Leading on Data Governance and Business Strategy: Patrick Chew
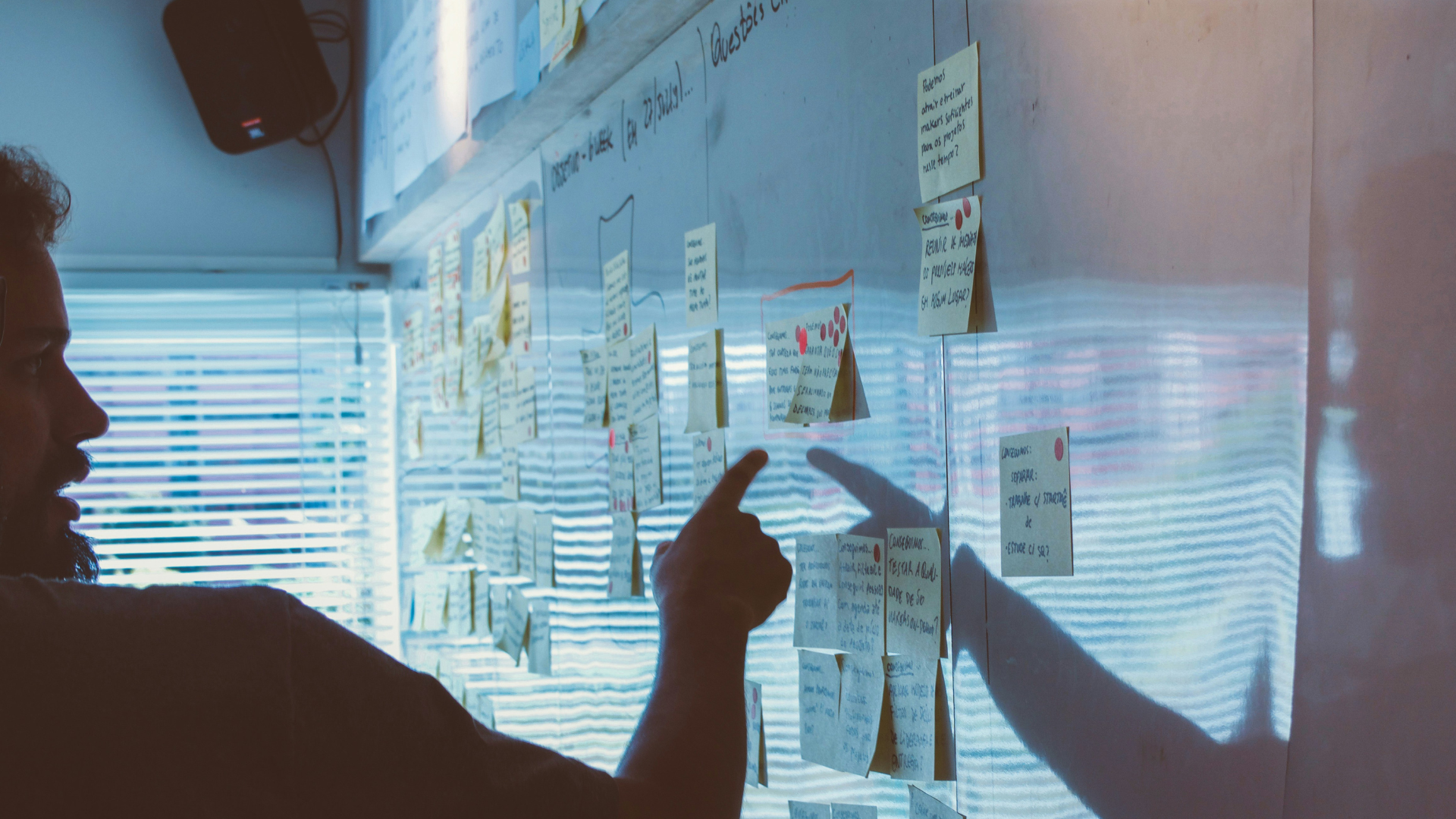
Patrick Chew, Global Head of Data Enablement at AIT Worldwide Logistics, explores the practical applications of AI in data governance and business strategy, and what it takes for CDAOs to lead these initiatives effectively
As a Global Head of Data Enablement, Patrick Chew drives initiatives that harness the potential of AI and data analytics to ensure insightful and informed decision-making. His approach focuses on the essential elements of data strategy, its alignment with business goals, and the importance of fostering a data-driven culture within organizations.
