How to Scale Data and Analytics Initiatives More Successfully with AnalyticsOps
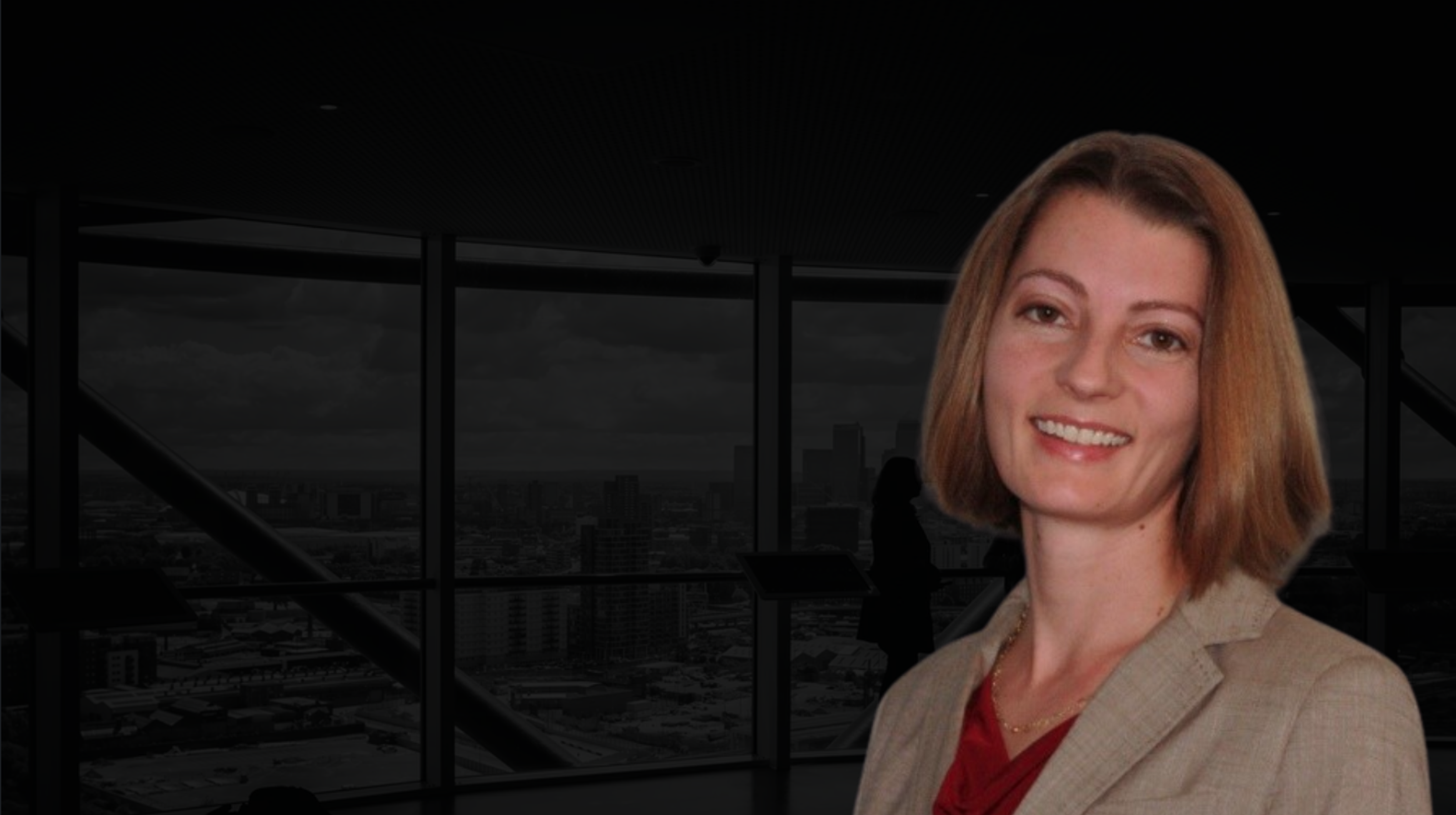
Laura Spencer, Head of Data and Analytics at Sendle, shares her tips on implementing AnalyticsOps for better business productivity and performance
Data and analytics teams have an automation problem. Research from software firm Alegion shows that as many as 96% of AI initiatives fail to enter production. Similarly, Gartner has predicted that only 20% of analytics projects will deliver positive business outcomes through 2022.
