How Data is a Cause of Risk: ANZ Bank's Trisha Lee
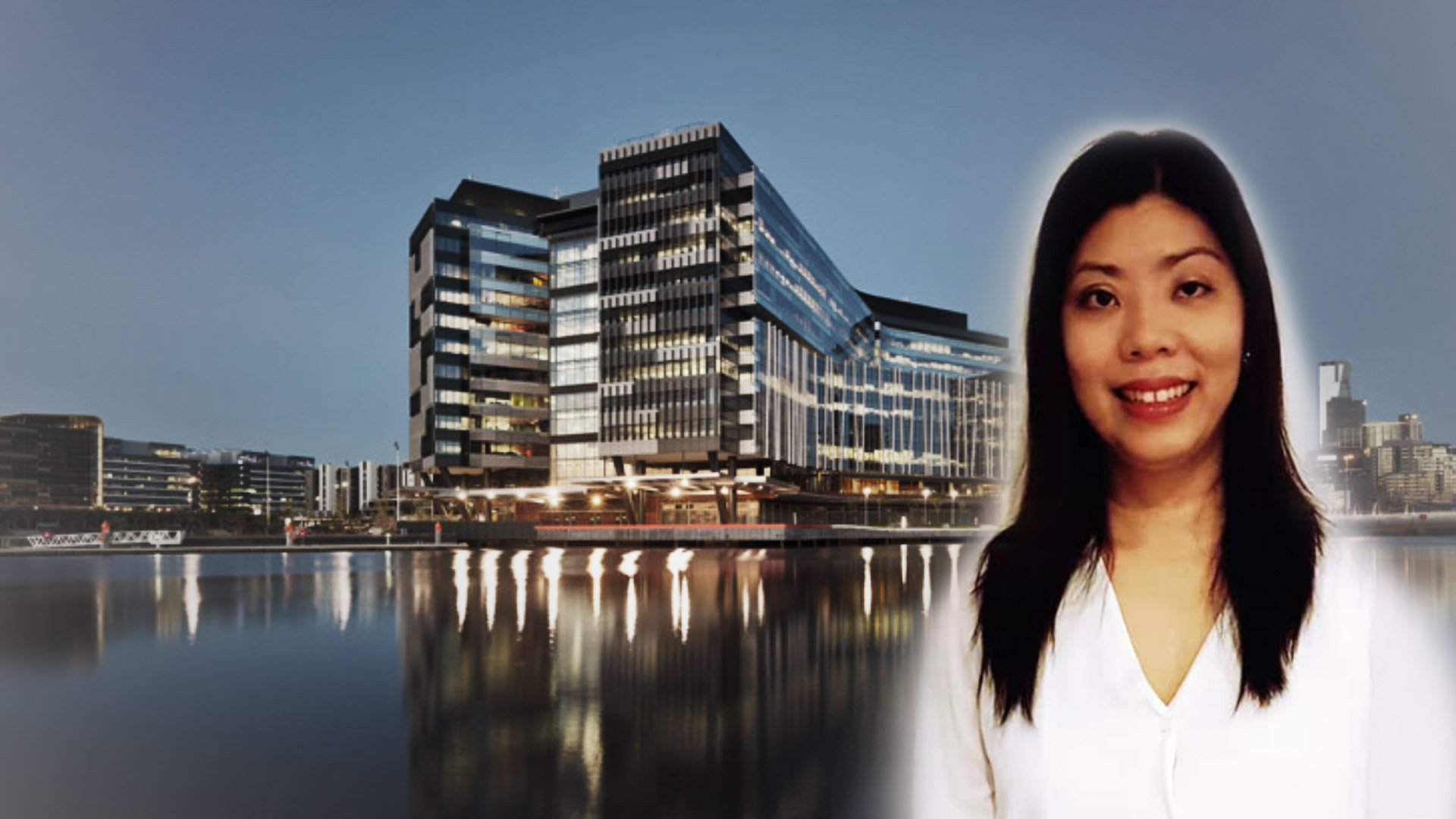
ANZ Bank Head of Data Risk and Compliance, Trisha Lee, discusses driving changes in thinking and culture around data and risk at ANZ
Organizations that don’t consider how data is contributing to their business risks are missing out on adopting data governance controls to mitigate these risks.
That’s one of the key points ANZ’s Head of Data Risk and Compliance, Trisha Lee, makes after spending more than a decade at the bank covering both risk management and data governance.
For the past five years, Lee has been working to bring greater data governance to all areas of the bank. She took the approach of driving data governance into the organization through the lens of risk management, which has the benefit of getting the focus and funding it needs.
Rather than introducing a data governance framework outside ANZ’s long-standing and trusted risk framework, Lee and her team integrated significant parts of their data governance approach and tools within the current enterprise risk framework. This helped stakeholders understand how improving data was not just a ‘nice to have for the future,’ but integral to improving how the organization manages its present critical business risks.
Most organizations understand the value of good data governance. But they don’t necessarily see fixing longstanding data issues as a critical priority given post-covid financial, customer, operational and innovation demands.
