Enterprise AI Maturity is Driving the Evolution of ModelOps
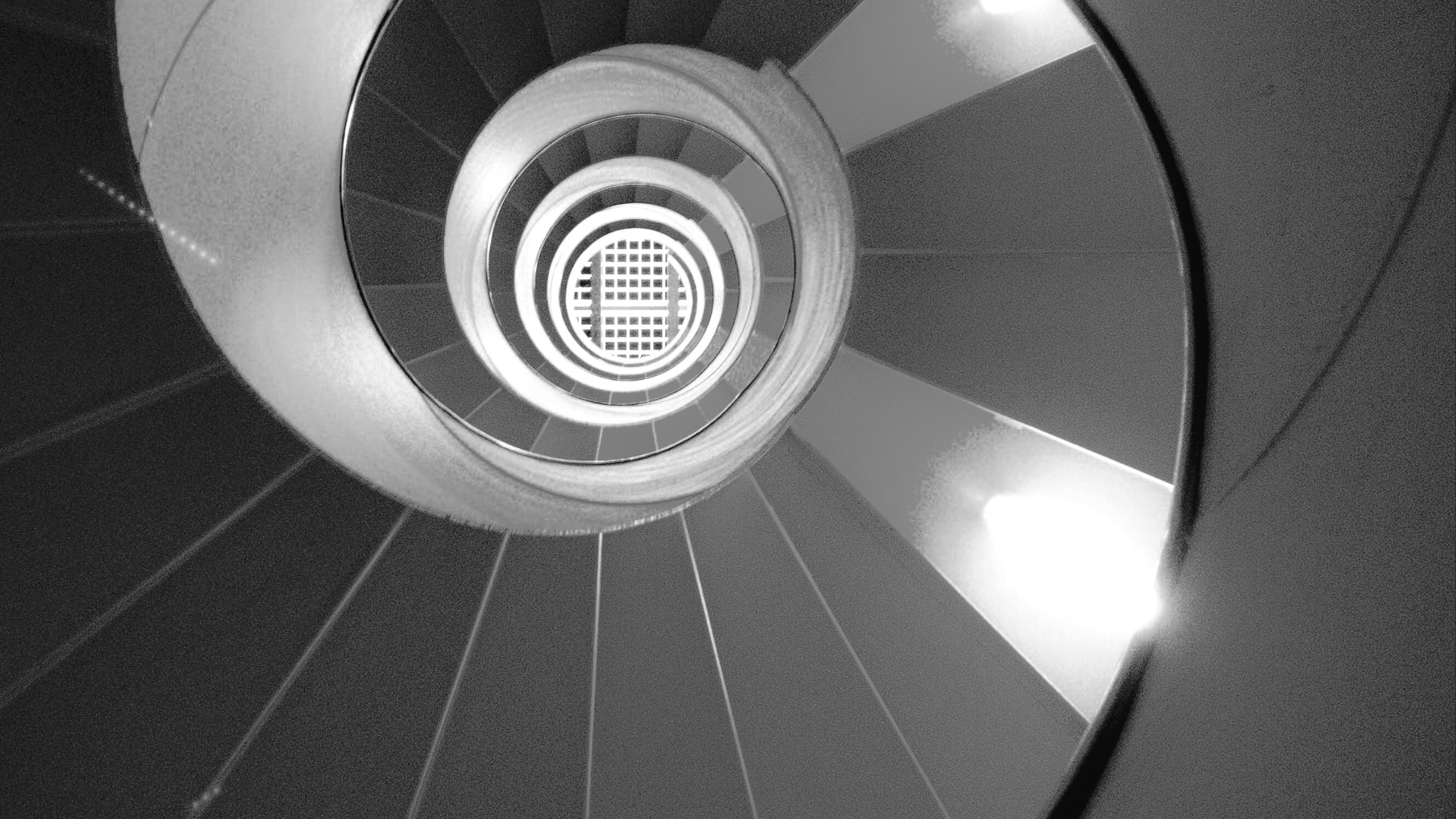
Enterprises know they must develop better practices for model operationalization and are investing accordingly. Meanwhile, those without mature ModelOps functions are struggling to get the most from their AI strategies
Global enterprises experienced a mass awakening to the need for better practices to manage and operationalize AI models in 2022, our second annual State of ModelOps survey reveals.
