Enterprise AI is Driving the Rise of ModelOps
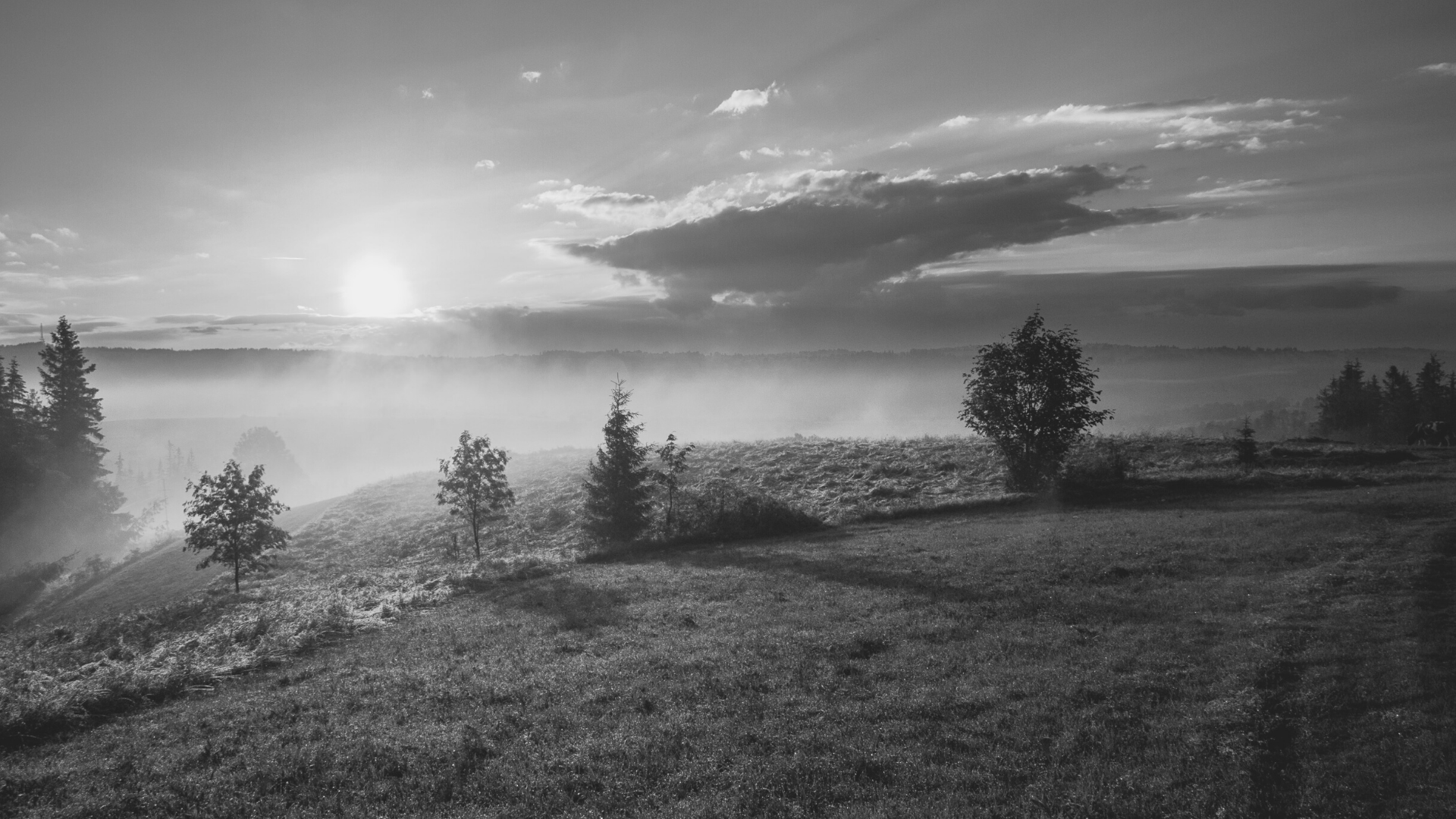
With the use of AI in business becoming more widespread, enterprises are developing mature ModelOps functions to facilitate better monitoring, managing and governance for AI systems in production
ModelOps is becoming a critical business function as more enterprises successfully deploy and scale their use of AI models.
Our survey of 100 AI-focused leaders from top financial services companies reveals that a typical enterprise of this size now has 270 models in production. One in five report that all business units in their organizations use AI regularly, while a further 60% report that at least some business units do so.
This is not entirely surprising, as the organizations we surveyed are highly advanced in their use of AI. However, the growing number of models at use in these enterprises is creating new challenges for AI-focused executives.
“For straight transactional processing, I think the model that we have works very well,” says Patricia Flynn, VP, Information Governance and Solution Delivery at State Street. “For machine learning and AI, it's not really clear that our existing process works.”
The term ‘ModelOps’ generally refers to the enterprise capability focused on governance and lifecycle management of all types of decision models, such as traditional statistical models, as well as AI models. ModelOps touches everything that happens with a model from the point at which it’s released into production until (and beyond) it’s ultimate retirement.