In this week’s episode of the Business of Data podcast, Laura Spencer, Head of Data and Analytics for international shipping company Sendle, argues that analytics ops may be the solution to this pervasive problem.
“Analytics without action is just expensive artwork,” Spencer says. “To provide benefit it really needs to be embedded in the company's day-to-day processes.”
She continues: “Too often, good analyses just grow out of date because they're not automated, or good models go unused because they can't make their way into a stable production environment. Analytics ops is really all about enabling companies to do this in a scalable and sustainable way.”
Paving the Way for AnalyticOps
As some of our recent research revealed, businesses are digitizing rapidly, and the pandemic has accelerated this trend. As a result, data and analytics teams and the insights they provide are in demand.
However, busy teams can create challenges for data and analytics leaders seeking to implement AnalyticsOps, especially if their teams struggle to see the immediate benefits.
“I think [analytics ops] really should be a foundational pillar of a data and analytics team, and internally I find you often have to sell it to your own team,” Spencer explains.
“[It’s about] really focusing on how it’s going to improve their day-to-day, reduce repetitive tasks, reduce the impact of fire drills that are going to come up from unmonitored systems that are breaking,” she continues. “It increases the value that they can drive across the business.”
Fundamentally, Spencer explains, promoting the benefits of AnalyticsOps is like promoting any other data and analytics initiative. Start small and grow.
“Start with a few proof of concepts. An easy place is often to look at some of your ad-hoc requests that are probably candidates for automation,” Spencer advises. “These quick wins then can help you build some momentum for that future investment.”
Overcoming Common Barriers to Adoption
Implementing a methodology like AnalyticsOps requires support from senior leadership. This also may require salesmanship, especially when change comes with a hefty price tag.
“One of the key barriers that I’ve seen is really that initial investment and the associated impact on cost and speed,” Spencer says. “It's going to be much quicker just to run something ad-hoc than to take the time to automate it.”
She continues: “But really, by automating you're trying to empower the stakeholders long-term. They're going to be able to track these things over time, receive fewer ad-hoc requests, and hopefully drive more value because you're enabling data-driven decisions through better democratization of the data.”
Another common barrier to adoption is the lack of analytics practitioners with the necessary skill set to successfully scale data and analytics initiatives beyond initial use cases.
“This is really not a typical skill set for most analytics practitioners to have,” Spencer notes. “Standard analytics or data science coursework, for example, is largely [focused on] one-off ad-hoc projects that are meant to teach a very specific analytical methodology. It's not focused on things like version control, coding best practices, efficiency, and scalability.”
To address this skills gap, Spencer suggests engaging software engineers who are interested in automation, machine learning and data and analytics.
“What has helped for me is to look internally [to] folks that know your systems. I’ve found that there tends to be a lot of intrigue among the engineers and finding somebody internally and bringing them on board has been the way that I’ve found success.”
Ultimately, transitioning successfully to an agile, AnalyticsOps methodology requires data and analytics leaders to be laser-focused on selling the long-term benefits to the business-at-large.
“Find the pain points, because those are often easier to sell across the company, and then understand how AnalyticsOps can really help you with that,” Spencer concludes. “Start small and have some of those wins and start to build the momentum.”
Key Findings
Make sure your analytics is not artwork. To make an impact, analytics needs to be embedded into day-to-day operations.
Sell the long-term business benefits. While the initial investment of time and money may be high, the dividends will be paid in efficiency and productivity.
Plug skills gaps with in-house talent. Software engineers can provide essential skills for scaling data and analytics initiatives successfully.