Key DataOps Challenges Enterprises Must Overcome
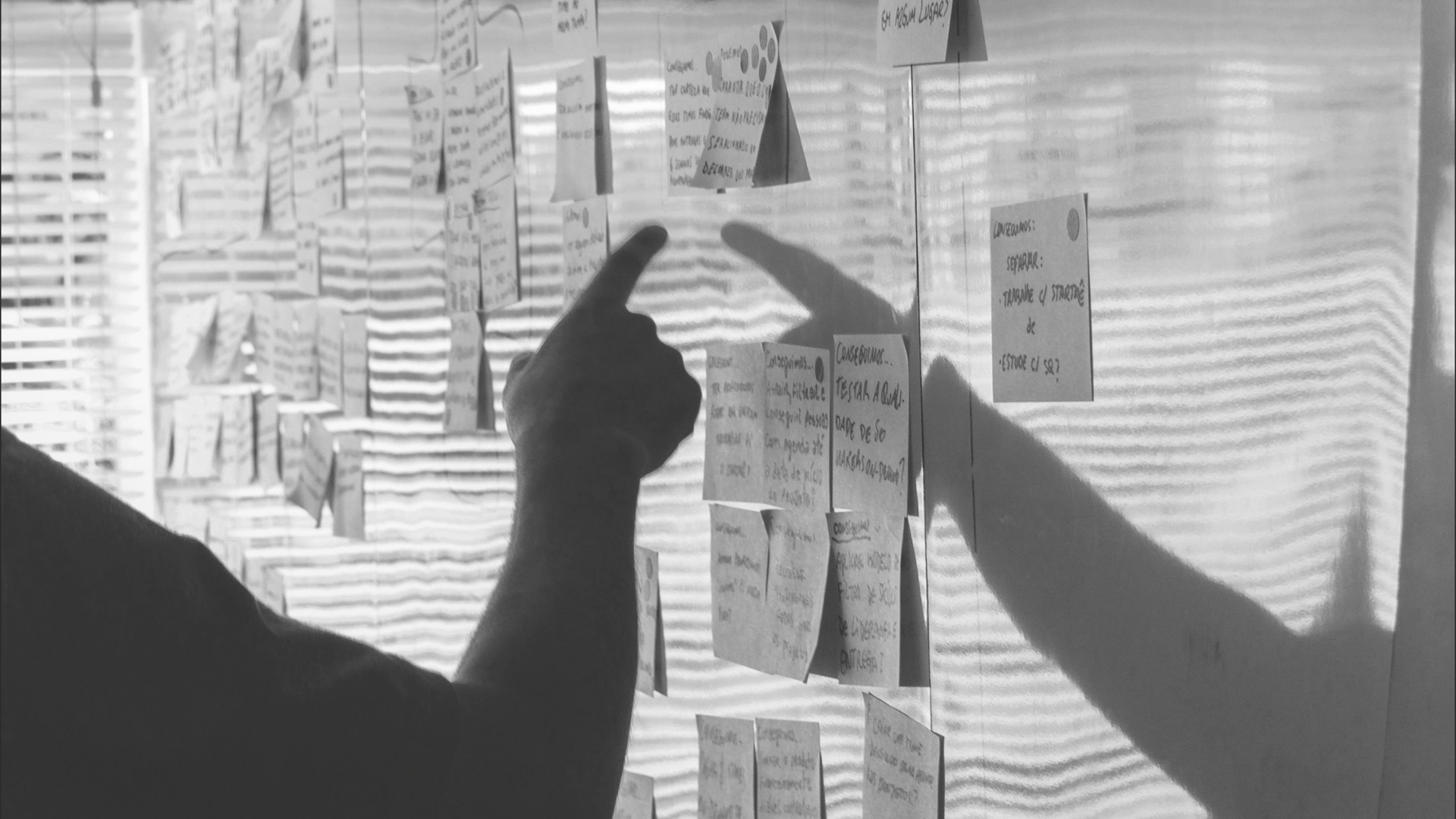
Enterprises must overcome a range of technical and human challenges to realize the productivity gains associated with DataOps adoption
Adopting DataOps requires a combination of technical investment, organizational restructuring and change management. It requires people to fundamentally change the way they work, and this change isn’t going to happen overnight.
Our research shows that the barriers to DataOps adoption in enterprises today can be divided into two types: technical challenges and human ones.
In both cases, many DataOps practitioners recommend changing things bit by bit. Just as enterprises can benefit from deploying a minimum viable product (MVP) and improving it over time, enterprise data teams can benefit from adopting DataOps best practices in a series of incremental steps.
“[It’s about] helping people understand that you’re not going to get the Cadillac at the beginning,” says David Wright, Chief Data Officer, Commerzbank AG. “This is an iterative process. We want to deploy something. We want to learn from you. We want to tack onto it.”
Technical Barriers to DataOps Maturity
Technical infrastructure and tooling is are essential to DataOps success. Teams need platforms that help break down data silos, observe data pipelines, orchestrate standardized governance practices and enable data management process automation.
However, companies appear to be struggling put effective systems in place for these purposes. Our survey of 100 European data and analytics leaders shows that teams are facing many technical challenges as they start out on their DataOps journeys.
“Large enterprises exist in a place where they have whatever data systems they have had in place for years,” notes Guy Taylor, Interim Director of Experimentation at Booking.com. “There seems to be, across organizations, an operational gap when it comes to resolving data quality issues.”
