Gareth Hagger-Johnson: Tackling Algorithmic Bias for Fair Credit Outcomes in Financial Services
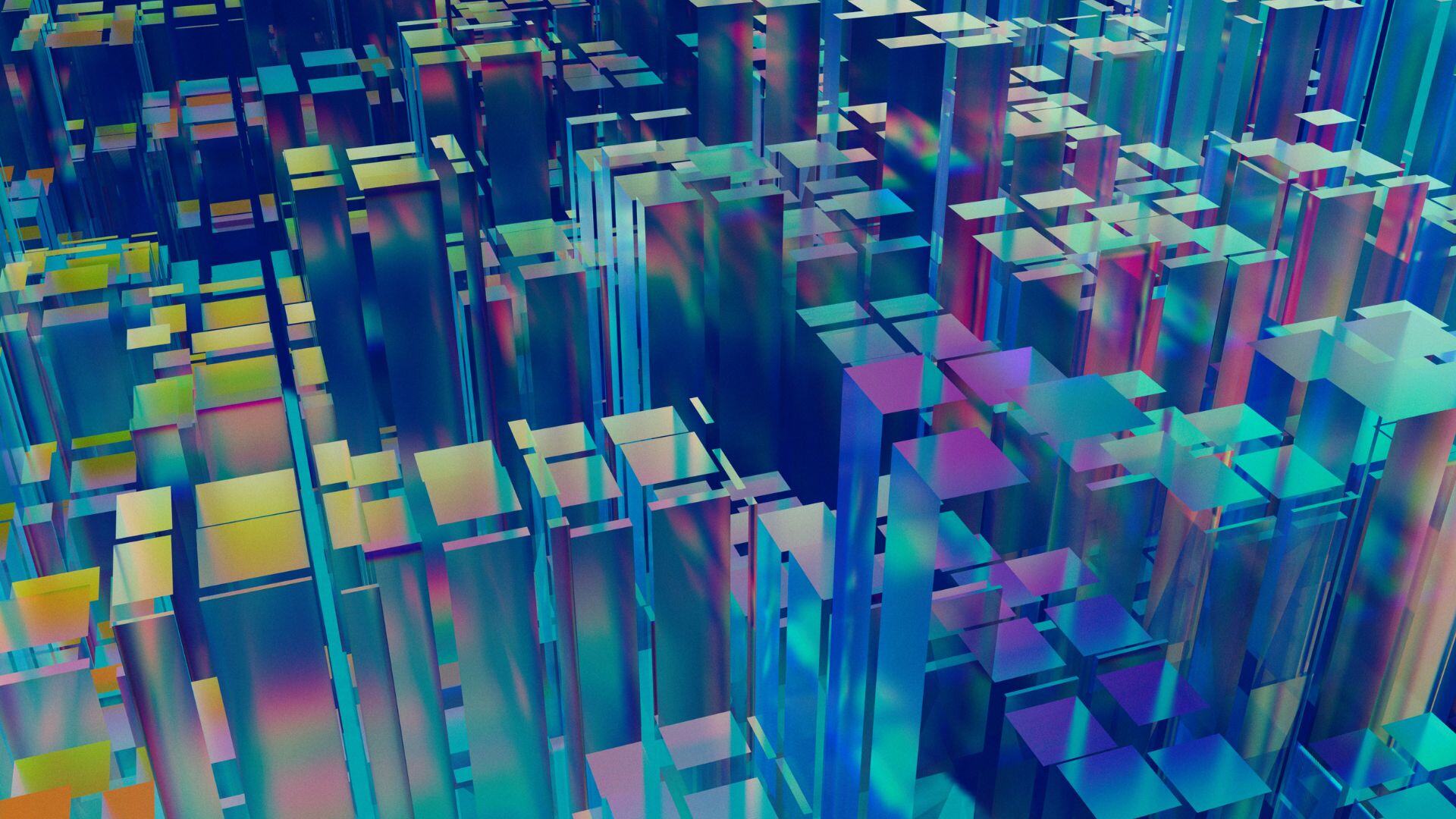
As the financial services industry harnesses the power of algorithms to refine credit decision-making, it faces a crucial challenge: ensuring fairness in customer outcomes.
In this interview with Gareth Hagger-Johnson, who has expertise in research methods to identify inequalities and algorithmic bias, we explore the implications of unintended bias. Gareth is Director of Data Strategy at the Nottingham Building Society and an Affiliated Academic at University College London (UCL), speaking in a personal capacity.
These biases, deeply embedded within the data and processes used to determine creditworthiness, risk perpetuating inequalities under the guise of neutrality. As we navigate the complexities of data-driven decision making, understanding and addressing these biases not only fulfils ethical mandates but also enhances the integrity of financial assessments.
Register now for CDAO UK in February to hear from Gareth about whether data quality is still the “elephant in the room” for data teams in 2025
Q: In your experience within the financial services sector, how do you address the challenges of ensuring fair customer outcomes, particularly in relation to algorithmic biases in credit decision-making, and what proactive measures are being taken to mitigate these biases?
Gareth Hagger-Johnson: A significant social challenge we face is ensuring fair customer outcomes. A major issue is algorithmic bias in credit decision-making. For instance, determining if someone is creditworthy for a loan can inadvertently bias against certain groups, even without including explicit demographic factors in the decision algorithm.
