Combating AI Model Bias in 2021
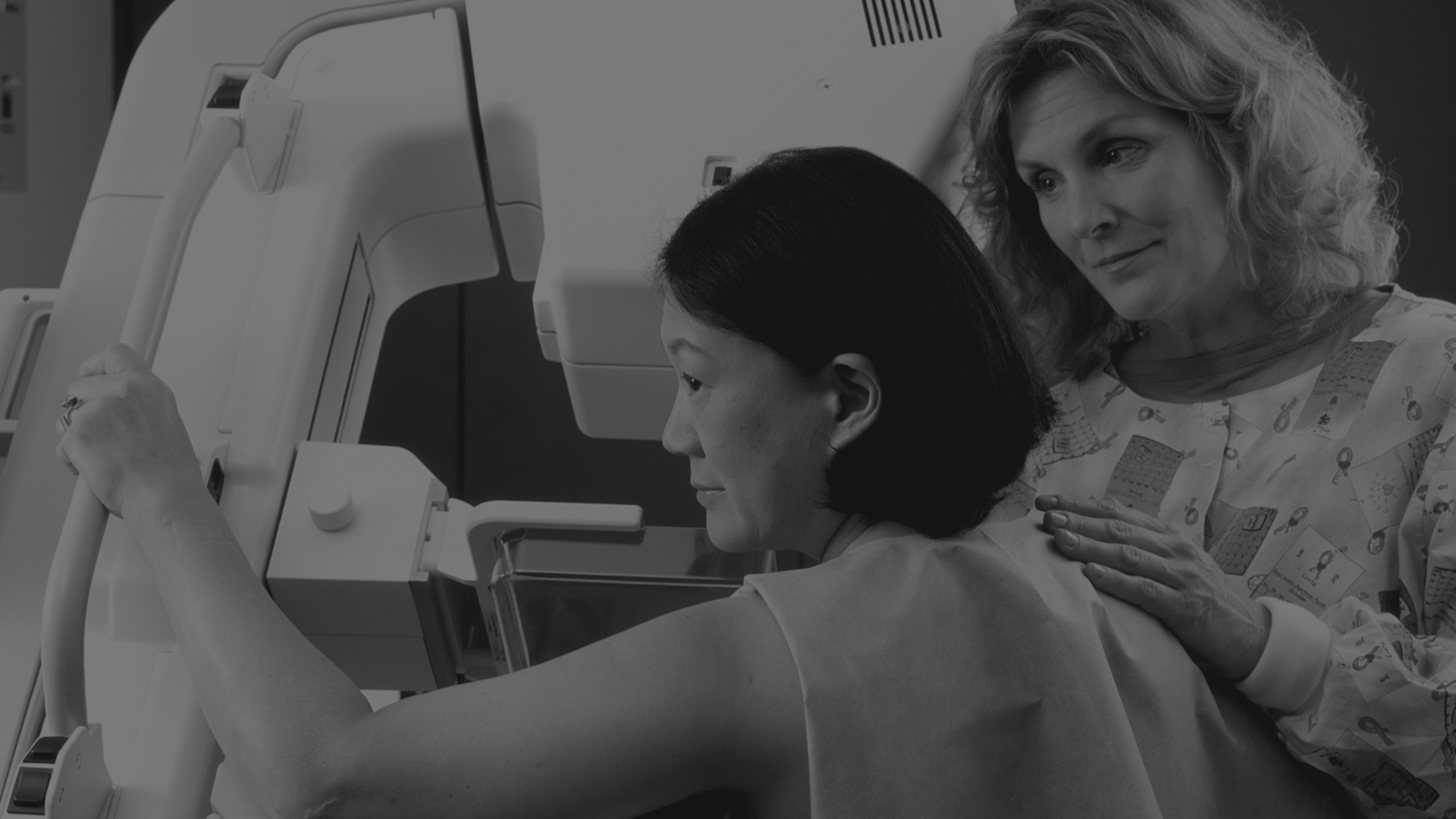
Combating AI model bias is an essential for ensuring the technology is used responsibly, but many enterprises don’t yet have the right measures in place to do this effectively
“Most of recorded human history is one big data gap,” writes Caroline Criado Perez in Invisible Women, her renowned book on data bias. “These silences, these gaps, have consequences.”
In this case, Perez is talking about the impact the underrepresentation of women in medical research data has had on the effectiveness of medical treatments on women. But there are many other ways bias can infect business processes or decisioning, especially in the realm of AI.
