Treading the Tightrope: Balancing AI’s Potential with Privacy and Ethics Concerns
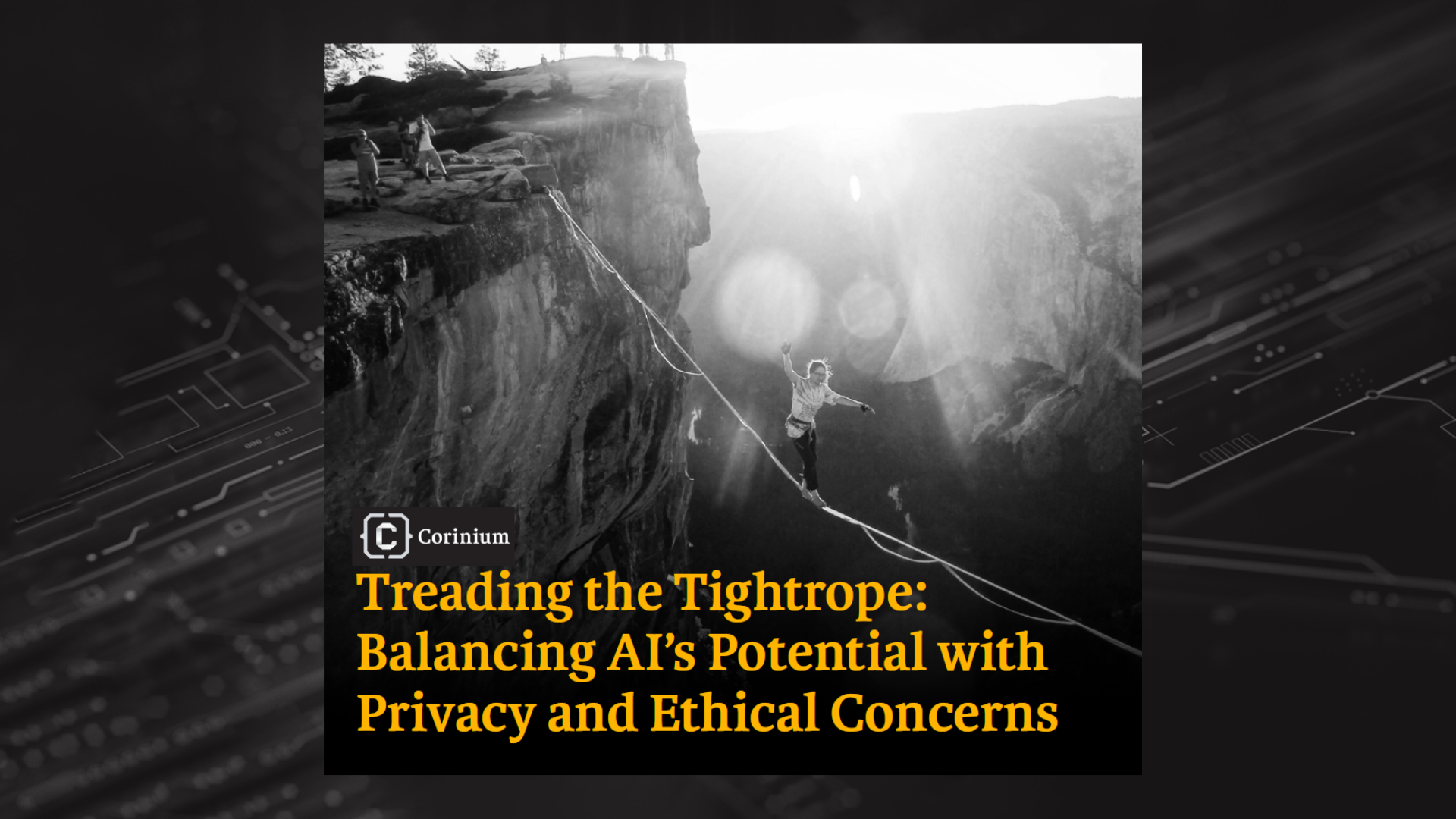
Data, analytics, and AI leaders are navigating the promise of AI and advanced analytics while weighing data quality issues as well as privacy and ethical considerations
Enterprise data and AI are crucial for modern businesses seeking to create value. But which use cases are prime for exploitation? And where might AI be less than commercially viable?
Data leaders are faced with the challenge of selecting the right AI initiatives that not only resolve friction points in the business but also lead to demonstrable value creation.
“If I were to advise executive leaders on AI strategy, I’d suggest focusing on identifying friction points within the business processes. Then, bring in experts to recommend whether AI or other technologies can address these issues,” says Cecilia Dones, Former Head of Data Sciences at Moët Hennessy.
