Could Localized LLMs Revolutionize the Fight Against Financial Fraud?
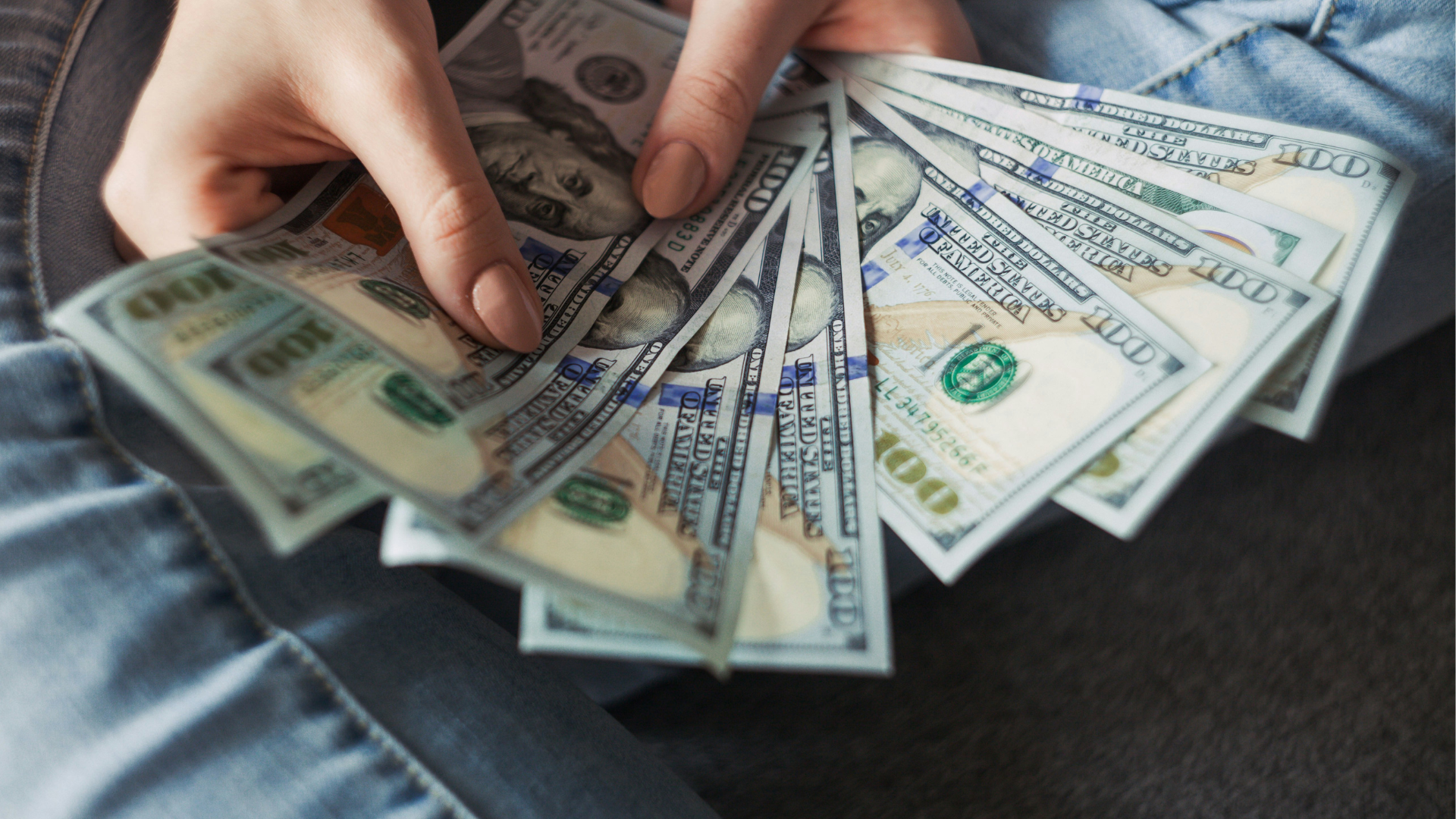
Financial fraud costs financial services firms billions worldwide. And while anti-fraud measures have become more sophisticated, so have the activities of the fraudsters. Now, an experimental approach to the use of AI and Large Language Models (LLMs) hints at how financial services firms may use this groundbreaking technology to revolutionize fraud prevention
In North America, it is estimated that financial firms lose 5% of their revenue each year to fraud. What’s more, the cost of these losses is rising, with each dollar lost to fraud costing financial firms $4.36 in related expenses. A recent report from Nasdaq estimates that fraud schemes cost financial firms USD 151.1 billion in the Americas in 2023.
In the world of finance, trust is paramount. Customers rely on banks and financial institutions to safeguard their assets and protect their sensitive information. However, with the rise of sophisticated cyber threats and financial fraud schemes, maintaining this trust has become increasingly challenging. Traditional methods of fraud detection are often reactive and can overlook subtle patterns indicative of fraudulent activity.
Already, we are seeing leaders in data and analytics experimenting with localized networks of LLMs. This kind of network could work in concert to analyze vast amounts of transactional data in real time helping to reduce the impact of financial fraud. Unlike centralized systems that require banks to relinquish control of their proprietary data, a distributed network of LLMs would allow institutions to retain sovereignty over their information, addressing concerns regarding privacy and ethics.
In a recent interview with Corinium Global Intelligence, Dean McKeown, Interim Director and Master of Management in Artificial Intelligence at the Smith School of Business at Queen’s University in Canada said: “What I see now, despite us referring to them as large language models, is a trend towards numerous smaller LLMs. We'll be able to tailor them for specific purposes.
