Who Should Be Responsible for ModelOps
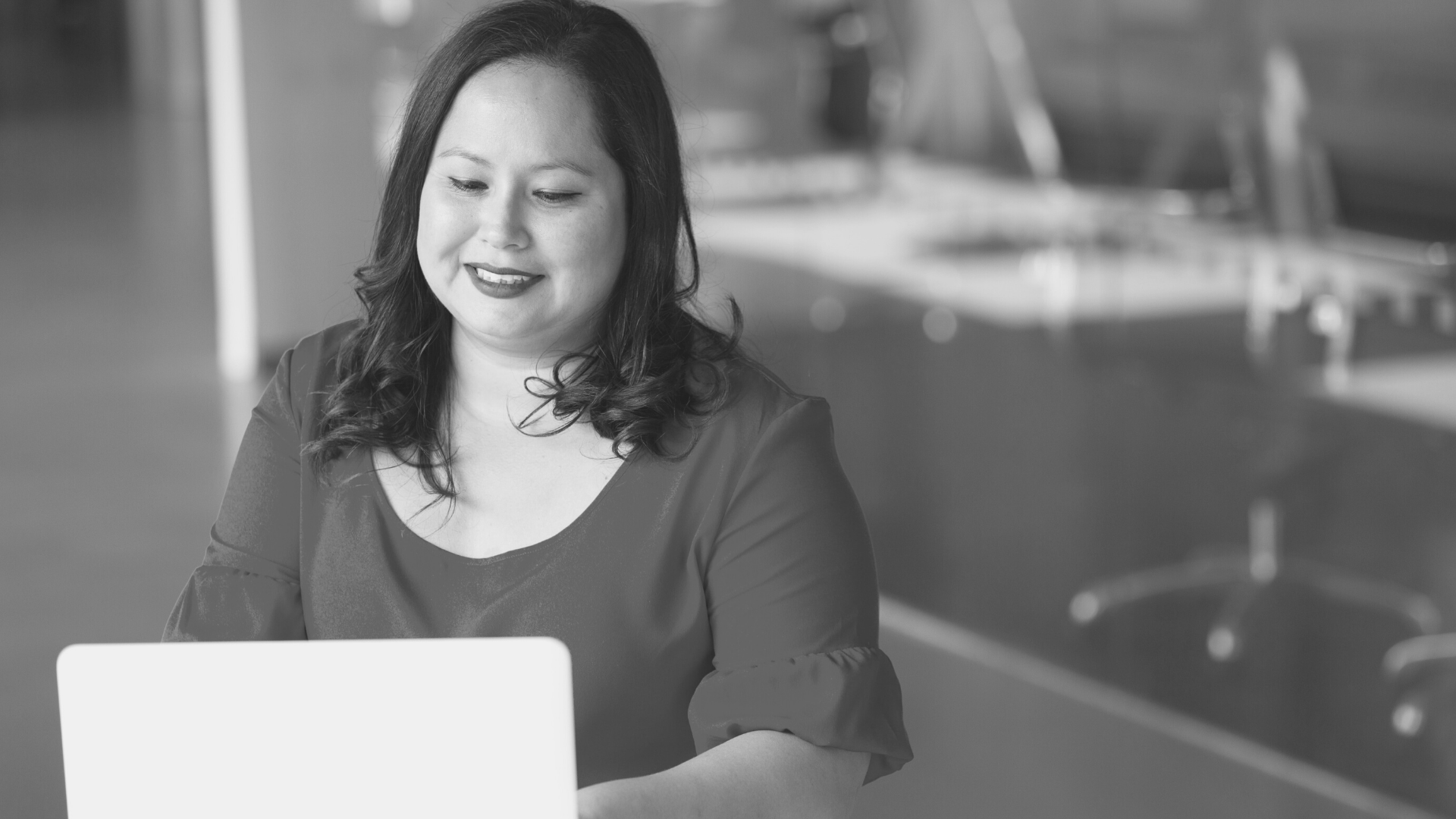
Enterprises are establishing dedicated roles, such as the ‘model operator’, to oversee the effective monitoring, governance and maintenance of AI models in production
The ModelOps function is underdeveloped in many enterprises because it has initially fallen to established organizations such as DevOps.
