AI, Data-Driven Decisions, and Financial Innovation: Dinto Jose
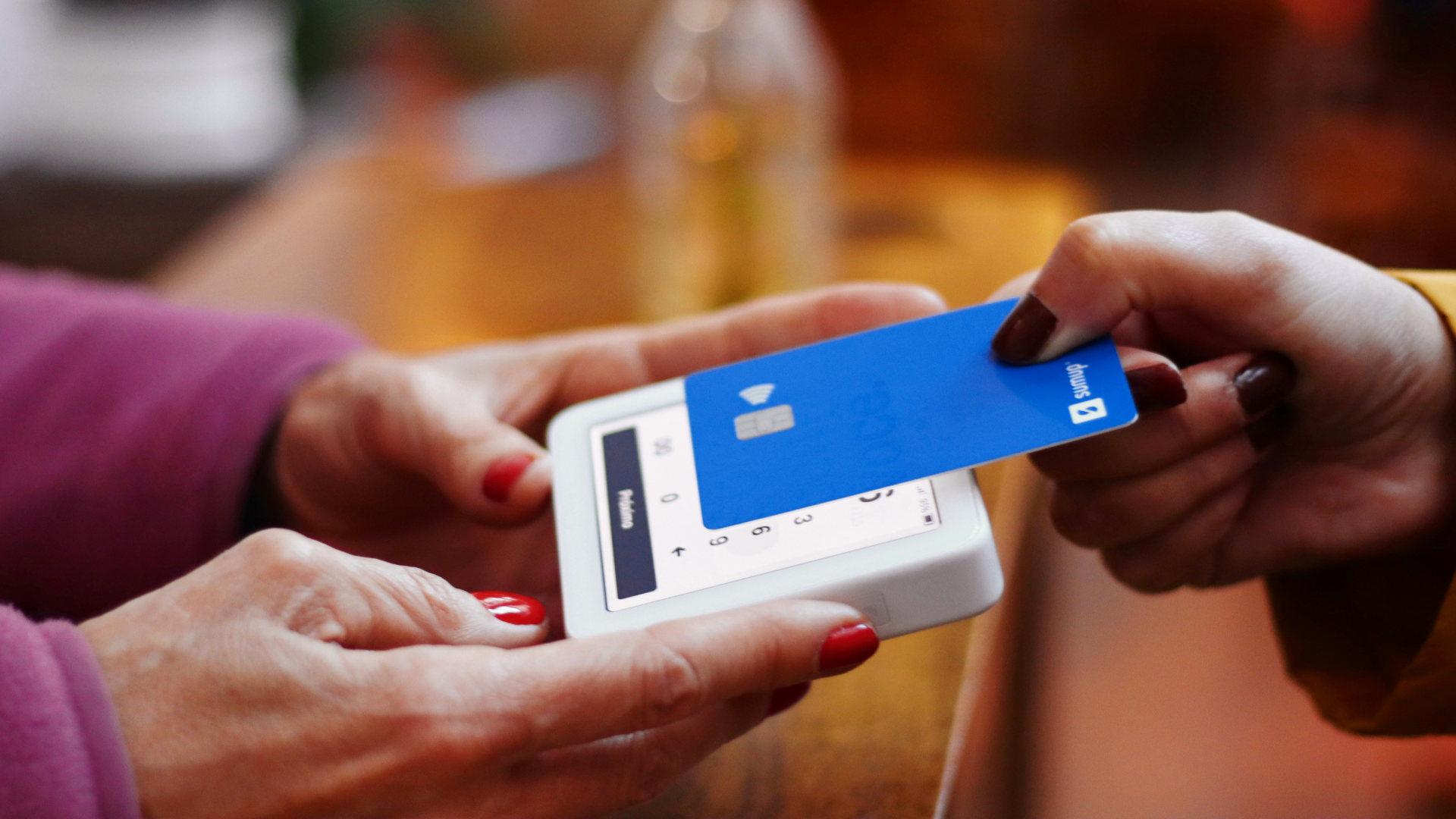
Dinto Jose is Vice President of Merchant Solutions at Deutsche Bank, with a background in engineering and extensive experience in model development. He worked at Accenture and PayPal before joining Deutsche Bank in 2018, where he now leads product management, focusing on revenue growth and innovative product development in financial services
In advance of his speaking engagement CDAO Frankfurt, we asked Dinto Jose, Vice President of Merchant Solutions at Deutsche Bank to share his insights into the latest trends and challenges facing banks in the era of data, analytics, and AI.
