Data Mesh Concepts Top the Financial Services Agenda at CDAO Fall Virtual
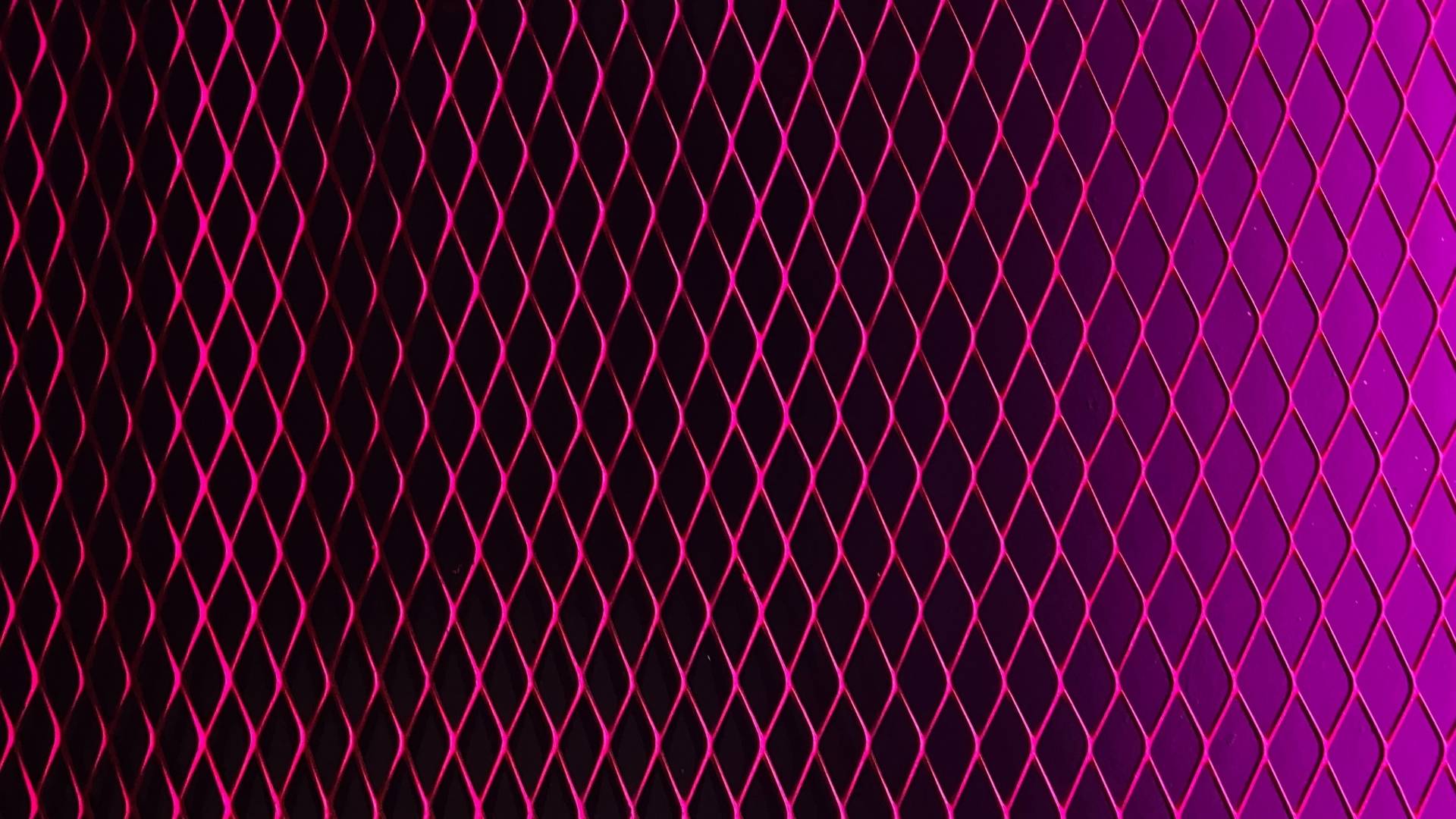
Data mesh concepts such as analytics self-service and decentralized data governance were ‘top of mind’ for the financial services executives who spoke at CDAO Fall Virtual 2021
We expected the much-hyped ‘data mesh’ concept to be a key talking point at Corinium’s CDAO Fall events this year. So, it was hardly a surprise when the financial services executives who spoke during the industry focus days at CDAO Fall Virtual proved eager to talk about how they’re applying data mesh concepts in their companies.
