Building Agile and Flexible Data Environments in Three Steps
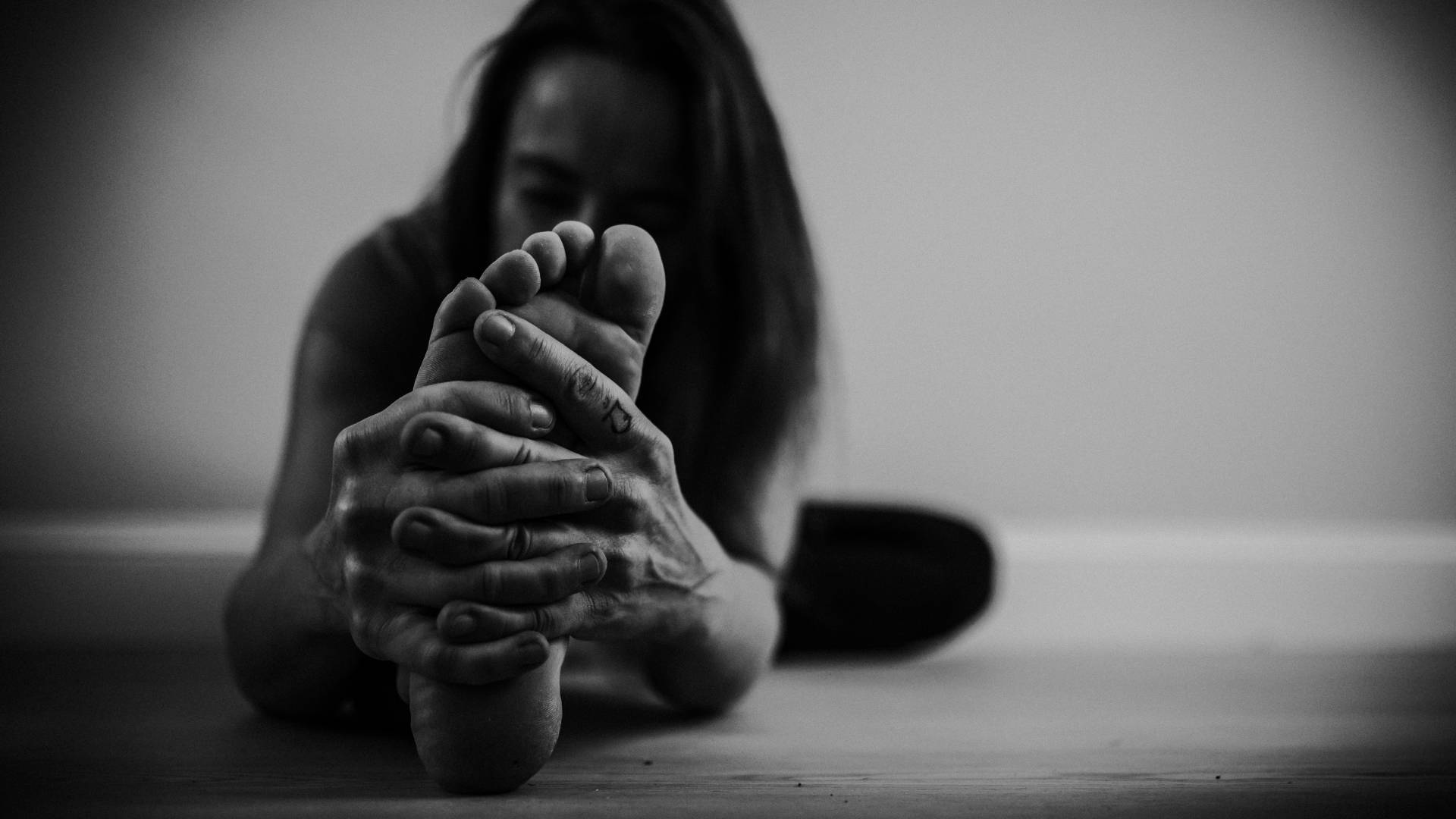
‘Agile thinking’, building collaborative, domain-centric teams and adopting a data mesh architecture all help data teams meet business needs more efficiently, according to panelists at CDAO Europe 2021
Business agility is key in today’s business environment, and analytics and data teams have a key role to play in supporting that agility. But without the right processes and structures in place, these teams will struggle to partner with company stakeholders to achieve business goals, executives speaking at Corinium’s CDAO Europe 2021 summit pointed out.