When we conducted our inaugural survey of 100 AI-focused executives last year, 49% of the executives we polled said their companies didn’t have a dedicated budget for model operations. This year, every single respondent to our research says their enterprises now have a budget for ModelOps.
Respondents were all selected from enterprises with annual revenues of at least $1.8 billion USD, meaning they work for the US and Europe’s largest companies. Our findings show that 52% of these companies have more than $500,000 USD allocated for ModelOps each year. What’s more, 12% have ModelOps budgets larger than $1 million USD.
“Awareness around the importance of ModelOps has definitely been growing,” reports Glenn Hofmann PhD, Chief Analytics Officer at life insurance company New York Life.
“Our ModelOps team has done quite a bit of work to govern and manage our models, adding value that has been recognized by others.”
These findings suggest a significant increase in awareness of the need for better enterprise ModelOps practices, which we define as the enterprise capability focused on governance and lifecycle management of AI-based decision models, as well as traditional statistical models. This covers everything that happens from moment a model is released into production until (and beyond) its ultimate retirement.
As one executive we interviewed last year noted, AI models cannot be managed and governed in the same way as conventional software. As such, AI adoption is putting strain on legacy enterprise business processes.
“For straight transactional processing, I think the model that we have works very well,” State Street VP of Information Governance and Solution Delivery Patricia Flynn said. “For machine learning and AI, it’s not really clear that our existing process works.”
But of course, awareness is just the first step of a larger journey toward ModelOps maturity. Indeed, our research suggests that the general immaturity of enterprise ModelOps functions today may be holding many enterprise AI strategies back.
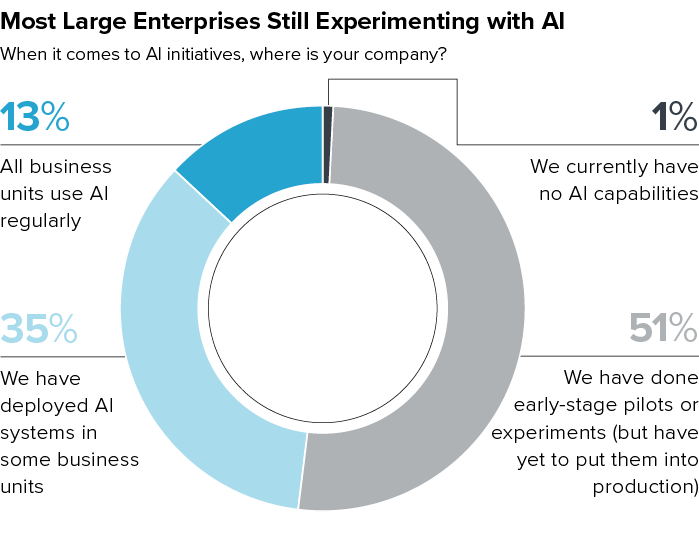
AI Maturity Varies from Enterprise to Enterprise
While past Corinium surveys have shown that many smaller enterprises are generating significant returns with their AI investments, our State of ModelOps surveys focus specifically on companies big enough to appear on the Fortune 500. When we look at these companies, a more nuanced picture of the state of enterprise AI maturity emerges.
A full 52% of the executives we surveyed say that, while they’re currently experimenting with AI, their companies still have no models in production. Meanwhile, 35% say they have deployed AI models in some business units and just 13% say all business units in their organizations use AI regularly.
At the same time, AI leaders report that many of the ModelOps processes they have in place are ineffective. Notably, 56% say their systems for maintaining an inventory of models in production are ‘not very effective’ and 42% say the same about their processes for monitoring those models.
