Canadian Data Leaders Eye New Opportunities with Generative AI
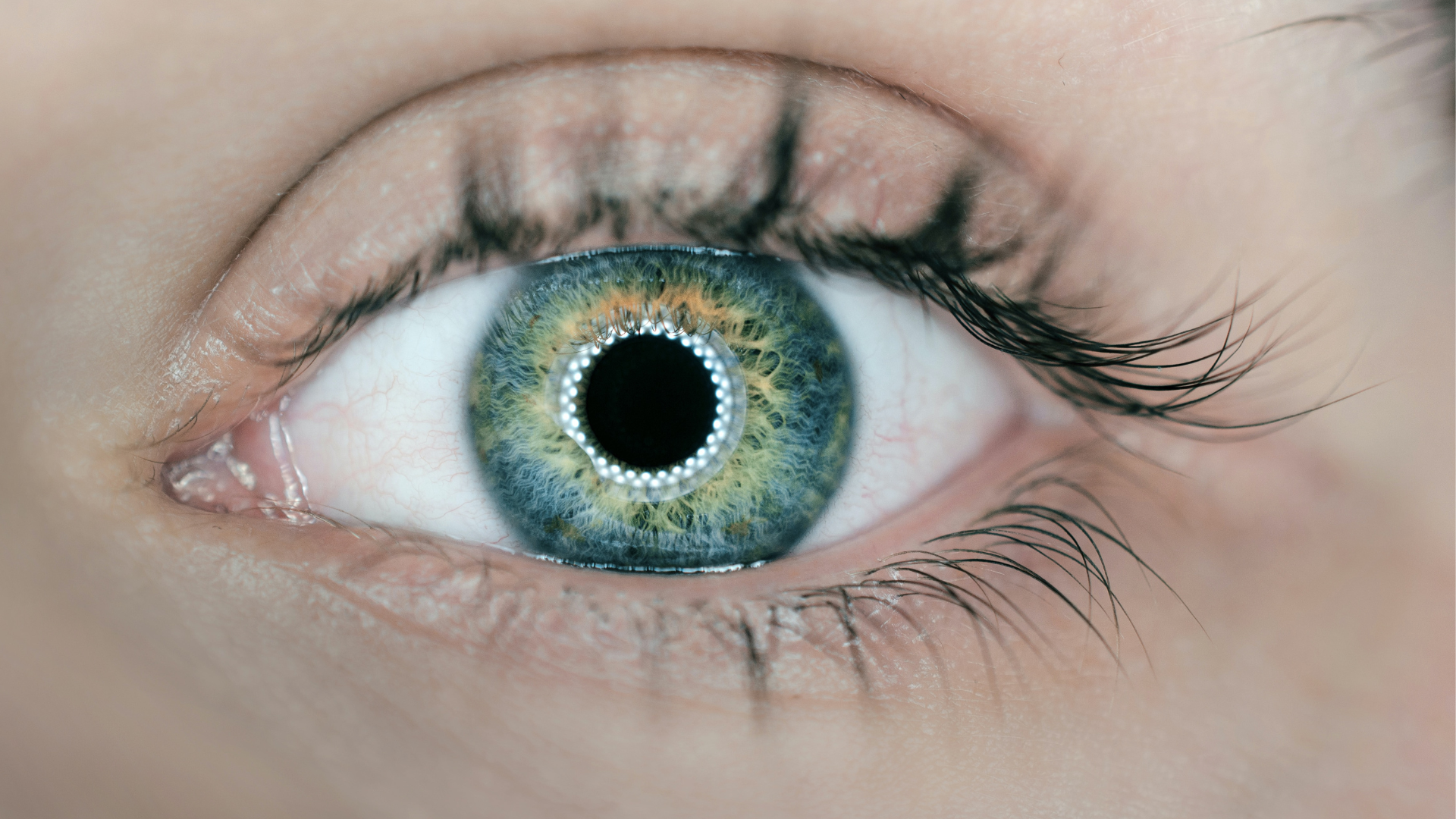
Dean McKeown, Interim Director and Master of Management in Artificial Intelligence at the Smith School of Business at Queen’s University shares his analysis of the key issues around implementing new technology in the age of generative AI
Where do the opportunities lie for Canadian enterprises when it comes to implementing generative AI?
"Canada does very well in terms of knowledge level, thanks to our high standards of education. We can hold our own against larger countries like the UK and America. However, Canadian institutions are generally very conservative, especially when it comes to spending on research and development and are quite risk averse.
