How Aegis Insurance is Overcoming Data Discrepancies to Price Catastrophic Risk
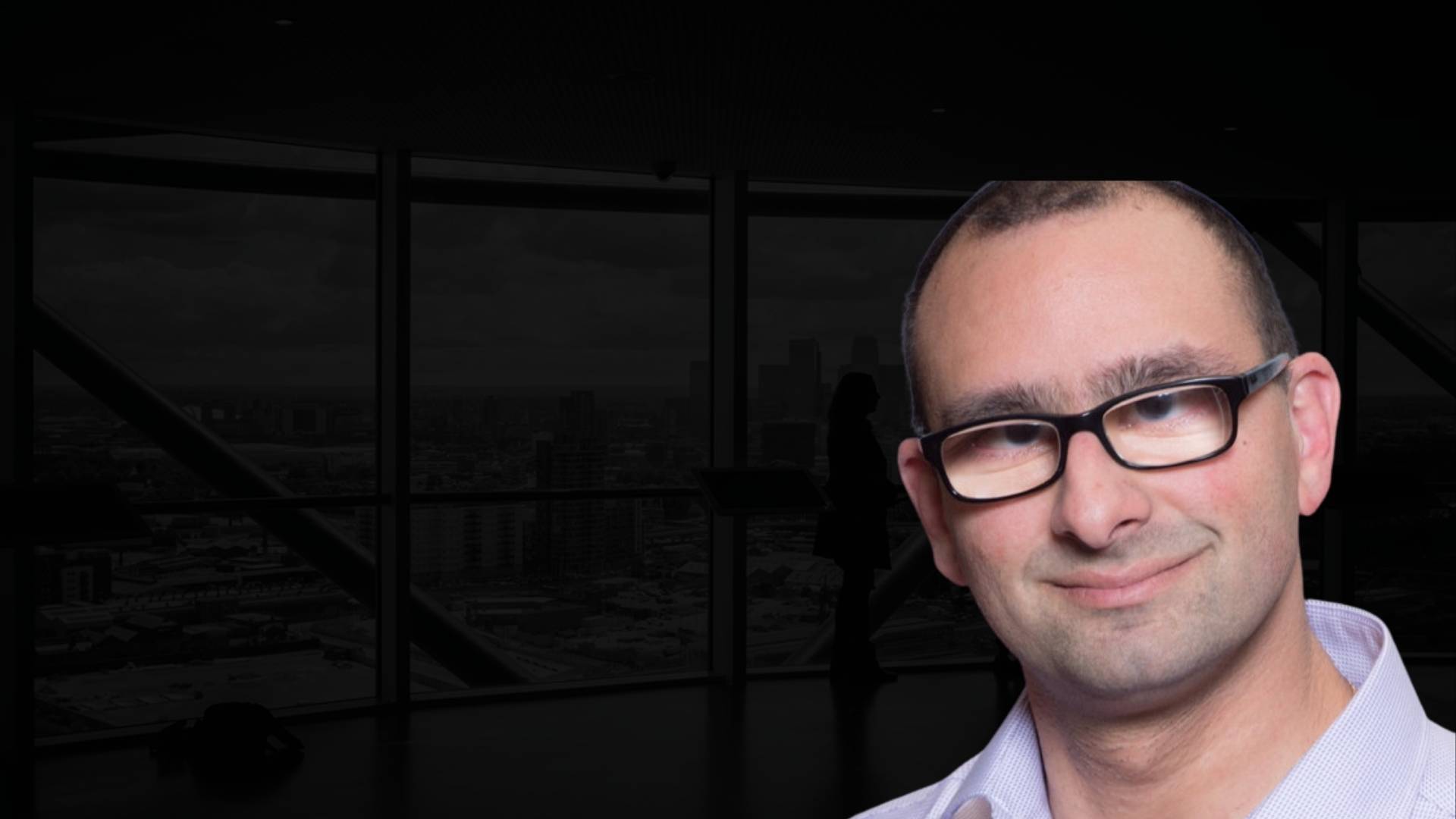
Dan Marzouk, Senior Vice President of Data Science at Aegis, explains how data science is shaping their approach to insurance
Wildfires are difficult to predict, grow rapidly and have the potential to cause damage worth tens of billions of US dollars.
This is a problem for insurers trying to price risk. The solution? Using data to develop a more complete understanding of risk, argues Aegis Insurance Senior Vice President of Data Science Dan Marzouk in this week’s episode of the Business of Data Podcast.
When evaluating, for example, the chances of a wildfire affecting a suburban home, there are a wide range of data points to consider and a variety of data sources to include. However, not all sources are of equal quality.
“The challenges are similar to comparing a Google review, a Yelp review and a Facebook review for a business. Each of those [reviews] have their pros and cons,” Marzouk notes. “Each of our data sources also have their pros and cons.”
The differing quality of data sources can lead to discrepancies in the data. That’s where data science comes in. Creating a consistent risk assessment requires building a model that quantifies the accuracy of input data.
“Over time we start to learn and utilize what we think is accurate from one dataset and continue on that path to build our own data integration system that understands what we believe to be the most accurate system,” says Marzouk.
Of course, weighing tens of thousands of data points takes time. However, as Marzouk explains, in the age of instant everything it is crucial to provide insights to decision-makers quickly.
“To do that, we have to both understand how to aggregate that data quickly and cull out what’s not as important or useful,” he says. “And be able to develop something that the underwriter can make a decision on quickly.”
Ultimately, to meet the business need the data must help to create a product that is appealing to the customer. That means that data scientists must also maintain a commercial awareness.
“Customers don't buy things because you told them that the model says [they’re] going to buy it,” Marzouk quips. “That's my advice to the data science community. Take a step back and say, ‘I know the data's telling me this, but does it make sense?’”
Key Takeaways
- Understand the data you have. Is it granular enough? How reliable is the source? The answers should inform your model.
- Maximize your data points. Innovative technologies like image recognition can dramatically increase the number of data points available
- Take a step back. Remember to evaluate what the data is telling you in the light of all other available information