Our research provides evidence that enterprises are bringing processes to combat AI model bias ‘in house’. Just 10% of the 100 AI-focused executives we surveyed now rely on a third-party evaluate models for them.
However, the effectiveness of processes designed to combat bias vary from company to company. For example, while 73% say they have effective processes for ensuring the data that feeds models is well-governed and unbiased, the remaining 27% say they do not.
“We see a lot of the bad behaviors that are going into artificial intelligence are really coming from bad data behaviors,” says AI Truth Founder and CEO Cortnie Abercrombie. “I've always said at 90% of what's going wrong in the AI world is related to the data itself and the way we use that data.”
Our research has uncovered three focus areas that can help enterprises de-risk AI innovation and combat all kinds of bias in AI models.
Practicing AI Ethics and Risk Management by Design
For Jordan Levine, MIT Lecturer and Partner at AI training provider Dynamic Ideas, the reputational risks that come with irresponsible AI use stem primarily from undetected instances of bias. As such, he argues that enterprises need rigorous processes to find, spot and remove bias across the model lifecycle.
He explains: “The problem statement is, by building a model, do I then have subsets of my population that have a different accuracy rate than the broader, global population when they get fed into the model?”
Our research shows that enterprises are using a range of approaches to root out causes of AI bias during the model development process. However, it also suggests that few organizations have a comprehensive suite of checks and balances in place.
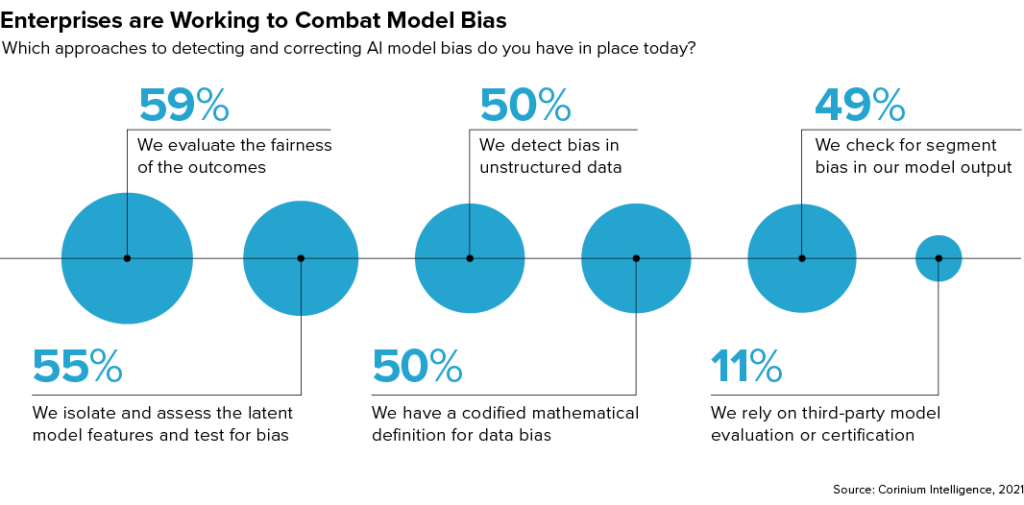
Evaluating the fairness of model outcomes is the most popular safeguard in the business community today, with 59% of respondents saying they do this to detect model bias. Half say they have a codified mathematical definition for data bias and actively check for bias in unstructured data sources. Meanwhile, 55% say they isolate and assess latent model features for bias.
Just 22% of respondents say their enterprise has an AI ethics board to consider questions on AI ethics and fairness. One in three have a model validation team to assess newly developed models and only 38% say they have data bias mitigation steps built into model development processes.
In fact, securing the resources to ensure AI models are developed responsibly remains an issue for many. Just 54% of respondents say they are able to do this relatively easily, while 46% say it’s a challenge.
As such, it looks like few enterprises have the kind of ‘ethics by design’ approach in place that would ensure they routinely test for and correct AI bias issues during the development processes.
Educating Business Stakeholders about Responsible AI
Some business stakeholders may view considerations around AI ethics as a drag on the pace of innovation. In fact, 62% of our survey respondents say they find it challenging to balance the need to be responsible with the need to bring new innovations to market quickly in their organizations.
This challenge goes all the way to the top of the modern enterprise. A full 78% of respondents say they’re finding it challenging to secure executive support for prioritizing AI ethics and responsible AI practices.
This may explain why so few businesses have everything they need in place to ensure the models they have in production are used responsibly.