“I think monitoring is an exceptionally useful thing to be doing with your data, and creating that observability is best practice”
Guy Taylor, Interim Director of Experimentation, Booking.com
‘Difficulty orchestrating code and data across tools’ and ‘difficulty monitoring the end-to-end data environment’ are the top technical DataOps challenges facing enterprise data teams today. These were both cited by 44% of our respondents.
At the same time, 31% say insufficient levels of investment in DataOps tools is hindering their DataOps initiatives. Meanwhile, 22% cite challenges rooted in a lack of automation tools and 18% say it’s proving challenging to build rigorous quality assurance tests upfront.
“I think monitoring is an exceptionally useful thing to be doing with your data, and creating that observability is best practice,” Taylor says.
“Setting up contracts between your producers and your consumers is good best practice,” he adds. “Understanding what kind of data you are expecting to land is good practice. Then, you can even do some anomaly detection across that stuff.”
Human Barriers to DataOps Maturity
While technical challenges can be difficult to overcome, some of the human barriers executives report around their DataOps initiatives are even more pressing. Changing culture, adopting new ways of working and bringing stakeholders on the journey are key.
Minna Kärhä, former Data and Analytics Lead at Finnair, highlights the people challenge as central to DataOps adoption.
She says: “If the DataOps team has people in it that are not experienced with this DataOps mentality and their background is more on the traditional side of data management, it's a big learning curve for them to forget the principles and practices they are used to.”
“Everybody needs to work together. It's not something that each team is just focusing on their own tasks. It's really a collaboration”
Minna Kärhä, Former Data and Analytics Lead, Finnair
Ciaran Dynes, Chief Product Officer at Matillion, agrees: “The ETL engineers of yesteryear weren't involved in automation to that extreme. They weren't looking at these thousands of different data sources.”
Our survey results support this view, with 48% of respondents saying their staff lack the time to learn new skills. Similarly, 34% report a lack of staff with the right DataOps skills and 35% say they’re struggling to establish formal processes to ensure consistent practices across the organization.
Meanwhile, 31% say it’s a struggle to get staff to adopt new practices and 22% are facing difficulties around adopting agile working practices.
For Kärhä, overcoming these challenges is essential to DataOps adoption. Building environments that foster collaboration between data producers, data consumers and the DataOps team is key. Staff at each stage of the data pipeline must understand the role in and responsibilities around ensuring that data is high-quality and well-governed.
An Agile Approach to DataOps Adoption
Harvinder Atwal, Chief Data Science Officer at MoneySuperMarket, recommends that companies at the start of their DataOps journeys take an agile approach. Start with a single use case and map the end-to-end processes and timeline for getting from A to B. Then, focus on applying DataOps principles to make that process more efficient.
“The first time it's done it can be quite hard and quite a big change for a lot of teams,” he says. “They may not even be used to working together. They may only know their respective parts of the process.”
Once a team understands the basics, it can start looking for opportunities to make improvements. This starts with identifying bottlenecks in data access or cleaning, model development or deployment and delivery management processes, and exploring how they may be alleviated.
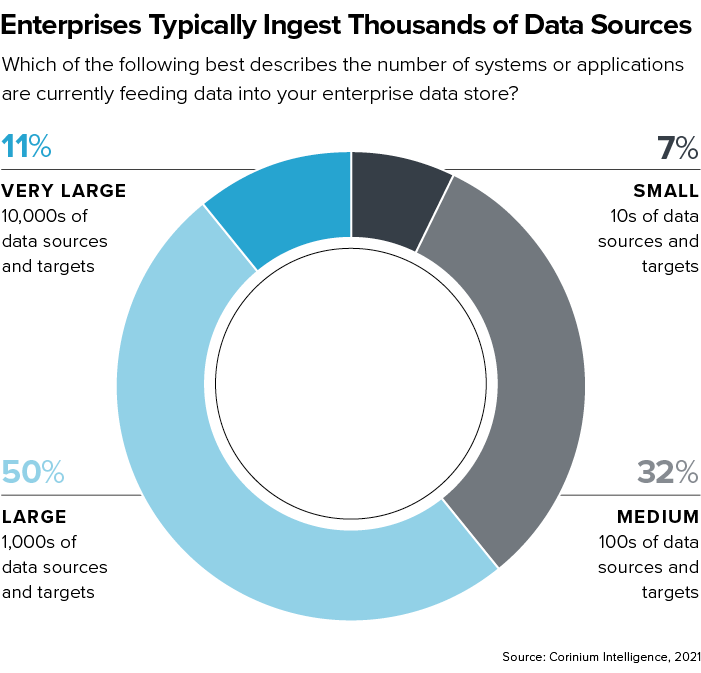
“It's always important to get very rapid feedback from your customers, but also on your processes themselves,” Atwal notes. “So, make sure there's the right telemetry and metrics to understand if you are improving.”
As Prabhu Chandrasekhar, Director, Data Foundation, Enterprise Data and Analytics at Advance Auto Parts, says, data teams generally support many business units with a range of data maturity levels.
“Everyone has different levels of engagement and maturity in the data science world,” he says. “At the end of the day, they’re all trying to meet the goals of their business units and we’ve all got to appreciate that fact.”
DataOps can help teams serve the rest of the business more effectively. But an iterative approach will generally be needed to overcome the technical and human barriers standing between them and DataOps maturity.
This is an excerpt from our The DataOps Agenda 2021 research report. To discover more about the maturity of DataOps practices in European enterprises today, click here now and claim your copy.