Business of Data Meets: Citi Chief Data Scientist Dan Costanza
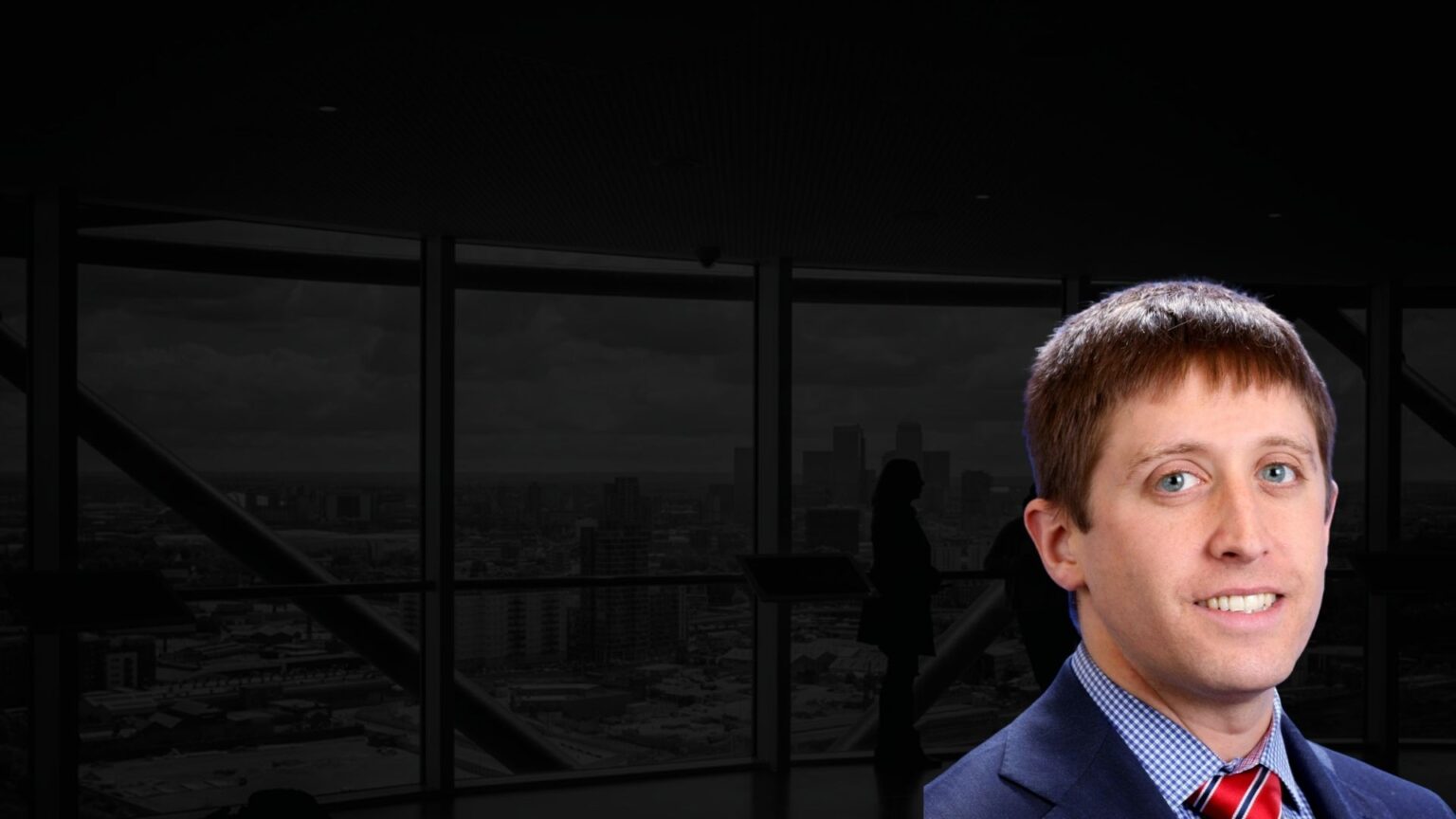
Dan Costanza, Chief Data Scientist: Banking, Capital Markets and Advisory at Citi, outlines how he’s working to democratize the bank’s data, what’s next for his data strategy and what makes his job is different from other C-Level data roles
What were your greatest professional achievements in 2019?