Ahead of Patrick’s talk at CDAO Chicago, kicking off on August 7th, we sat down to discuss the critical responsibilities of data leaders in driving enterprise AI initiatives, the need for strong business sponsorship, the cultivation of a robust data-driven culture, and the strategic management of talent in the era of AI.
For those interested in the future of AI in business, his insights will be invaluable.
Check out the full agenda and register for the event here.
Could you discuss the importance of a robust data strategy in supporting business goals and objectives, particularly in leveraging AI?
Patrick Chew: Establishing a solid data strategy is crucial because it underpins your entire business framework. This strategy supports your business goals and objectives, guiding the organization’s vision and mission. Once you have a clear data strategy, you begin to naturally inquire how you can achieve these business strategies within the established goals and overall vision.
Breaking it down into manageable, achievable initiatives is key. These initiatives need prioritization and should align directly with why you’re looking to implement AI or develop analytics platforms. The core of this approach involves deriving business objectives that are in sync with the company’s mission, which then shapes your strategy and approach.
Asking the 'why'—why we are pursuing certain projects, why we are aiming for specific outcomes—leads naturally to discussions about value creation and realization. It becomes almost intuitive to then measure the effectiveness of these initiatives, whether they’re AI or analytics-focused, and assess whether they're meeting targets, delivering value, and creating a meaningful impact.
In your view, how should CDAOs measure business value from data and analytics, and what common challenges do they encounter in proving ROI?
In my experience, there are two major challenges. The first is the inability to directly link data to tangible outcomes. It's crucial to establish a clear association between the data initiatives and the outcomes they're meant to drive. Without this link, it’s challenging to demonstrate the value and impact of the data strategy.
The second major challenge involves distractions during execution. Even with solid plans in place, organizations can lose sight of their objectives. Often, this occurs because other pressing needs divert their attention and resources, diluting their focus on the original data initiatives. This leads to a wavering commitment and can result in poorly defined objectives and goals that are hard to measure, track, and manage.
Additionally, there’s an overarching theme in many organizations: starting with a solution in search of a problem. It's essential to begin with clear, defined problems and use data and analytics to address these specific issues, rather than adopting technologies without a strategic purpose. This approach prevents misalignment and ensures that initiatives are directly tied to solving real business challenges.
Can you share insights on the importance of business sponsorship in data projects? How should data leaders cultivate these relationships to ensure project success?
Patrick Chew: Sponsorship is absolutely vital. Any initiative driven from the bottom up, particularly IT-driven projects, is at high risk of failing if it lacks strong business sponsorship. This is because technology serves as an enabler rather than the primary driver of business. I strongly advocate for incorporating business leadership from the outset — what I refer to as bringing them into the traditional design-build tent.
Ensuring that the business leaders are directly involved and understand what you are attempting to solve or achieve is crucial. This shared responsibility means that both IT and business units are invested in the outcomes; if challenges arise, they are faced together, and successes are celebrated jointly.
The linkage between business sponsorship and project outcomes — value creation and realization — cannot be overstated. Effective sponsorship ensures that the project aligns with business strategies and goals, enhancing the likelihood of success. Without this alignment and shared commitment, even well-planned initiatives struggle to gain traction and produce meaningful results. It’s essential that these relationships are nurtured to ensure that both the vision and operational execution are aligned, ensuring that initiatives not only start strong but also maintain their momentum to successful completion.
What are the key components of a data-driven culture that every organization should aim to establish, regardless of industry?
