What Companies Get Wrong About AI
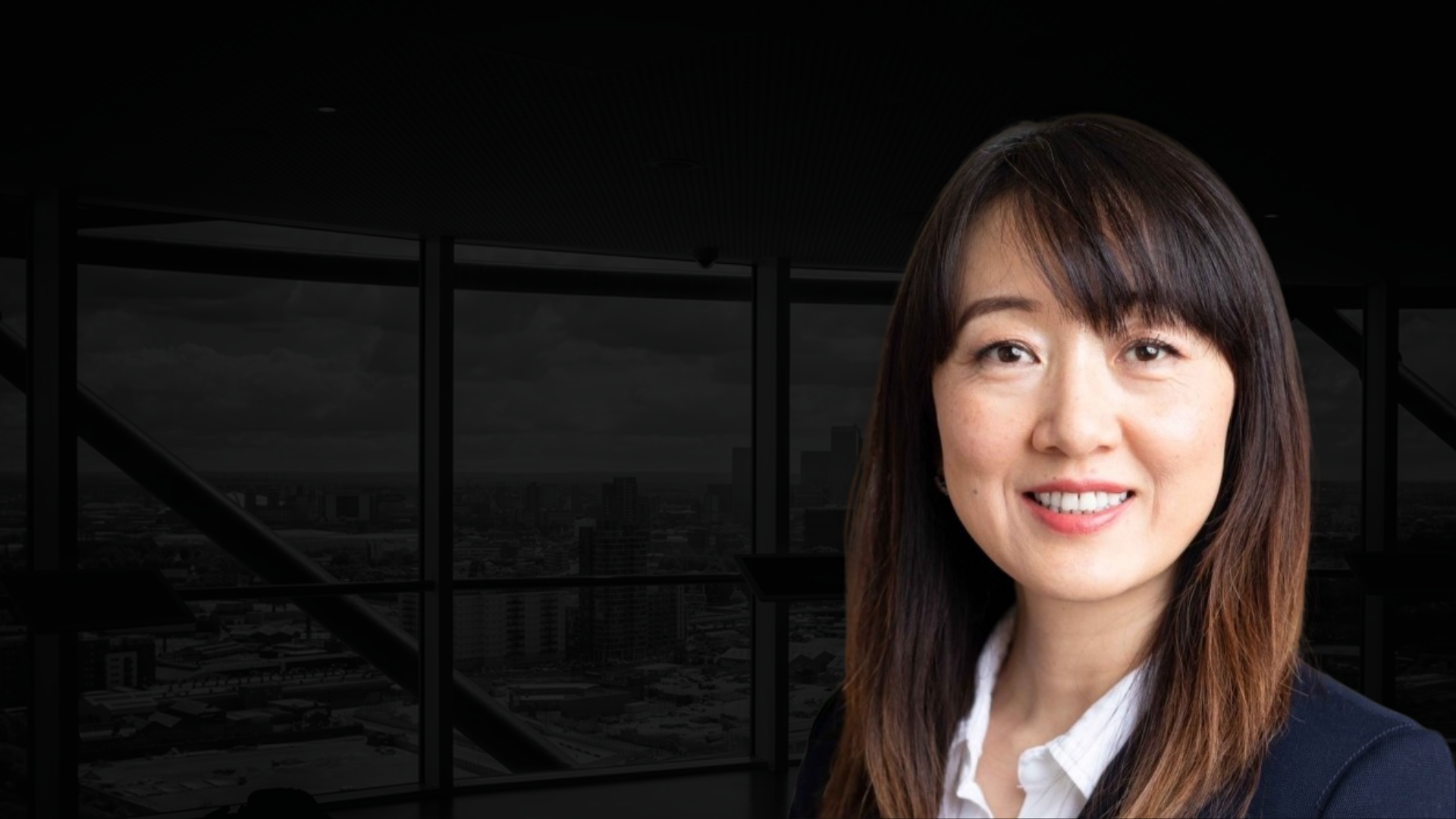
Wendy Zhang, Director of Governance and Data Strategy at Sallie Mae, discusses why companies must put the right culture, value and quality into their AI initiatives
Since Gartner’s famous proclamation that 85% of AI projects end in failure, the maturity of enterprise AI functions has improved dramatically. But the high number of projects that continue to end in failure suggests that many companies are still getting the basics of AI development wrong.
In this week’s Business of Data podcast, Wendy Zhang, Director of Governance and Data Strategy at banking company Sallie Mae, shares her views on why so many AI projects don’t deliver results.
For Zhang, common issues like poor data quality, trouble identifying valuable applications for AI and lack of buy-in for investing in or adopting AI technologies are symptoms of a more basic problem.
“There are a lot of different reasons [AI projects fail],” she says. “But it all starts with a lack of fundamental understanding of AI, what it is and what it can or cannot do.”
AI Success Starts with Asking the Right Questions
Zhang warns against doing AI for the sake of AI. She argues that companies must start with the business challenges they need to solve before considering what value AI might bring to these initiatives.
“The next [thing] you have to really assess is, is this something that AI can actually do?” she continues. “Is this appropriate for the business problem you’re going to solve?”
Once an AI-focused executive has identified projects that could benefit from AI-driven technologies, they must consider what they need to deliver these projects successfully. This includes assessing what resources, funding, datasets and support they’ll need for each project.
“It’s really got to become the company’s DNA,” Zhang adds. “It requires people to really look at a lot of your business processes and to think about different possibilities, and that requires mindset change.”
“It’s not so much working and doing the same things over and over and just automating a few things and having AI on the side,” she concludes. “If you really want to get a massive benefit, you have to be able to experiment and fail and also incorporate that into your core business.”
Simpler is Often Better for AI Beginners
When companies are new to AI, they typically don’t have fully formed strategies for adopting these technologies. It’s more common for enterprises to begin experimenting through trial and error to discover the types of AI systems that are relevant to them.
When starting out on this journey, it’s good practice to start with projects that can be delivered using data the company already has. The sooner they are implemented and delivering value, the better.
Similarly, Zhang notes that simpler AI models can be easier for fledgling AI teams to deliver. Even for more advanced AI functions, she warns against overcomplicating AI systems unnecessarily.
“I think of simple models as, in plain terms, you get more bang for your buck,” she quips. “The more complicated the models are, the harder it is to have a higher interpretability.”
“The other component is having the right people,” she adds. “It’s important to build AI capabilities in-house. However, when you first start out with a pilot project, it might be beneficial to get external help, just so that you can get the ball rolling and gain some momentum.”
“You really have to go through a lot of trial and error,” she concludes. “Start with pilot projects to score some small wins to get some buy-in and build your credibility to get faith for your team.”
Key Takeaways
- Start with the right questions. Only embark on AI projects when they’re the best answer to a pressing business question
- Find use cases you can deliver with what you have. Identify what data, resources and support you’ll need before you begin
- Simpler is often better. As any engineer will tell you, the more parts something has, the more bits there are that can break