“ModelOps maturity in spaces like retail is lower than other sectors,” notes Frederique De Letter, Director, Enterprise Data Intelligence at Domino’s.
“Spaces like banking are the most progressive in that area. They have more consumer-facing models where, if it goes wrong, it can go horribly wrong. I think that’s what drives ModelOps maturity.”
As companies attempt to put the pandemic behind them and forge a path toward the new normal, our research shows that budgets for enterprise AI strategies are rising again.
Last year, 19% of respondents said their AI budgets had remained static year-on-year and 10% said theirs had fallen, possibly due to pandemic-related business disruption. But in 2022, 94% say their AI budgets have increased, with 66% reporting an increase of at least $500,000 USD.
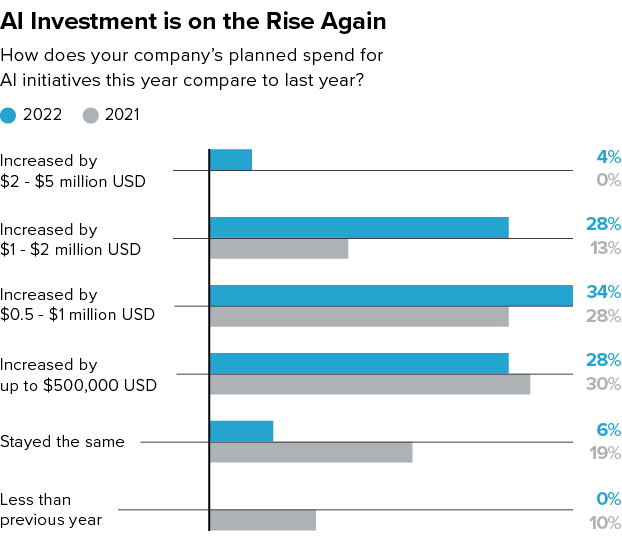
Awareness Issues Give Way to New Challenges
Despite the increased investment our research has uncovered, the executives we surveyed still say a range of challenges are hindering the effectiveness of their ModelOps functions.
A full 80% of respondents say it’s at least ‘quite challenging’ to find staff such as model operators or AI engineers, with 59% describing this as ‘very challenging’. This situation is likely compounded by the lack of a clear organizational focus for ModelOps and a tendency to duplicate functions across, and even within, business units.
Of the AI leaders we surveyed, 48% say responsibility for ModelOps currently falls to their companies’ data scientists, 40% say IT operations is involved, 32% say they have dedicated model operators or engineers and 3% say their DevOps teams are responsible.
A lack of standardized processes for ModelOps and the cost of operationalizing models are jointly the second biggest ModelOps challenges facing these executives today. For both, 74% of respondents describe them as at least ‘quite challenging’. This lack of standardization and enterprise support contributes directly to the increasing costs of ModelOps.
AI leaders’ assessments of the weaknesses in their ModelOps infrastructures also reveal some interesting surprises. A full 56% rate their model inventories as not very effective or not at all effective. Yet, 55% say their model governance is very effective.
Since effective governance starts with complete visibility into all models in production and the ability to monitor them fully, these results appear contradictory. There are several possible explanations for this, perhaps including a bias towards overstating one’s governance capabilities. Whatever the reason, there’s agreement that visibility into models in production is weak and needs to be addressed.
“I am dealing primarily with compliance risk and the fair lending sides of banks and fintechs,” notes Melissa Koide, CEO of US-based AI policy thinktank FinRegLab.
“They’re all quite attuned to, and quite anxious about, how they do governance around using more opaque models successfully.”
So, while our 2022 State of ModelOps research shows that AI leaders are taking the topic of ModelOps seriously, a lack of organizational alignment is preventing many from establishing optimal practices for governing, monitoring and maintaining AI models effectively in production.
This is an excerpt from our State of ModelOps, 2022 research report. Click here now to read the full report.