that, if all you have is a hammer, every problem looks like a nail”
Luke Dicken, Director, Applied AI, Zynga
“The problem with a whole bunch of these technologies is, they can be any kind of tool that you want them to be,” notes Luke Dicken, Director, Applied AI at gaming company Zynga. “But [they’re] never going to be exactly what you need.”
Saeed adds: “If you don’t have defined processes, if you don’t have a defined business model, if it’s not digitized or you need manual intervention, it will be very difficult to implement AI.”
Ultimately, many organizations are still testing the water when it comes to AI. But the top 25% which have the AI maturity to take advantage of these cutting-edge technologies are in a very enviable position.
“AI-driven processes are coming,” Dicken concludes. “It has to be coming. It’s what’s next. You’re in a position now, across various industries, where you can actually catch this wave early.”
This is an extract from our 2021 Transformational Data Strategy US report. For more exclusive insights about the technologies shaping the future of data and analytics in America, click here now.