This is why it was important for the team at ANZ to talk about data risks from the perspective of business risk management rather than as part of good enterprise data management practice. Ahead of her speaking engagement at Corinium’s CDAO Deep Dive: Data Governance Online A/NZ, Lee sat down to chat with Business of Data.
“When organizations, particularly financial institutions, understand how data contributes to or impacts key business risks; they will then understand that good data governance is a valuable contribution to risk management; this helps drive the right risk mitigation outcome by introducing the right data governance controls,” Lee says.
“As an example, think about something like business continuity. In the past, organizations may identify the systems, hardware or buildings that may be impacted during a disruption event. However, organizations may not have thought of the type of data needed to arrive at the decision. When an event happens, would they have the right data to contact their staff? Are the organizations’ systems updated with accurate data? Is it complete? This is not front of mind because it is not linked to a data cause or a data risk. It has traditionally been thought about as being an operational risk.
“There has been less effort focused on looking at the quality of the data or identifying the data required for decision making. If you don’t identify that you need good quality data or that the data needs to be complete or accurate, then you won’t see the connection between poor data quality and business disruption. This means it won’t even cross your mind to consider a data governance type control as an option for mitigating the original risk, business disruption.
“At ANZ we have reframed the discussion about data and business risks by introducing a new concept: data as a cause of risk. This means asking teams to identify where and when poor data is impacting current business risks. Poor data could be incomplete data or missing data or data that is duplicate and therefore inconsistent. By identifying poor data as a cause of risk, you broaden your options for suitable controls to manage this risk.
“Historically, everyone looks at data from the perspective of information security. For example, did someone hack into the data or systems or did someone try to steal the data? But data governance asks different questions: are you missing data or do you have duplicate records of your customers or employees and can’t tell which one is the most accurate? Most don’t differentiate between data risks or data as a cause of risk and information security risks with the data. They are completely different.
“This is where we have changed the debate by expanding how we think about the ways data can impact business risks. We found that integrating the data governance debate into the risk management debate and framework was the way to go; by introducing data as a cause of a risk, to make sure the impact of poor data is understood and then introducing the correct controls.”
Controls on Data
Data controls can be quite simple and sensible. They can range from adopting reference data like let’s all make sure data controllers use AU instead of AUS, to names and phone numbers conforming to length and character rules, to requiring a large amount of tagging.
“Controls help get consistency on how the data is captured. We are trying to map data lineage so that we know where the data is moving across its lifecycle, and you can put in place controls to ensure the data does not change as it moves,” Lee says.
“Metadata is very important as well. A lot of times data is created and not tagged correctly, so you can be half-guessing what the data is meant to be or what it’s for. By the time it gets downstream, people have no idea where it came from, no idea what it was used for, or how it changed and then it can be used in the wrong way or bastardised.”
Another measure Lee says her team is taking is to create an authoritative source of where the data comes from. If it’s customer data, all users must be authorised and can access the data from the same system, rather than have it available across several systems with inconsistencies on which is the most up-to-date: the golden source. This is important because it enables data to be trusted.
“I think that is something a lot of organizations are struggling with because there will likely be multiple source systems, and you need to define which one will be the key,” Lee says.
Lee also adds that while some controls may sound simple on the surface, the ripple effect of poor data controls can be profound.
“An example I can use from a marketing perspective, in the past a default number used to be entered if a customer did not provide a mobile number. The number they were inputting actually belongs to a person in Australia,” she says.
“Apparently many banks used the same default number, and this person gets thousands of messages every day. He also won’t change his number because he loves it, and I don’t blame him!
“So we introduced a control whereby if anybody tries to input that number, it will be rejected automatically by the system.”
Incomplete or incorrect mobile numbers can also be a concern if the bank should need to alert a customer about suspicious activity taking place on their account.
“There are many possible implications. There are examples where if we don’t capture the information correctly we could cause harm to an individual. It seems simple but it can have huge impacts.”
Integrity on Lifecycle
Putting controls around new data is one thing, but financial institutions are often dealing with customer data that stretches back decades, so data controls must also involve constant monitoring and alerting as data moves across systems for various uses.
“We need to identify what we call hops across the system and ensure, depending on what data is moving, that there are integrity controls that we can introduce,” Lee says.
“To monitor these controls we actually create a dashboard which will report whether or not the integrity of data it monitors has deteriorated or if the quality is not where we want it to be from a tolerance level perspective.”
Lee says if it was possible, starting from scratch would be the best way to ensure great data, given all we know now compared to decades past.
“We need to think about capturing customer information right the first time,” she says. “What organizations don’t always think about is for every product or reason that data is collected, how in the long-term will that data be handled and how many users will use it.”
Lee adds that questioning who gets access to certain data and understanding the use cases forms usage rules that further control the integrity of data and the insights that organizations produce. Putting all of those in place at the foundation of an organization would be ideal.
“As a company is formed and it expands and diversifies its offerings, the users and uses of the data starts to change,” she says.
“In turn, your requirements for data governance will start to change. You need to be able to go back, review and ensure the sources of your data remain fit for purpose. This will be a big challenge for a lot of organizations adapting to important data ethics trends today.”
This is where data governance is playing a key role, not just for managing critical business risks, but more importantly for helping organizations trust their data.