In essence, data mesh is a collection of ideas about how enterprises should manage big data for analytics. They include: 1) decentralized data ownership and architecture, 2) serving data to business domains as a product, 3) self-service data infrastructure to enable autonomous, domain-oriented data teams and 4) federated data governance.
As Mike Oppenheim, VP, Data and Analytics at Discover Financial Services, said, many of these ideas are starting to be seen as essential for the success of digital transformation projects.
“We’re on a journey to develop much more of a producer/consumer type of environment, where the producers are the experts in their data,” Oppenheim said. “That concept of data producers creating and owning high-quality data and the consumers trusting it and using it in the right way, I think, is essential to making this whole digital transformation work.”
Pairing Data Governance with Domain Expertise
Speakers at CDAO Fall Virtual agreed that financial services companies must build truly integrated digital ecosystems to succeed with their digital transformations. However, many have struggled to achieve this using traditional approaches to data management and analytics.
“It’s tough but critical to build that kind of an ecosystem,” said Deep Srivastav, SVP, Head of Digital Solutions at investment management firm Franklin Templeton. “It means an ability to capture information, transform information, convert it into something which is insightful and be able to feed it back to those clients or decision-makers.”
Historically, cumbersome data governance and access processes have meant it takes too long for analytics teams to get business stakeholders the insights they need. Meanwhile, a lack of domain expertise in centralized data teams has made it hard for them to manage data quality effectively.
To address these challenges, many executives who spoke at CDAO Fall Virtual have built cross-functional teams embedded in business functions and are empowering them to own and govern and own their own data.
“Knowing what you’re measuring and what you’re striving for; I think that’s where the conversation starts”
Alex Golbin, Chief Data Officer, Morningstar
“[You need] to have cross-functional teams that go and make decisions as close to the work as possible,” noted Oppenheim. “You have to have absolute trust in the data you’re using, and that only happens when you have the appropriate structure and pieces in place.”
“People who create the data or modify the data need to be accountable for its quality,” added Jay Franklin, VP, Enterprise Data and Analytics at First Tech Federal Credit Union. “Being able to compensate for the lack of consistent understanding by putting a cross-functional team together is a pretty good way to make sure that you have more trust in the insights.”
Bringing the Analytics to the Data
When it comes to using data to generate insights, Alex Golbin, Chief Data Officer at investment research firm Morningstar, noted that the biggest challenge is often feeding models with the right data.
“I’ve probably spent countless hours, weeks and months, building data extraction, moving those files over and building the whole ecosystem to make sure that the movement happens [and] that at the destination you’ve got all the data you sent at the source,” he said.
“About a year and a half ago, we pivoted the direction of travel,” Golbin continued. “Instead of extracting data and moving it to analytics, we’re now moving analytics to the source of data. And we’ve discovered that moving a model is much easier than moving the terabytes of data.”
Rather than moving data from the central repository to the analytics model, Golbin’s team is achieving greater agility through building analytics layers on top of Morningstar’s data stores.
“That actually makes it easy and also creates this notion of ‘citizen developers’, of democratizing your data science,” Golbin reported. “Once [we’d] actually moved people towards data, versus the other way round, time to market had actually shrunk significantly.”
Through adopting this kind of data architecture, executives can embrace the idea of ‘data products’ and enable business users to generate new insights more quickly through reusing existing models, features and code.
“I love the concept of bringing the models to the data,” agreed Oppenheim. “I think this is absolutely critical.”
Data Mesh: The Future of Financial Services Data?
Some have argued that the term ‘data mesh’ is just a new way of describing what the leaders in the data and analytics space are already doing. Indeed, it is possible that the financial services sector was moving towards these paradigms before the phrase ‘data mesh’ came along.
But, whether or not this is the case, it is looking likely that the practices and methodologies that make up the data mesh concept will form the basis for a great deal of the financial services sector’s data and analytics innovation in the coming months.