We’re seeing a trend toward more proactive approaches in other industries, including retrospective equality audits to identify inequalities in experiences for minority groups, in public sector settings. Prospective methods are also being developed to test for unintended biases before decisions are made. Though the term "discriminated" might seem strong, it captures the essence of introducing bias unintentionally.
We are at the very early stages of addressing these issues, but the conversation is advancing, particularly in the U.S. These cases highlight the need for better use of data to address such concerns. A recent UK review concluded that there is no intentional bias in credit decisions, as factors like ethnicity or gender are not used. However, that doesn't go far enough, in my opinion. Unintentional biases that emerge from how data is processed still pose a problem, as seen in my research with colleagues at UCL with hospital data linkage. The challenge is that the algorithms may not be sensitive enough to the nuances affecting various groups.
Such biases are not about explicit prejudice or discrimination; there's rarely any intentional desire to treat customers or service users differently. However, the biases inadvertently creep in through the algorithms, often driven by the data and correlations between where people live and their identities.
Q: In your view, do these biases predominantly stem from the data itself, the algorithms, or are our cultural assumptions being projected onto these tools?
Gareth Hagger-Johnson: Traditionally, mortgage was tailored to someone with a permanent job and regular income. Not everyone makes their money in this way today. In my opinion organizations need to be more aware of the potential for bias in data, before making decisions that impact customers or service users.
We've also seen geographical scoring used by lenders, where your credit score might partially reflect your neighborhood’s overall creditworthiness—this isn't intentional bias but an attempt to gauge broader lending risks, whether related to the property or the person. While lenders aren't targeting specific groups, socioeconomic factors correlated with social groups inevitably introduce biases.
Moreover, bias can manifest at various stages, not just in data correlations but also during manual review processes. Studies from both the U.S. and here have shown that discrepancies in documentation, such as a surname appearing differently across documents, can lead to declines or delays, impacting crucial timelines like home purchases. This can occur due to structural data correlations, algorithmic decisions, or at the ID verification stage, whether automated or manual.
So, while there's no intentional discrimination by individuals, biases can inadvertently arise at multiple points in the process. Looking ahead, I anticipate more discussions and trends focusing on these issues in the coming year.
Q: Shifting to a focus on data and analytics trends, you've indicated an expectation of more customer-focused analytics, particularly in using data to anticipate vulnerabilities and support customers who need additional help. Could you elaborate on what specific vulnerabilities you're referring to and how these individuals could be supported?
Gareth Hagger-Johnson: In many industries, and particularly in regulated sectors like financial services, we are required to pay close attention to vulnerable customers. Vulnerabilities might include factors like older age, which is not necessarily a vulnerability in itself but is a risk factor. Health conditions such as dementia, autism, and various forms of addiction are also considered vulnerabilities. These conditions are quite sensitive, and we must carefully manage how we support these customers because their needs are specific and unique.
Looking beyond financial services, I anticipate that sectors like grocery and retail might also begin to adopt more customer-focused, data-driven approaches. These sectors will need to account for customers who are not tech-savvy and those who prefer direct human interaction over digital solutions, like AI chatbots.
With the aging population and a segment of individuals living longer but in poorer health, including declines in literacy and memory skills, there's a growing need to use data not just for automation or digital transitions but to enhance support where it matters. Proper application of AI should safeguard these 'moments that matter' with a human touch, ensuring that traditional customer service values are not lost in the push towards digital transformation. We're not quite ready to forego face-to-face, person-to-person interactions entirely.
Q: Finally, your talk at the upcoming CDAO UK event asks if data quality is still “the elephant in the room.” Could you elaborate on why you chose to ask this?
Gareth Hagger-Johnson: The issue of data quality is often acknowledged but not adequately addressed. I wanted to examine whether this is still the case and explore how analytics has evolved, including the use of AI to assess where data quality is strong and where it isn't, allowing for targeted actions with different types of data.
Historically, there was a belief that all data needed to be completely cleaned before it could be useful, but I think we've moved past that. It's now recognized that analytics can still be effective with suboptimal data quality, and large companies have started investing in data quality teams. However, many smaller organizations may hesitate due to cost or because they perceive it as less exciting.
There's also a 'decay' in data quality, especially with marketing data. For customers who engage regularly and update their preferences, the data tends to be reliable. But what about those who have been inactive for many years? Should you contact them again to confirm their details? It's a common dilemma.
In my talk, I discuss strategies to handle these challenges and introduce methodologies from scientific and academic literature for modeling data quality issues, such as segmenting customers based on data quality—something not commonly practiced.
The key is to obtain high-quality metadata about your data quality. When I join an organization, one of the first things I recommend is to create a dashboard to assess the quality of the data, treating it as an asset. If it were gold, you’d want to know its purity and also any security arrangements for keeping it safe. It's the same for data.
While sessions on data monetization are typically packed, those on data quality are less attended, yet understanding and improving data quality is crucial for leveraging data as a revenue-generating asset. It's a fundamental step that ultimately pays dividends.
_____
Click here to register now for CDAO UK to hear directly from Gareth and other industry leaders about the future of data-driven strategy across industries.