“AI vendors really need to insist on going through the process of vetting AI models with the right people at the table”
Cortnie Abercrombie, Founder and CEO, AI Truth
“Always start with a business problem first,” Levine recommends. “Then, take serious effort to translate it into an analytics problem statement. Think through the data you need, the models you will generate, the decisions the models will influence and the value that will drive.”
From there, Levine says analytics leaders must give their teams protocols that bring business stakeholders into the process, to show business stakeholders that they understand the data and lean on their subject matter expertise.
He also suggests running through the finished model with business stakeholders to ensure they understand how it arrives at its decisions and its true accuracy, so they can make rational decisions based on its outputs.
Only then should enterprises run a small-scale pilot, measure the results the model generates and consider scaling it across the organization.
Cutting corners at any of these junctures can expose enterprises to risk. So, it’s up to AI-focused executives to educate their colleagues about those risks and ensure everyone understands how the AI systems they use work.
Renewing the Focus on Explainable AI
Explainability is a key indicator of responsible AI use. If a company can’t explain how an algorithm makes decisions about its customers, they can’t be certain that the model is treating all customers fairly.
So, it’s surprising that this year’s research implies a shift in business priorities away from explainability and toward model accuracy. This could be because securing buy-in for AI projects is becoming easier or due to increased awareness that model bias often manifests as inaccurate outputs for certain population segments. Either way, it’s important that the role explainability plays in ensuring AI is used responsibly is not forgotten.
“Companies must be able to explain to people why whatever resource was denied to them by an AI was denied,” says Abercrombie.
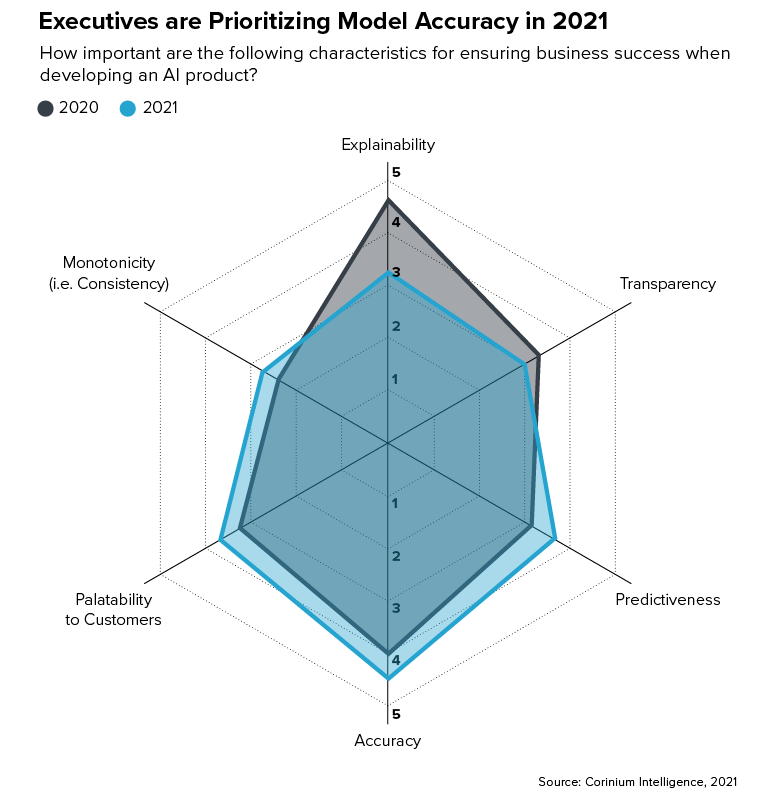
Again, our research shows that many businesses have a way to go, here. While 51% do have processes in place to ensure their teams can explain how AI-based decisions or predictions are made in general, just 35% can explain how specific decisions of predictions are made.
There are two ways to address this challenge. AI-focused leaders can either select AI model types that are inherently explainable or use ‘bolt-on’ tools that help to explain the results more complex models generate.
“I recommend using optimal classification trees,” says Levine. “While the algorithm might lose accuracy, the benefits in human interpretation generally, in my mind, outweigh the delta.”
He adds: “In my view, every case requires an analytics team or a board or a set of researchers and analysts to evaluate both a tree-based method and the best black box method they can find.”
This article is an excerpt from our The State of Responsible AI: 2021 research report. To discover more about how C-level executives are combatting AI model bias and ensuring AI is used responsibly in 2021, click here now to access the full report.