This arrangement is not ideal, due to factors including the unique characteristics of models compared with conventional software, the internal and external compliance and regulatory requirements around AI models and the need to support many stakeholders and teams.
Enterprises often lack a dedicated team with the knowledge, remit or authority to orchestrate ModelOps as a cross-functional capability. Some say that data science teams should be responsible, but others feel that this isn’t the way to go.
“You have to have this third-party that knows what they're looking at and knows how to measure it,” argues BNY Mellon Data Science Fellow Skip McCormick. “You never let the data scientists create their own paper because they're too close to the problem.”
“You should not have data scientists governing and maintaining their own models. In the past that was often true, and it was not effective”
Glenn Hofmann, Chief Analytics Officer, New York Life Insurance Company
There are many differing views as to where responsibility for ModelOps should live. Perhaps not surprisingly, respondents in IT functions are the least likely to say that they feel responsible for ModelOps. Executives in these roles believe data scientists, model operators or model engineers should have this responsibility.
However, a consensus may be emerging that people skilled in ITOps have a strong foundation for ModelOps and may be best positioned to become model operators, as they have deep experience keeping production systems running ‘round the clock’.
The Rise of the Model Operator
Until recently, DevOps professionals oversaw model operationalization in most enterprises. One 2019 Gartner study found that this was the case in 61% of organizations.
“That's why my group was introduced,” recalls Patricia Flynn, State Street’s VP for Information Governance and Solution Delivery. “I support five businesses within Global Markets, and I sit in the risk organization. I'm independent to the business lines, so I don't have the revenue concerns.”
But over the past 12-24 months, a new class of AI professional has emerged: the model operator.
Our research suggests that 60% of large financial services enterprises now have model operators or model engineers overseeing model operations across their businesses. By contrast, just 26% of the 100 executives we surveyed say their DevOps teams are responsible for ModelOps.
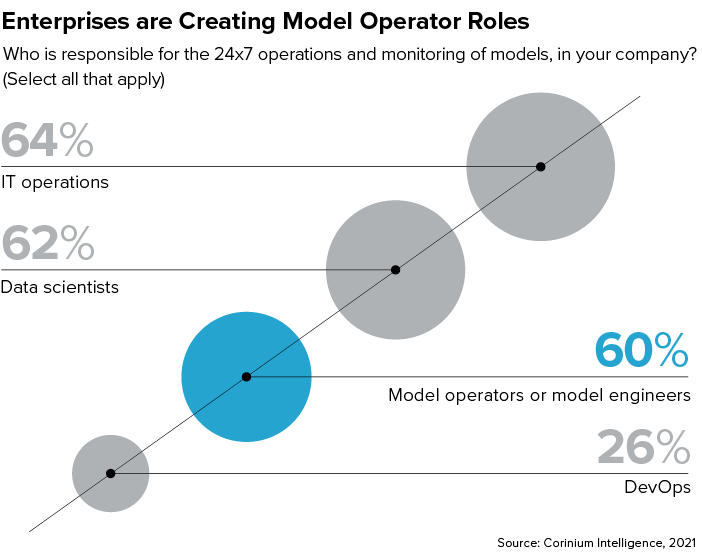
“From a monitoring perspective, we have a couple of dedicated people on my team,” says Glenn Hofmann, Chief Analytics Officer at New York Life Insurance Company. “All they do is post-deployment monitoring and reporting.”
The creation of this new class of AI professional reflects the growing consensus that managing AI models is different from managing traditional software or statistical models.
Yet, despite the fact that data scientists are generally not skilled (or interested) in running 24x7 production operations, 62% of respondents say their data scientists should have a role in ModelOps.
For McCormick, this indicates that many enterprises still have some maturing to do. In particular, he argues that having data scientists manage their own models creates a conflict of interests.
At the same time, having data scientists monitor and manage their own models is inefficient. Data scientists have unique expertise in model development and are expensive. So, it makes sense to ensure they can spend as much of their time as possible actively driving business value and innovating with new ideas.
“You should not have data scientists governing and maintaining their own models,” Hofmann agrees. “In the past that was often true, and it was not effective.”
Today, 40% of enterprises still lack dedicated model operators to handle day-to-day ModelOps tasks. But if current trends continue, this should be expected to decrease.
This is an excerpt from our State of ModelOps 2021 research. To discover more about this emerging and vital business function, click here now to access the full research.