Patrick Chew: Establishing a data-driven culture involves understanding and integrating six core “KY” (know your) areas, which I've detailed in an article previously published in CIO Review Magazine. These areas are crucial for any organization aiming to leverage data effectively:
• Know Your Business: This is about understanding the fundamental reasons why your business exists. What products or services are you offering? What processes are you creating for your customers? This knowledge is the cornerstone of all data initiatives.
• Know Your Process: Detailed knowledge of your business processes is essential. It allows you to optimize and streamline operations through data.
• Know Your Data: This involves not just accumulating data but also understanding its relevance, the insights it can provide and the actions following
• Know Your Culture: Your organizational culture should support and drive data initiatives. It’s about creating an environment where data-driven decisions are valued and promoted. The biggest threat for any organization is one that doesn’t accept failure, ideas from outside or cannot change.
• Know Your Talent: Assessing and understanding the skills and capabilities of your workforce is vital. It's important to identify if there are gaps in skills, especially with emerging technologies like AI.
• Know Your AI Governance: This pertains to understanding the guidelines and frameworks that govern your AI initiatives, ensuring they align with your business ethics and compliance requirements.
These six areas are interconnected, starting from a deep understanding of your business to how your organizational culture and governance structures support your data strategies. By addressing these components, organizations can lay a solid foundation for a robust, effective data-driven culture.
This approach ensures that all data-related activities are aligned with the broader business objectives, enabling better decision-making and more strategic use of technology.
The conversation around talent in data and analytics has shifted in recent years, particularly with the advent of AI. Could you share your perspective on how these changes are influencing the talent market?
Patrick Chew: There's a common misconception that AI will replace all jobs, which isn't entirely accurate. It’s crucial to understand the context. AI will indeed impact certain roles, like those in customer support or HR call centers, where chatbots can handle routine inquiries. This automation allows organizations to redirect their workforce to more strategic tasks.
However, the critical takeaway here is the necessity for companies to adapt and capitalize on AI. It's not that AI will take over entire functions; rather, competitors who effectively leverage AI will outpace those who don’t. This brings us to the importance of upskilling our workforce. Organizations must ensure their teams are AI-literate and understand how to use AI to enhance business functions, whether that’s increasing market share, improving processes, or applying emotional intelligence more effectively.
This approach to talent development helps foster an environment that supports staff retention by making them feel valued and integral to the company’s evolution in the AI landscape. In essence, the goal is to use AI not as a replacement but as an empowerment tool that augments the capabilities of the existing talent pool.
This is the best way to capitalize on AI tools and capabilities, to use it with all available data points to help find your differentiator. After all, it’s what distinguishes you from your friendly or foe competitors that counts!
How would you advise data leaders to identify the most appropriate applications for this technology and convincingly present these ideas to senior leadership and stakeholders?
Patrick Chew: Identifying the right use case is crucial—it acts as a litmus test. When I propose a new AI initiative, I start by outlining the specific use cases and discussing with stakeholders the expected value and impact. It's essential to start small and demonstrate value quickly, leveraging today's AI technologies, which allow for rapid testing and results. This approach helps in quickly showcasing the benefits without the long lead times traditionally associated with technological implementations.
Moreover, it’s vital to never sideline human validation. Over-relying on AI without human oversight can lead to significant issues, from regulatory fines to reputational damage if things go awry.
Always ensure that AI initiatives are supported by strong business cases and clear value propositions to engage stakeholders effectively. Also, it’s important to synch up your data governance and AI relationship. Being aware of these stages will help you significantly in managing your risks or tolerance level as depicted here.
Finally, it's about continuous improvement and recalibration. That’s the basis of AI or GenAI – recursive self-improvement. Begin with small-scale projects, evaluate their success, and then gradually expand. This methodical approach prevents the common pitfalls of scaling too quickly and ensures that each phase of implementation is robust and successful before moving on to more ambitious projects. This strategy not only secures buy-in from leadership but also builds a strong foundation for wider AI integration.
Want to learn more?
Patrick Chew is speaking at CDAO Chicago, kicking off on August 7th, 2024. Join him and many more world-class data and analytics leaders to learn more about the data and analytics trends driving value creation. Register to attend here.