Building the Foundation: Data Ecosystems Essential for Advanced Analytics and AI Success
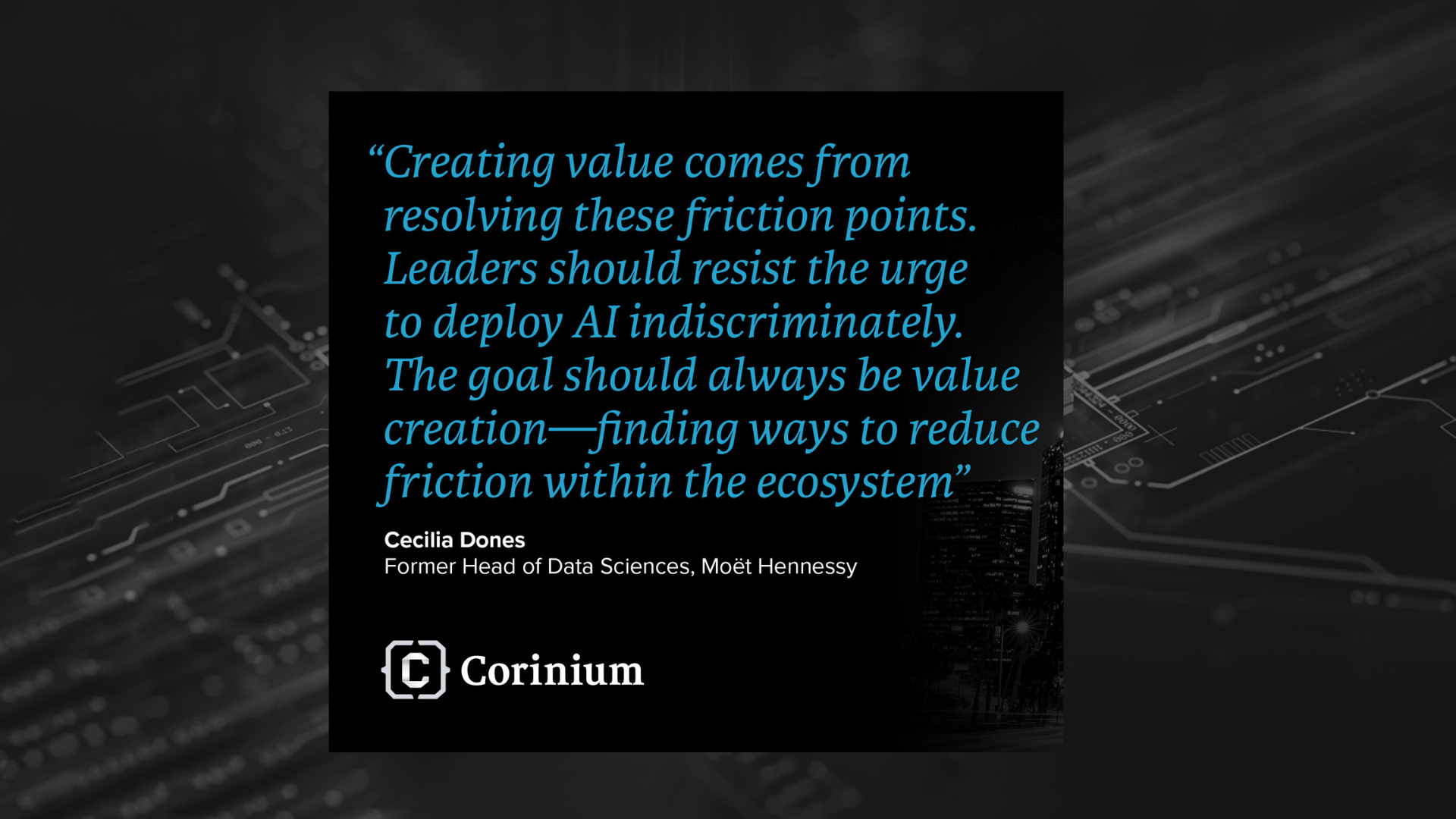
Optimizing data ecosystems is critical for leveraging AI
and advanced analytics in business decision-making amid challenges of data quality, availability, and integration
Data, analytics, and AI are essential enablers in today’s business landscape. They are key to promoting innovation, increasing efficiency, and managing risks.
To wield these tools effectively, modern businesses rely on interconnected networks of data, tools, and processes that enable the management and analysis of vast amounts of data.
These data ecosystems include various data sources, both internal and external, as well as data storage, data processing tools, and analytics applications. A well-organized data ecosystem is crucial for large organizations as it ensures data accuracy, consistency, and availability, which are key for informed decision-making and gaining a competitive edge.
Moreover, it also addresses challenges related to data security, compliance, and integration, thus ensuring smooth functioning and maximizing the value derived from the data.
“Enterprise data ecosystems involve making data available for business use, not only for insights and analytics but also for dashboarding, reporting, and critical decision-making at the executive level,” says Anish Agarwal, Global Head of Analytics at Dr. Reddy’s Laboratories, an Indian multinational pharmaceutical company.
Yet, the implementation of innovative data, analytics, and AI initiatives is often more difficult than anticipated. Over time, many businesses accumulate disparate systems, fragmented data, and outdated technology, all of which can hinder or even halt efforts to innovate and modernize.