“Creating value comes from resolving these friction points. Leaders should resist the urge to deploy AI indiscriminately. The goal should always be value creation— finding ways to reduce friction within the ecosystem.”
“The business side understands the data and sometimes the potential of AI and machine learning, but they might not know where or how to apply it,” adds Ines Ashton, Director of Advance Analytics at Mars Petcare.
“Balancing feasibility with value is crucial. AI and machine learning can be exciting for business, but they must be applied where the value generated outweighs the cost.”
Additionally, technological partnerships play a pivotal role in the success of AI initiatives. Knowing when to employ external assistance might be a far more cost-effective solution than attempting to develop one in-house.
Our research shows that ‘leveraging technology partnerships’ is a top priority for 65% of organizations when it comes to AI investments.
Zachary Elewitz, Director of Data Science at Wex, emphasizes this point: “Just because AI can be used doesn’t mean we should always develop it in-house. There are countless tools available, and sometimes external solutions can offer value more rapidly and cost-effectively.”
Empowering AI Initiatives with Quality Data
In a world where businesses increasingly rely on analytics and AI for decision-making and innovation, the quality of data fed into these systems is crucial. Data and analytics leaders must consider several key aspects when preparing data for AI and analytics initiatives.
Our research with 100 C-suite leaders and senior decision-makers in Data, AI, and Innovation shows that the most important data preparation aspects to consider are: ‘maintaining data integrity and quality’ (60%), ‘categorizing or classifying data for easier analysis’ (48%), and ‘cleaning data to remove noise and errors’ (46%).
Vivek Soneja, Corporate Vice President - AI, Analytics Data and Research at WNS Triange, stresses the need for a strategic approach to AI implementation and the role of data quality in that strategy.
“AI is already present in many organizations. But for AI to be a game-changer, businesses need a strategic approach. Implementation often faces issues due to data quality, which needs addressing,” Soneja notes.
“Feeding poor data into an AI system results in equally poor output. Enterprises need advanced data engineering and governance to maintain clean data, especially if AI will increasingly complement human decision-making in the future.”
“Poor data quality can lead to significantly flawed outcomes,” Agarwal adds. “Inadequate data quality can negatively affect AI outcomes, resulting in inaccurate predictions or ‘hallucinations’ in large language models. Additionally, there could be regulatory repercussions if reports based on poor-quality data are found to be inaccurate.”
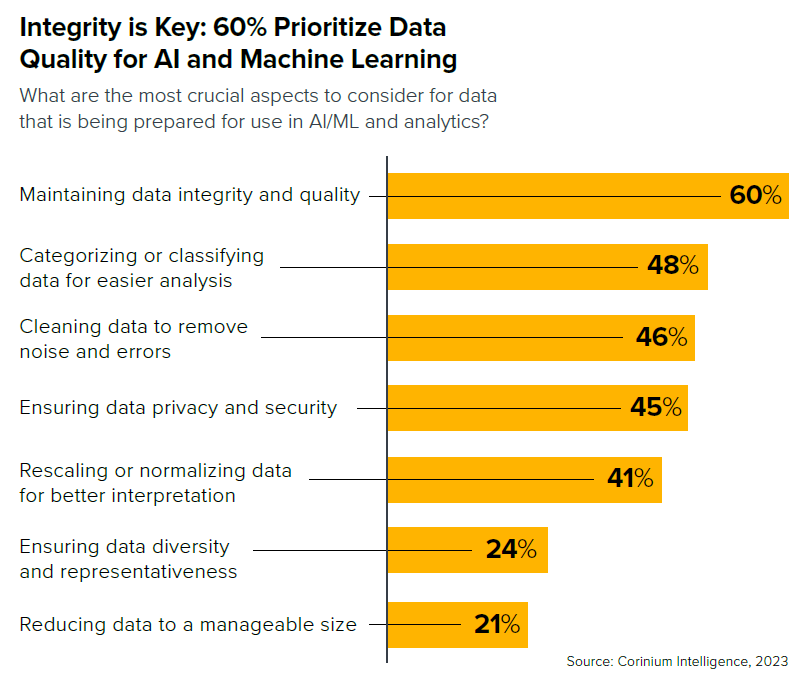
Empowering AI Initiatives with Quality Data
Businesses increasingly rely on analytics and AI for decision-making and innovation, so the quality of data fed into these systems is crucial. Data and analytics leaders must consider several key aspects when preparing data for AI and analytics initiatives.
Our research with 100 C-suite leaders and senior decision-makers in Data, AI, and Innovation shows that the most important data preparation aspects to consider are: ‘maintaining data integrity and quality’ (60%), ‘categorizing or classifying data for easier analysis’ (48%), and ‘cleaning data to remove noise and errors’ (46%).