With a background in bioinformatics and extensive experience in consultancy and payments, Dinto brings a deep understanding of how data and AI can transform business strategy in the financial sector.
In this exclusive interview, Dinto shares insights into the challenges traditional banks face when integrating AI and machine learning and offers practical advice for financial institutions looking to innovate while navigating regulatory complexities. He also explores the evolving role of AI in areas like risk management and customer experience.
Register now for CDAO Frankfurt to hear directly from Dinto Jose and other leading professionals about the latest trends in data and analytics in DACH.
C: How do you see the role of traditional banks evolving in the era of AI, especially with the rise of fintech and alternative financial services?
Dinto Jose: I see three key areas. First, in customer experience, we’ll see more personalized services and products offered by banks. AI will allow for greater customization to meet individual customer needs.
The second area is cost optimization. We’ll see more transformation projects in banks with a focus on reducing costs, driven largely by AI-powered automation. These projects will target operational efficiency and risk management—two areas where AI can make a significant impact.
Finally, when it comes to fintech, we’ll see more partnerships between traditional banks and fintech companies. The advantage fintechs have is their ability to move quickly—they can go to market fast, with small teams and fewer processes. They can test, iterate, and adapt rapidly. Banks, with their established customer bases, will likely partner with fintechs, where fintechs provide the technology and banks act as the front-end service providers.
C: How do you see the evolution of AI and data-driven decision-making progressing, and where do you think we stand today?
Dinto Jose: Traditionally, decision-making has been based on experience. But with more data becoming accessible, we’re seeing a shift toward data-driven insights guiding strategic decisions.
These days, you don’t have to be a data scientist to derive insights from data. There are tools available that make it easier to access, process, and analyze data. Cloud services have also simplified data sourcing, which means collecting and processing data is far easier and more cost-efficient than before. I believe this trend will continue.
The second area I see is predictive analytics. More organizations are leveraging data to make predictions, and AI and machine learning are playing key roles in enhancing these capabilities.
The third area is risk assessment and management. AI is becoming crucial in evaluating and managing risks. These are the three areas where I believe AI will play a pivotal role in shaping strategy development moving forward.
C: What do you see as some of the major challenges for banks today in integrating AI and machine learning into their existing systems? And what strategies can they use to address these challenges, especially in such a heavily regulated environment?
Dinto Jose: I see two main challenges. First is data quality and integration. Data comes from various sources, and in most banks, it’s spread across multiple systems and geographies. This can create issues when implementing AI, particularly because of inconsistencies in data quality.
One potential solution is centralizing the data into a unified repository, but this isn’t always feasible due to regulatory constraints. The key is finding ways to integrate the data to create a holistic view across the bank, and not just in individual businesses.
The second challenge is regulatory compliance. Banks are highly regulated, and there are concerns around data privacy, biases in AI models, and other compliance issues. One way to address this is by adopting a "compliance by design" approach. This means incorporating regulatory considerations from the very start of AI model development. By embedding these elements early on, we can mitigate many of the compliance challenges that could arise later during the model's development and deployment.
C: How do you see AI continuing to transform the customer experience in banking, particularly in areas like payments and personal banking?
Dinto Jose: If we look at personal banking today, most of what we get is standardized products. In the future, I expect to see more personalized services. Banks won’t need more people to deliver this—they’ll use data to analyze customer behavior, create patterns, and offer personalized recommendations.
