Agile Innovation: How Data Leaders are Solving their Productivity Problem
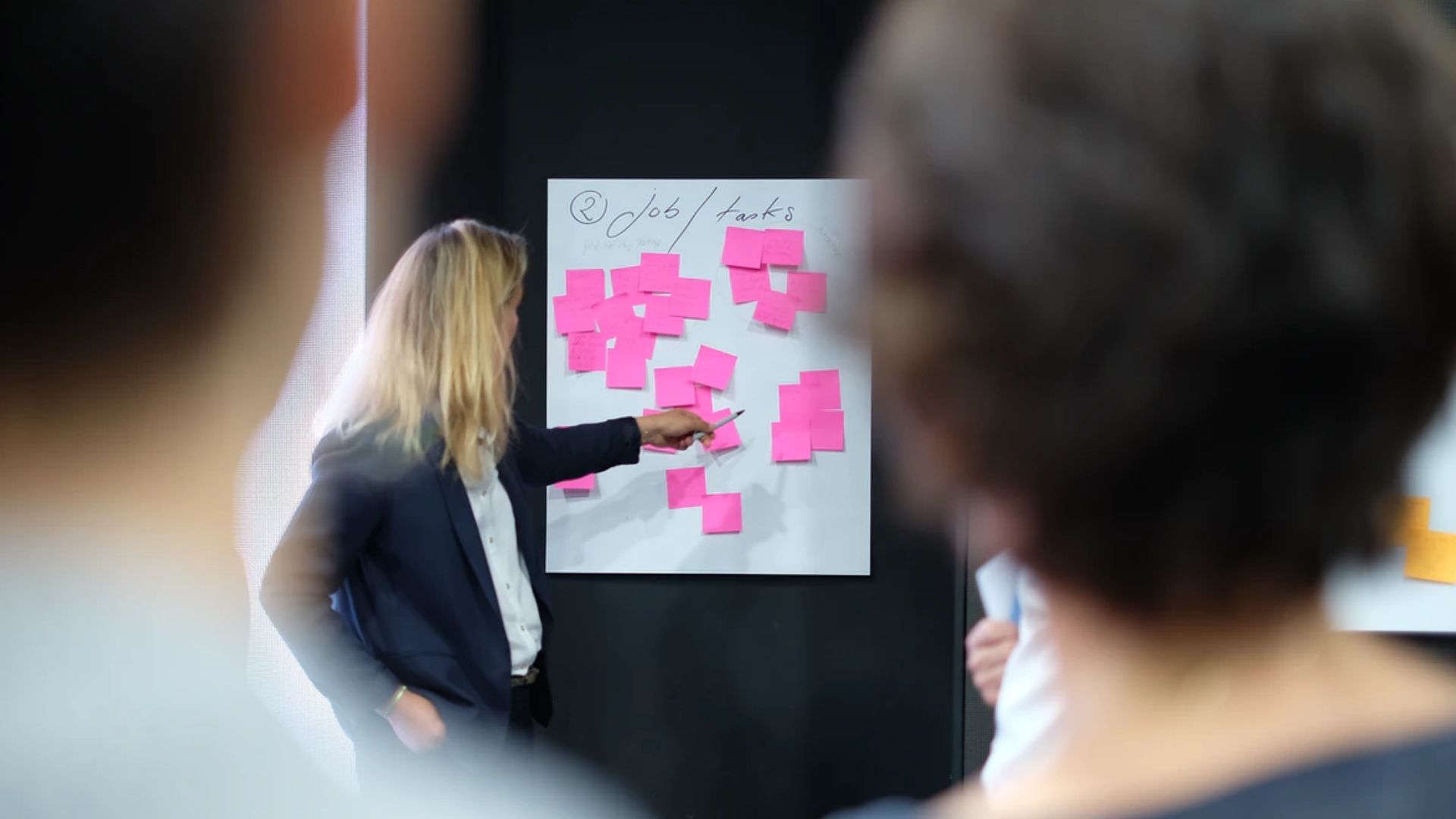
CDAO Fall 2020 showcased the Agile and collaborative techniques enterprises are using to unleash the full potential of their data and analytics teams
Data and analytics has a productivity problem. Despite the wealth of data and technology at their fingertips, many teams drive less value than they should for their organizations because inefficient processes are holding them back.
As the industry experts who spoke at Corinium’s 2020 CDAO Fall conference last week note, there are many factors that can create challenges for data and analytics team productivity.