AI models have unique characteristics and cannot be managed and governed in the same way as conventional software. So, while the enterprises we surveyed have decades of experience with models, the growing number of AI models at use in these enterprises is placing new strains on their business processes.
AI and Model Operationalization Investment is Increasing
The prevalence of AI in business is only going to increase in 2021. Our research shows that 71% of financial services companies have increased their AI budgets in 2021, with 13% reporting an increase of more than $1 million USD.
At the same time, enterprises are also investing in ModelOps. More than half of our respondents say their enterprises already have a dedicated budget for ModelOps. A further 37% expect theirs to have one within 12 months.
Wells Fargo is one example of a large corporation that is prioritizing aspects of ModelOps such as monitoring and governance as it scales up its AI initiatives.
“That's something that was really heavily invested in over the last four years,” says Eli Bernstein, Head of Data at Wells Fargo Asset Management. “We are in the middle of building out the infrastructure that will scale.”
However, our findings show that the maturity of the ModelOps processes enterprises currently have in place varies greatly from company to company.
Many executives are running into trouble with fairly basic tasks, such as inventorying the models that are currently deployed within their organizations. Just 25% of survey respondents say the processes they have in place for this are ‘very effective’. Meanwhile, 43% would describe theirs as ‘ineffective’.
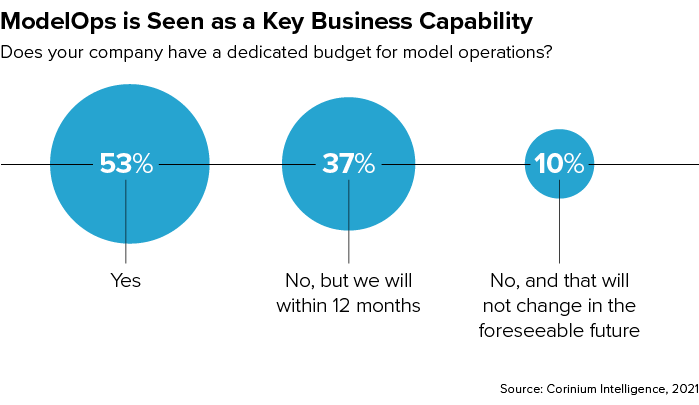
“I think it's because a lot of AI projects are so approachable now,” suggests Skip McCormick, Data Science Fellow at BNY Mellon. “It's very easy to get ahead of whatever model governance organization you already have in place for more traditional models.”
“You have this Wild West of people doing exciting things with software they download,” he adds. “Then, they encounter [ops] guys, who are like, 'Wait, you're working on a model? We have a whole governance process and you're supposed to submit forms to us before you start.’”
Governance is emerging as a key driver for investment in ModelOps. Our survey respondents cite ‘maintaining model audit trails for regulatory compliance’ and ‘ensuring visibility into the status of models’ as two of the top ModelOps challenges facing enterprises today. Respectively, only 31% and 32% say they have ‘very effective’ processes for these tasks in place.
The companies we surveyed had already been working to address model management challenges. But less than half rate their current practices as ‘very effective’. Indeed, just 41% claim they have very effective processes for standardizing model operations, 46% say they have very effective processes for monitoring AI models and 48% believe their model governance processes are very effective.
How AI Adoption is Giving Rise to ModelOps in 2021
Of course, enterprises have had ops teams to manage software and legacy (non-AI) models in production for years. But the constantly evolving nature of AI models means new processes are needed to operationalize them effectively.
“We have a very formal and rigorous model governance process and model risk management organization,” says McCormick. “What we're doing now is extending that to cover these continuously improving models.”
Our research shows that enterprises are facing a range of operational ModelOps challenges as they put more AI models into production.
Not surprisingly, risk is a key issue in the financial services sector: 80% say that difficulty managing risk and ensuring regulatory compliance is a barrier to operationalizing AI in their enterprises.
“ModelOps is the logical next step after DevOps. We're looking for a systematic way to make sure that the models we're putting into play actually do what they should do”
Skip McCormick, Data Science Fellow, BNY Mellon
Additional barriers include ‘integration with existing systems’, ‘gaps in staff and skills’, ‘process inefficiencies’, ‘lack of standardization’ and ‘cost’. Respectively, 72%, 70%, 64%, 65% and 62% of respondents cite these five hurdles as barriers to ModelOps in their organizations.
This reflects in part a historic focus on building models rather than deploying and operating them, as McCormick points out.
“A lot of AI capabilities are still at the stage where it's a lot of potential,” he concludes. “Few organizations are simultaneously putting sufficient resources into the infrastructure they'll need to have beneath that in a production environment.”
The rise of ModelOps can be seen as a response to the increasing recognition of the unique challenges of operationalizing models at scale.
Models have long been seen as critical enterprise assets, and AI models are showing their ability to deliver very significant value. Enterprises increasingly understand that to continuously capture this value while managing risk requires ModelOps practices for the age of AI. As a result, they’re investing in ModelOps.
This is an excerpt from our State of ModelOps 2021 research. To discover more about this emerging and vital business function, click here now to access the full research.