Vivek Soneja, Corporate Vice President - AI, Analytics Data and Research at WNS Triange, stresses the need for a strategic approach to AI implementation and the role of data quality in that strategy.
“AI is already present in many organizations. But for AI to be a game-changer, businesses need a strategic approach. Implementation often faces issues due to data quality, which needs addressing,” Soneja notes.
“Feeding poor data into an AI system results in equally poor output. Enterprises need advanced data engineering and governance to maintain clean data, especially if AI will increasingly complement human decision-making in the future.”
“Poor data quality can lead to significantly flawed outcomes,” Agarwal adds. “Inadequate data quality can negatively affect AI outcomes, resulting in inaccurate predictions or ‘hallucinations’ in large language models. Additionally, there could be regulatory repercussions if reports based on poor-quality data are found to be inaccurate.”
Challenges When Scaling AI Initiatives
Scaling AI initiatives in enterprises comes with its own set of challenges, which are underscored by the findings of our research.
These challenges include ‘building trust in AI systems among stakeholders’ at 55%, ‘scarcity or lack of quality data for AI training’ at 47%, and ‘high cost of AI implementation and maintenance’ at 42%.
Ravindra Salavi, Senior Vice President – AI, Analytics Data and Research at WNS Triange emphasized these challenges and others: “Many of these challenges tie back to the aspects of starting correctly and ensuring continuous improvement. Initial challenges arise if there’s insufficient data, lack of skillset, or no clear use case.”
He continues: “As companies scale AI, they need wider and deeper data – spanning multiple domains and historical data. Another challenge is the need for skilled resources and increased involvement from stakeholders. An AI initiative won’t succeed if it’s limited to just the engineering and data science teams.”
Salavi’s insights, combined with our research findings, underline the importance of building trust among stakeholders, having sufficient and wide-ranging data, and skilled resources from the outset.
Organizations need to prioritize maintaining data integrity and quality, categorizing, or classifying data for easier analysis, and cleaning data to remove noise and errors. This will ensure that the AI systems are fed with clean and accurate data, leading to more accurate and reliable outcomes.
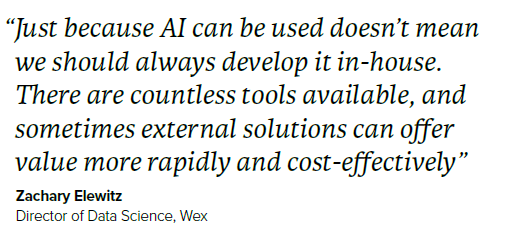
Avoiding an ‘Accountability Crisis’ in AI Decision-Making
The swift expansion and utilization of AI in various enterprise sectors, especially in heavily regulated industries such as financial services, banking, and healthcare, has brought concerns about data security and privacy into sharp focus.
Our research highlights key concerns among data, analytics, and AI leaders when it comes to data privacy and security as it relates to AI use. Organizations face several challenges, primarily ‘risks of model poisoning or adversarial attacks’ at 66%, ‘ensuring data privacy during AI processing’ at 52%, and ‘compliance with data protection regulations in AI use’ at 47%.
“We need clear strategies and policies to ensure we’re not infringing on privacy or ethics. With the vast amount of data available, it’s possible to know intricate details about a person’s life. Organizations must establish boundaries,” says Vivek Soneja, Corporate Vice President - AI, Analytics Data and Research at WNS Triange.
This concern about AI decision-making was echoed in our research findings. A remarkable 72% of respondents are extremely concerned about ‘AI decision-making accountability,’ making it the most significant ethical concern related to AI use.
This ‘accountability crisis’ underscores the importance of understanding and controlling AI actions to prevent uncontrollable or dangerous situations.
“Algorithmic objectivity can be compromised if algorithms unintentionally reflect human biases. Addressing these challenges is crucial for ethical AI deployment, and defining accountability is essential,” says Anish Agarwal, Global Head of Analytics at Dr. Reddy’s Laboratories.
“Some organizations now have a Chief Ethics Officer responsible for ethical standards and accountability,” he continues. “In the context of data, informed consent is vital, ensuring individuals are aware of how their data is used.”
As enterprises continue to undertake AI initiatives, it is crucial to proactively address data privacy and security concerns. Organizations must create clear strategies and policies and understand and control AI actions without breaking social contracts. By addressing these concerns, organizations can harness the power of AI while maintaining trust and ethical integrity.
This article is an extract from our recent industry report: The Future of Enterprise Data and AI. You can download the full research report here