Recently, we conducted a survey of 100 C-suite leaders and senior decision-makers in Data, AI, and Innovation in partnership with AI, analytics, data and research firm, WNS Triange. This research identified a host of challenges for enterprises on the road to modernizing their data ecosystems.
The Importance of Modernized Data Management and Its Key Challenges
The single biggest enterprise data challenge revealed in our research is ‘data quality,’ named by 57% of respondents.
“From a data perspective, the primary challenge is data quality, which includes several dimensions: completeness, conformity, accuracy, integrity, and currency,” Agarwal explains. “It is vital to establish a method to measure, monitor, and improve data quality.”
Additionally, nearly half of respondents cited data availability, accessibility, useability, and data governance (48% and 47%, respectively) as significant challenges when creating better data ecosystems.
“Many organizations view data governance as a significant obstacle, regardless of their level of maturity,” notes Cecilia Dones, Former Head of Data Sciences at Moët Hennessy. “This is because governance often reveals accumulated technical debt from past decisions, such as failing to normalize data or maintaining records exclusively in Excel. These short-term choices can hinder long-term capabilities.”
“One of the key challenges in data management today is data availability. It’s crucial to have the right data, in the right format, available when it’s needed,” adds Ravindra Salavi, Senior Vice President – AI, Analytics Data and Research at WNS Triange.
He continues: “Many analytical programs haven’t delivered the ROI businesses expected because data scientists often haven’t received the correct data. Additionally, the data might not be updated frequently enough, or it might not meet the quality and conformity standards the business needs. The depth and history of data are also essential for analytical models.”
Salavi’s insight underscores the ripple effect of this challenge, affecting not only the ROI of analytical programs but also the overall decision-making process of the business.
What’s more, aspects of modernized data management, like having an efficient data catalog, are at the heart of solving data accessibility issues and are essential to implementing advanced analytics and AI initiatives.
“When building a model, it’s crucial to determine if a variable is well-populated, as this impacts model accuracy,” says Ines Ashton, Director of Advance Analytics at Mars Petcare. “With an efficient data catalog, one can quickly identify and prioritize the most reliable variables for modeling. This speeds up the decision-making process and reduces the chance of investing in ineffective models.”
Data Integration Tools and their Role in Integrating Siloed and Fragmented Data
In a world increasingly powered by data, enterprises face the overwhelming task of managing scattered and compartmentalized data due to a variety of factors, including the use of diverse systems and technologies, and the impact of legacy technology on data and analytics initiatives.
Over half of companies (57%) use data integration tools to dismantle silos, making it the most adopted strategy by companies to address fragmented data. Meanwhile, 54% of companies are executing phased integration of systems to minimize disruption, which is the leading strategy for bridging the gap between legacy and cloud systems.
However, data migration to the cloud remains a significant obstacle for two-thirds of respondents, 67% of whom find it difficult to navigate the complexities and challenges associated with this task.
“Data integration platforms offer significant benefits,” says Agarwal. “First, they provide a comprehensive view of underlying metadata, allowing insights into additional attributes essential for data cataloging. Second, these platforms can measure and, to a large extent, remediate data quality. Plus, they integrate with third-party databases, ensuring data consistency.”
The need for data integration tools is accentuated by the complexity of aggregating data from multiple sources while ensuring data integrity. Indeed, our research indicates that more work needs to be done to prepare data for use in AI initiatives.
“Aggregating data from multiple sources is a complex problem,” Agarwal emphasizes. “Data integrity is crucial. Usability is not just about availability; it’s also about creating a comprehensive data catalog that allows data scientists, analysts, and businesses to understand and utilize the data effectively.”
The impact of legacy technology is significant. Cecilia Dones, Former Head of Data Sciences at Moët Hennessy, notes: “For legacy organizations with established data architectures, transitioning everything to the cloud can be risky due to fragile components in their system. For most large organizations with legacy systems, a hybrid solution might be necessary.”
The challenge of modernization is further complicated by the makeshift solutions many organizations use to supplement their older systems.
“Many organizations are trying to compete with the surge of tech-enabled companies by applying makeshift solutions,” Siddharth Bhatia, EMEA Growth & Practice Leader - AI, Analytics, Data and Research at WNS Triange says. "They’re trying to supplement their older systems with solutions like intelligent process automation, robotics process, or data workflows. However, no matter how much you modify an old system, it won’t become a cutting-edge solution."
“The biggest challenge for many CIOs, CDOs, and CTOs is transitioning to a modernization agenda within their organization. The primary concern is falling behind technologically due to the weight of their legacy systems and the vast amount of potentially irrelevant data they’ve amassed,” he concludes.
The transformational possibilities of well-managed data, free from fragmentation and silos, are immense and crucial for the future success of any enterprise.
Data leaders who successfully navigate these complexities can unlock a wealth of insights, enabling smarter decision-making, enhanced customer experiences, and streamlined operations.
Moreover, by dismantling silos and integrating data across the enterprise, organizations can cultivate a culture of collaboration and innovation, ultimately driving competitive advantage in an increasingly data-driven world.
As technology continues to evolve, so too will the tools and strategies available to data leaders, opening up new opportunities for leveraging data as a strategic asset and catalyzing positive change across the organization.
This article is an extract from our recent industry report: The Future of Enterprise Data and AI. You can get access to the full research report here