The second area is the payment experience. Currently, when payments move from one country to another or between individuals and corporations, there are strong regulatory controls and risks involved. As AI and technology advance, these processes can become more streamlined. AI can help assess and manage risk more effectively, which could result in a smoother, more seamless payment experience for customers.
Risk controls are not going to go away, but the efficiency of these controls will be significantly increased, which will result in smoother customer experience.
Another area I see transforming is proactive customer support. Today, we contact customer service when we have an issue. But in the future, we could see customer service reaching out to us preemptively, identifying potential problems based on our transaction behavior and offering solutions before we even realize there’s an issue. This kind of proactive support could become common as AI and machine learning continue to develop.
C: You mentioned how AI might affect payments. Do you think that will be the next big trend in payment solutions, or do you see something else on the horizon that could be transformative?
Dinto Jose: One trend we’re seeing is embedded finance and invisible payments becoming more mainstream. This essentially means financial services are being embedded into non-financial platforms. For example, with Uber, you travel and pay, but you don’t really think about the payment—it happens in the background. Similarly, with Amazon Prime, you receive the service without actively processing the payment. This concept of embedded finance and invisible payments will become more common in our everyday lives.
Another area is the reduction of payment acceptance costs for merchants. Right now, there are significant costs involved when merchants accept payments. With Open Banking and QR code-based payments, I expect to see these costs drop, making it easier and cheaper for merchants to accept payments.
The third area is cryptocurrency and stablecoins. A few years ago, cryptocurrencies were seen as speculative and risky. But recently, we’ve seen stablecoins and cryptocurrencies gaining more traction, being used by different financial service providers. This will likely continue to grow and impact the payments landscape.
C: How do you see AI reshaping fraud detection, money laundering detection, and compliance processing in banks?
Dinto Jose: Risk management is at the core of banking—if you ask any banker, they’ll tell you that banking is 50% risk management. One of the biggest challenges in this space is the high number of false positives.
Often, what appears to be fraudulent activity turns out to be normal behavior after manual investigation. With the use of data-driven analytics—whether you call it AI, machine learning, or advanced predictive analytics—I expect we’ll see a reduction in false positives over time as the models become more efficient.
The second area is prioritization of investigations. When suspicious activity alerts are triggered from AML models, operational teams need to manually investigate them. The challenge is in deciding which alert to investigate first. AI can play a significant role in helping prioritize these investigations based on historical data.
Finally, predictive analytics will become more prominent. By using historical data, banks can predict emerging trends and expected behaviors, allowing for more proactive risk management. So, in risk management, we’ll see three key changes: fewer false positives, efficient investigation of suspicious activities, and greater reliance on predictive analytics, all driven by AI.
C: When you're working with your team, how do you foster a culture that embraces AI and innovation while ensuring they’re prepared for the rapid changes in technology and regulations?
Dinto Jose: When people from the business side hear about AI and machine learning, their initial reaction is often, “That sounds very technical; it’s not my area.” They might feel like it’s something for their tech colleagues to handle. But with more tools becoming available, there’s a lot more that can be done from the business side to be a data driven organization.
The first step is fostering a culture of continuous learning and upskilling. This is essential for helping colleagues adopt new technologies, increase productivity, and free up time for more valuable work.
The second aspect is promoting experimentation and innovation. When dealing with new technologies, people need to feel comfortable testing ideas, even if they fail. Failure is part of the process, and if you don’t encourage experimentation, no one will take the initiative to try new things. As leaders, it’s crucial to create an environment where new ideas can be tested and explored, with the goal of finding ways to bring more value to clients.
The third key element is building a data-driven culture. Traditionally, decisions might have been based on experience or using basic tools like an Excel sheet. But real data-driven decision-making is still gaining traction. Leaders need to promote this shift by making data accessible and encouraging data literacy across the organization. You don’t need to be a data scientist to gain insights about customer behavior and figure out how to better serve them. Leadership needs to push this data-driven mindset from the top down to ensure everyone can leverage data effectively.
_____
Register now for CDAO Frankfurt to hear directly from Dinto and other top professionals in Germany about the future of data, analytics, and AI