Many organizations have small research departments exploring AI, but operationalizing it is a different story. In the financial sector, for example, large language models, particularly in chatbots, have had a significant impact. Canada's structured and centralized banking system, with its major banks, has embraced using chatbots to support customers, recognizing that customers want information quickly rather than necessarily wanting to talk to someone.
This trend is also extending into the retail sector. In industries like oil and gas and natural resources, there has been notable movement, especially in the last five years. As oil prices fell, many companies realized the need for digital integration and transformation. I've had frequent calls with oil companies about digital transformation, especially when oil prices were low. However, as prices have rebounded and profits returned, the urgency for digital transformation seems to have lessened somewhat.
Despite this, analytics plays a crucial role in areas like pricing, preventive maintenance, and exploration in natural resources. Different organizations vary in their maturity levels in using analytics, but the companies poised for success in the long term are those already looking at how to leverage technology to improve their operations."
Regarding the integration of technology and AI, what are the key challenges on the people side?
"It's multifaceted. You've got senior people resistant to change, sticking to the 'we've always done it this way' mindset. That's like Kodak, who had good research into digital and cloud storage for photos but failed to adapt and ultimately disappeared. Companies must embrace AI and figure out how to apply it to their specific situations.
The key now is working with people, educating them, and democratizing data. This means not relying on an IT group for data access anymore. Tools like Microsoft Fabric, Snowflake, and Databricks allow organizations to share data among businesspeople in a controlled, governed way.
Once data sharing is established, the next challenge is educating businesspeople in analytics, and teaching them to distinguish between relationships and causality. Decisions must be evidence-based, and supported by data analytics, while still valuing gut instinct and intuition.
The process involves using the scientific method: identify a problem, formulate a hypothesis, test it, and learn from the results. We need to maintain this framework, combining intuition, data, analytics, and technology. It's about ensuring the right data is in the hands of the right people for analysis.
In summary, we need to educate senior leaders about the importance and potential of this technology, what competitors are doing, and the need to adapt. Once the C-Suite is convinced, develop a training program for businesspeople to understand analytics and its capabilities.
Build out governance sections for appropriate data access, considering privacy, legal, and regulatory aspects. Then, push this knowledge out to the customers. Businesspeople, who know the business best, are the ones who can truly leverage technology effectively."
Moving to ethics and regulation in AI, what's the situation in Canada, and what do you foresee for AI regulation?
"That's a tough one because we have multinational corporations and organizations. Nobody lives in a vacuum anymore of just a specific country or even a specific province. And so, this is where the challenge is.
In Canada itself, we do have a couple of bills waiting to be passed regarding AI and AI regulation and privacy. We're still waiting to see how that plays out, likely after the holiday break, in January or February. But it's going to be hard. Europe, for instance, has taken a much harder line with things like GDPR, emphasizing individual privacy and the right to be forgotten. That's one end of the spectrum. On the other end, you have the U.S., where a lot of the privacy and AI regulation is happening at the state level.
The big question is whether people will start choosing organizations based on their AI and ethical principles or just focus on the bottom line and products. This is a game that's going to be played out over the next decade. There’s going to be a tightening of regulations, and then there must be the enforcement piece, which is going to be extremely difficult.
Then there's the layer of large language models and generative AI, which changes the game every four months. Once regulation gets passed, there’s going to be some technology around the corner that’s going to necessitate rethinking.
It's a push and pull between great technology and the potential misuse of data. Layering regulations on top of that could get messy very quickly."
What developments do you anticipate in generative AI for Canadian businesses in the near future?
"Sustainability is key. Products like ChatGPT are hugely intensive in processing power and electricity, making them expensive to run. With considerations like carbon pricing and the location of cloud computing servers, these factors will need significant consideration.
What I see now, despite us referring to them as large language models, is a trend towards numerous smaller LLMs. We'll be able to tailor them for specific purposes. For example, in financial institutions, instead of one large language model running the entire bank, there will be smaller models for specific tasks like customer relations chatbots or capital market research. This approach will reduce the processing power required, making it more cost-effective and sustainable.
The future lies in understanding the technology and operationalizing it affordably. Another critical aspect of the next iteration of LLMs is building checks and balances to prevent issues like hallucinations and false reporting. We've seen instances, especially in academia, where models provide fabricated sources. This is concerning, as are the social biases embedded in some models, which can pose significant risks.
I've heard of companies running three or four different LLMs to test the results from the first one. This is to ensure accuracy, as hallucinations can be perpetuated. So, the next steps involve making these models cost-effective and accurate."
Want to learn more?
CDAO Canada Public Sector kicks off on June 18th, 2024 in Ottowa. Join the leading lights in Canadian public sector data and analytics to learn about the latest trends and opportunities in the industry. Register to attend here.