“Analytics and IT should not remain as support functions,” argued Jamill Del Rosario, Global Head, Analytics and Master Data at building materials manufacturer Holcim. “They should really partner with the business [and] have a common objective, guided by business cases.”
“Such a partnership is what will truly transform analytics into a relevant and strategic business enabler,” he added.
In a panel discussion on the topic, speakers identified three steps data-focused executives should explore to help their teams support business agility more effectively.
Step 1: Adopting an Agile Mindset
For many, Agile has overtaken its predecessor, Waterfall, as the data product development methodology of choice in recent years.
Speakers at CDAO Europe 2021 were keen to point out that having defined processes like these to ensure efficiency and alignment with business needs when developing new data products can be very helpful.
“Having an Agile approach to some extent helps to build some kind of framework on how we are working,” said Óli Páll Geirsson, former Chief Data Officer for The City of Reykjavik. “The main thing here for agility is to have a framework that your team has buy-in for. That’s the most important part. They need to see the benefits of working in this framework.”
“If you belong to a huge company where new people come and go in the team, obviously having a framework that sustains that way of working would be beneficial for the operations and for the sustainability of the analytics team,” Del Rosario added.
However, the panelists broadly agreed that instilling the right mindset in staff is more important than making them study for Agile certifications.
“The most important thing is about mindset,” concluded Roberto Cavicchioli, Data Analytics Office Manager at food packaging and processing company Tetra Pak. “It’s about putting the final user at the center and continuously looping back and understanding if we are going in the right direction.”
Step 2: Building a Flexible Data Environment
In addition to having efficient working practices, data and analytics teams need the right data infrastructure in place to support business agility.
Andrea Proserpio, Head of Analytics at financial services company Gruppo MOL, said: “Once the machine is working, finding the right tools to move [as little] data as possible and stuff like that is of course helping the speed to market for initiatives.”
Charles Southwood, Regional VP, Northern Europe and MEA at data virtualization specialist Denodo Technologies agreed that data architecture also has a role to play in facilitating business agility.
He argued that the key is to use a virtual environment to enable staff (even those without specialist data engineering skills) to access many data sources without needing to copy everything into a central data store.
“The way we seem to be helping organizations most is by providing a common layer where you don’t move and copy that data,” Southwood explained. “The federated model of the shape using data virtualization has most recently been coined as a ‘data mesh’.”
He added: “The technology to be able to do this has been around [for a while]. But concept of a data mesh is a relatively new one and is based on a centralized infrastructure where, instead of being managed by a single team, the data is distributed across different domain-specific teams.”
Step 3: Cultivating Cross-Functional Collaboration
One of the key benefits of agile thinking and flexible data architectures is that they support and encourage collaboration between data-focused teams and the wider business.
But building cross-functional teams and developing tools that allow non-technical stakeholders to self-serve certain insights is not sufficient to cultivate the conditional for analytics success by itself. Data governance, data literacy and business fluency also have roles to play.
Del Rosario noted that data team challenges arise when analytics, IT and business operations teams don’t understand each other.
“The analytics and IT teams should speak the business language and should understand the business contexts and the pain points that they’re trying to address,” he said. “Vice versa, the business should also try to understand how analytics and IT could enable the business and [have] some basic understanding of the function.”
“You [need] common definitions, which are the models that are consistent across the whole business,” Southwood added. “Elements like ‘customer’ and ‘product’, where you have an agreement on the semantics of all the common entities, so you get the same answer to the same question.”
Overcoming these challenges often requires learning and development investment across the enterprise. But once data, business and IT stakeholders understand each other, have access to self-service data environments and have processes for working together efficiently, the results can be transformative.
“If it’s deployed properly, it can be very effective,” Southwood concluded. “We’ve seen organizations that have literally transformed their business by deploying this.”
This article contains reporting from a panel discussion at CDAO Europe 2021. To access this and all the other sessions from the event on-demand, click here now.