They may find it hard to align their efforts with business needs. They may struggle to adapt to changing business requirements. They may burn themselves out fighting fires or making heroic efforts to meet deadlines. They may even struggle with clunky, outdated data infrastructure.
“[COVID-19 has increased] the need for data governance, data sharing and analytic methods including AI and machine learning,” says JoAnn Stonier, CDO at Mastercard. “Everything is in greater demand and together, as data professionals, we really need to come up with new solutions.”
To help data-focused executives do exactly that, we’ve pulled together the key insights around boosting team productivity that were discussed at this three-day virtual event.
Why it’s Important to Cultivate Agile Mindsets
Delivering analytics or AI projects requires collaboration between staff in many different roles. As Goldman Sachs Director of Advanced Analytics El Diawlol notes, a lack of alignment between any of these stakeholders can have a significant impact on team productivity.
“What type of skills will you need within your team?” he asks. “We talk about technology. We talk about core computer science and programming skills. But also, understand that you need soft skills. You need people who can visualize your data. You need people who can articulate the findings of your analytics effort to the business.”
Building multidisciplinary teams to deliver data and analytics projects helps to ensure the people who will use a company’s data-driven insights or tools are involved in the development process. It also avoids potential bottlenecks and delays that occur when projects are passed between teams.
But as Dominic Fortin, AVP of BI and Data Strategy Solutions at insurance firm The Co-Operators, notes, staff on these teams mustn’t be solely focused on the tasks they’re explicitly responsible for. Effective collaboration means everyone taking joint responsibility for a project’s success.
“It’s no longer, ‘The project screwed up, but I did not,’” he says. “It’s, we succeed together, or we fail together.”
Reframing data or analytics projects as ‘products’ that are delivered to business ‘customers’ can help, here. Teams must understand that delivering things that drive business results is their primary responsibility.
The ‘75% Principle’ for Iterative Improvement
It might be tempting to try and make a data or analytics product perfect before delivering it to the end-user. But it’s often better to deliver some value quickly and to perfect things later.
James Royster, Head of the Global Commercial Operations Transition Team at pharmaceutical company Celgene, calls this the ‘75% principle’. Giving users access to three quarters of the insights or functionality they originally asked for creates an opportunity for them to provide feedback.
“We learned through that process that very often, even though the customer very clearly gives you a direction of what they want, they don’t always really know what they want,” he says. “Giving them that iterative process helps them formulate what they want.”
This approach helps to nurture the culture of constant communication and collaboration that’s needed to ensure data and analytics professionals are well-aligned with the needs of the business units they serve.
It also fits neatly with the Agile mantra of delivering value regularly. Splitting large projects into smaller chunks that can be delivered in 12 weeks or less helps data and analytics teams keep their colleagues engaged with the development process by regularly providing them with new capabilities.
Working in this way also makes it easier for data leaders to regularly reprioritize staff workloads to reflect changing business needs. Involving business stakeholders in this process is another great way to maintain alignment and set expectations about what will be delivered when.
Developing a Data Infrastructure That Enables Agile
For Josh Brickman, VP Business Strategy at Boston sports arena TD Gardens, overcoming the ‘silo mindsets’ that are entrenched in many enterprises is essential for enabling data and analytics value creation. Achieving this goal depends on there being the right data infrastructure in place to facilitate Agile data practices.
“Data has lived in siloed systems for such a long time that people are just used to going to multiple places to get the information they need,” says Brickman. “Understanding how to be able to bring that information together is obviously tantamount to our success.”
At the same time, data leaders should build the right data management and governance steps into their data team processes. Automated quality assurance tests can be an efficient way to safeguard data quality and avoid lengthy ‘finger pointing’ exercises when issues are spotted late in the development process.
“You can iterate yourself [towards] getting it right,” says Christopher Bergh, CEO and Founder of DataOps platform DataKitchen. “Having any customer master is better than not. Having any data dictionary is better than not. And then iterating and improving it over time can also help.”
Building an efficient data or analytics team is an evolution. It takes practice, iteration and continuous improvement. But through applying the techniques we’ve looked at here, data and analytics leaders can empower their teams to drive greater business results.
Royster concludes: “Over time, what you do is you take those problems away and you start to allow people to fulfil some of those things that I think most analysts would like to fulfil, which is being able to deliver something quickly or being able to do something creatively.”
Catch up on all the talks from CDAO Fall 2020 and discover how America's top data leaders are making their teams more efficient by registering to view the on-demand sessions right here.