In 2019, our real focus was on increasing engagement with non-data-driven parts of the organization to try to get them to think in a more data-driven fashion.
So, less about trying to design the world’s most complicated and perfect predictive analytics and more about, ‘How do we change the culture of the way people interact with or think with data?’ and trying to turn everyone in the organization slowly into something that could loosely be described as a data scientist.
I think we made some really good headway with that in some specific business units and are continuing, this year, to really broaden that scope.
How will you build on those achievements in 2020?
The focus for me now is identifying the next set of adopters.
We’ve had the early adopters, who are the people who you can convince to buy in based on the concept. Those are the lowest hanging fruit, who are really open to the things that we’re doing.
Now, we have actual specific results that we can take around to show to people who are interested and engaged but a little bit more skeptical and that’s a much broader universe of people. So, over the next year, the hope is that those people are where we will really build engagement, so we can convert them.
That will still be a reasonably small universe, but larger than what we were working on last year.
As you convert more people, that hopefully gets you more advocates who are walking around telling their colleagues about the value that’s being created and putting your work in front of clients and seeing the client impact of that.
What are the key challenges you anticipate running into as Citi embarks on this next phase of its data journey?
The space of problems that exist to be solved in what we would call our ‘sandbox’ is very big. So, I think the challenges are primarily around how we identify the right problems to focus on.
How do we deliver immediate impact while still building things to scale? How do we identify projects that are worth doing and which we can execute on in a way that is efficient enough to maintain people’s focus and excitement?
If we disappear for a couple of months and come back, people will have forgotten about us. But we must also work without writing a bunch of ‘throw away’ code that we’re just hacking together as quickly as we can.
We need to be building good components and good pieces that will allow us to take the next step and continue to drive forward without bogging ourselves down with legacy code.
That is less about the technical implementation and more about project selection. So, identifying projects that we know are going to lend themselves to building useful modules and being able to quickly deliver and build towards bigger strategic goals or projects.
Beyond selecting projects that feed into larger strategic goals, how else do you ensure your team can deliver data products efficiently?
A big part of it is managing feature bloat and making sure that we keep our minimum viable products really tightly scoped and focused on a specific objective, instead of trying to solve all of the problems up front.
We also make sure that all the projects we work on are easily modularized so you can break them down into pieces.
Then, the other big challenge is making sure that we do go deep enough into each area that we have real impact. If we work with a ton of people, at the end of the day, who are the people who are going to stand there and say, ‘These guys have added value’?
We need to make sure that, while we cast a wide net to identify the right people and the right places to add value, we also continue to delve deep enough and integrate closely enough with the work they do. We need to become core partners in those business areas, instead of just being people who did an interesting thing or helped out here or there.
How do you think the role of sort of a company’s Chief Data Scientist will continue to evolve in the coming months?
That’s a good question. And I think it’s very idiosyncratic to the company and the type of data science they’re doing.
The work that someone’s going to be doing at AIG, for example, can be very different from the things that might be done in a retail company versus things that might be being done in a bank, versus other places. These different focuses lend themselves to different approaches.
So, in some organizations, they have a core data operation that is steadily gaining power and influence within the organization.
But for me, my goal is to diminish the power of the data organization by making it more of a part of the rest of the business. I don’t want to build a giant system of data scientists sitting within this broader bank. I want every team within the bank to use data in the way they think.
So, for me the growth of the role is all about how we integrate, not about how we build a build a platform or build a large team.
I don’t want to have 200 data scientists working for me. I’d rather have a bunch of bankers around the firm, where we have 10,000 people who all can do a little bit of data science but are addressing the right problems. Then we have a small team of specialists who come in and help out when you need a little bit more algorithmic design or structure.