“The future lies in understanding the technology and operationalizing it affordably. Another critical aspect of the next iteration of LLMs is building checks and balances to prevent issues like hallucinations and false reporting.”
Enhancing Localized Fraud Prevention
Leveraging the power of natural language processing and machine learning, LLMs can meticulously analyze patterns in transactional data, offering a proactive approach to identifying anomalies. By scrutinizing vast amounts of data with unparalleled speed and accuracy, LLMs enable financial institutions to stay one step ahead of fraudsters, mitigating losses and safeguarding customer assets.
Paul Twigg, Chief Technology Officer at Digital Commerce Bank recently told Corinium Global Intelligence that he thinks a localized approach to the use of LLMs could be the next step in fighting financial fraud.
“Every single bank in the US has a fraud module in its core banking system and a dedicated fraud team. Still, globally there are trillions of dollars of fraud in the banking system every year.
“The technology behind LLMs is amazingly useful, so if we start applying domain-specific knowledge to the technology of an LLM, all of a sudden, we can get something that's extremely powerful.”
One of the key advantages of localized LLMs lies in their ability to capture region-specific nuances, a critical aspect often overlooked by centralized fraud detection systems. Each geographic region possesses unique transactional behaviors, cultural norms, and regulatory landscapes, which can significantly impact the manifestation of fraudulent activity. Localized LLMs could effectively reveal these subtleties, allowing financial institutions to tailor their fraud detection strategies to the specific characteristics of their operating markets. Moreover, as fraud tactics evolve, localized models offer the flexibility to adapt and evolve alongside emerging threats.
By harnessing the granular insights provided by localized LLMs, financial institutions can strengthen their defenses against fraud while minimizing false positives and preserving the integrity of legitimate transactions.
Ensuring Data Privacy and Security
With the increasing digitization of financial services and the growing volume of personal and financial data stored and processed by banks and other institutions, the need to protect sensitive information has never been more critical. Centralized fraud detection systems often require the sharing of vast amounts of data between institutions, raising concerns about data sovereignty and the potential for breaches or unauthorized access. Decentralized LLMs offer a potential solution to these concerns by keeping sensitive information within the confines of individual institutions.
“With a domain-specific LLM focused on financial fraud, banks could in theory share data with other banks at an aggregated level, so no personal information is shared,” said Twigg. “This will allow banks to better understand the patterns of fraud and reduce or even eliminate a cost that weighs heavily on the global banking industry.”
This approach not only enhances data privacy but also strengthens security by reducing the attack surface and limiting the potential impact of data breaches. Moreover, decentralized LLMs empower financial institutions to comply with stringent data protection regulations.
By design, a distributed model could better align with the principles of data minimization and purpose limitation, ensuring that data is processed lawfully, transparently, and for specified purposes. This alignment with regulatory requirements not only mitigates the risk of non-compliance but also fosters trust and confidence among customers, who entrust financial institutions with their sensitive information.
Implementation Challenges and Concerns
As financial institutions adopt localized Language Models (LLMs) to bolster their fraud detection capabilities, several challenges and considerations arise that must be addressed to ensure the effectiveness and integrity of these systems. One significant challenge is interoperability between disparate systems used by different institutions.
Each firm may have its own infrastructure and data formats, and seamless integration and communication between localized LLMs would be complex. Standardizing data formats and establishing interoperability protocols will be essential to facilitate smooth collaboration and information sharing among institutions.
What’s more, ensuring the integrity and reliability of LLMs is paramount to their effectiveness in detecting fraudulent activity. These models rely on vast amounts of data to learn patterns and make predictions, making them vulnerable to manipulation or adversarial attacks. Robust security measures, including encryption, access controls, and continuous monitoring, are necessary to safeguard against unauthorized access or tampering.
Additionally, the need for transparency and accountability in the operation of localized LLM networks underscores the importance of robust governance frameworks. Clear policies and procedures governing data access, usage, and sharing are essential to mitigate risks associated with malicious actors and ensure compliance with regulatory requirements.
“Another critical aspect of the next iteration of LLMs is building checks and balances to prevent issues like hallucinations and false reporting,” said McKeown. “We've seen instances, especially in academia, where models provide fabricated sources. This is concerning, as are the social biases embedded in some models, which can pose significant risks.”
Collaborative efforts among industry stakeholders, regulatory bodies, data and analytics leaders, and cybersecurity experts will be instrumental in developing and implementing these governance frameworks, fostering trust and confidence in the reliability and integrity of localized LLM networks.
Looking to the Future
In the ever-evolving landscape of financial fraud prevention, the potential of LLMs to revolutionize the fight against illicit activities is both promising and compelling.
A network of distributed LLMs could empower financial institutions to proactively detect and mitigate fraud while preserving the privacy and sovereignty of customer data. By leveraging region-specific nuances and adapting to evolving fraud tactics, these models hold the key to staying one step ahead of sophisticated perpetrators.
However, as with any transformative technology, there are several key challenges and considerations. From interoperability issues to ensuring the integrity and reliability of LLMs, the journey towards widespread adoption is not without its hurdles. Yet, it is in overcoming these obstacles that the true potential of localized LLM networks will be realized.
Moving forward, collaborative efforts among industry stakeholders, regulatory bodies, and cybersecurity experts will be key to developing robust anti-fraud strategies that can move the needle on reducing financial fraud.
Want to learn more?
Paul and Dean will be speaking at CDAO Canada on March 26th-27th, 2024 in Toronto. Join them and many other data and analytics leaders to learn about the latest trends and opportunities in the industry. Register to attend here.