Navigating the Future of Data Engineering: Tiffany Qiao
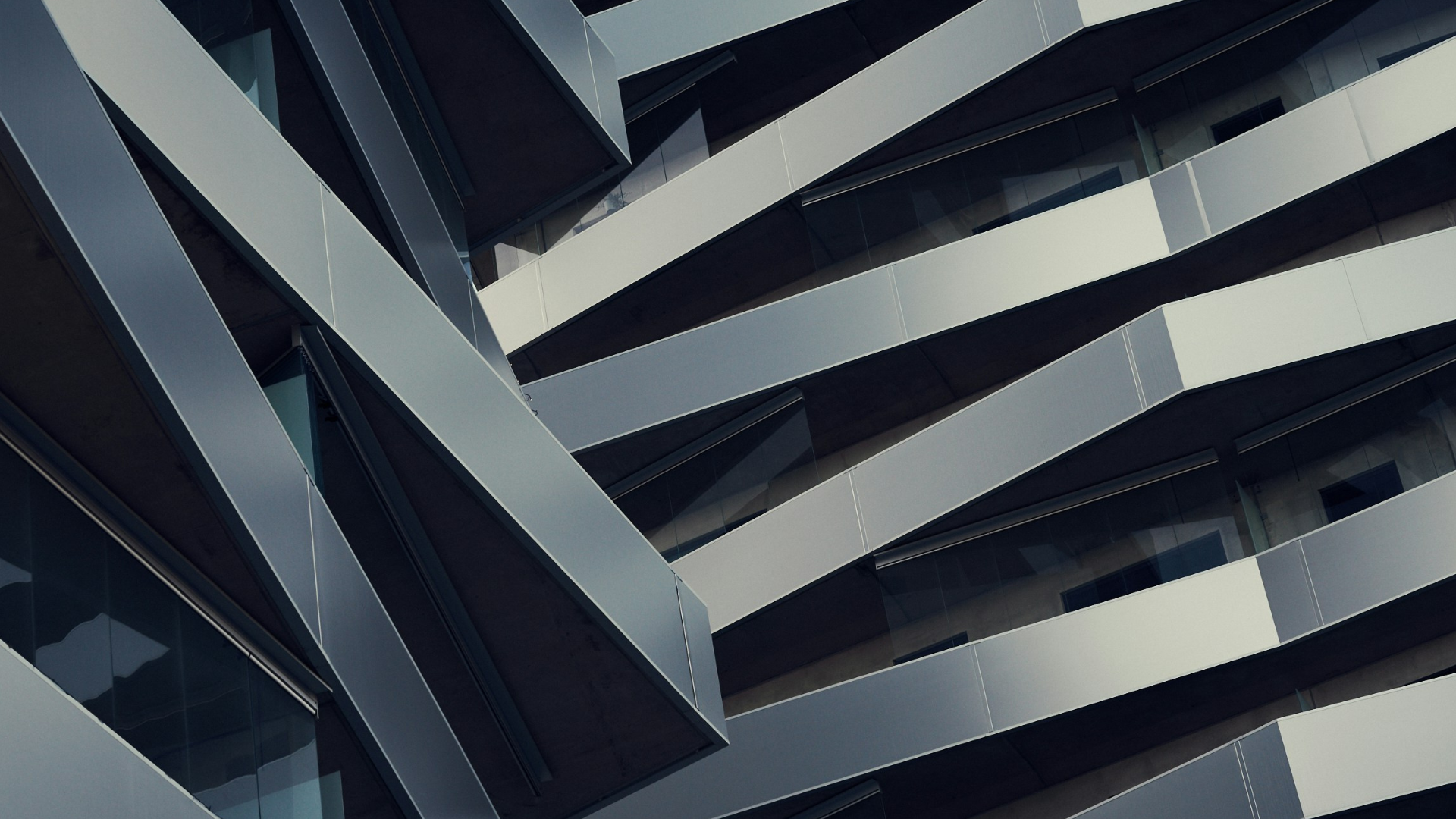
Tiffany Qiao, Senior Data Engineer for AI and Innovation at the world-leading jewelry brand Pandora, has a rich background in finance and technology. After an eye-opening educational journey in the U.S., where she interacted with tech giants like Google and Apple, she was inspired to pursue a master’s in computer science. Specializing in financial modeling with Java and Python, she seamlessly transitioned to data engineering, blending her robust financial knowledge with cutting-edge tech skills to innovate in business intelligence and AI.
Ahead of her talk at the Data Architecture London, we had the opportunity to sit down with Tiffany Qiao, Senior Data Engineer for AI and Innovation at the world-renowned jewelry brand, Pandora.
In this interview, Tiffany discusses the critical trends shaping the industry, such as the widespread adoption of cloud infrastructure and the integration of AI into everyday business processes. She also offers practical advice for data engineers and business leaders aiming to stay ahead of the curve and transform their data practices effectively.
Don't miss out on this opportunity to gain industry-leading insights! Register now for Data Architecture London to hear directly from Tiffany Qiao and other top professionals about the future of data engineering and AI.
C: What are the key trends you see shaping the future of data engineering and AI in the industry today?
TQ: The ongoing adoption of cloud infrastructure is definitely a key trend shaping the future of data engineering and AI. Major tech companies like Google, Microsoft, and Amazon are heavily investing in AI tools and cloud platforms such as GCP, AWS, and Azure. The move towards cloud-native architectures is accelerating, driven by the scalability, flexibility, and cost-efficiency of these platforms. This trend is no longer limited to large enterprises; sectors ranging from luxury retail to healthcare and gaming are also transitioning to the cloud. Even mid-sized companies must adopt cloud solutions to remain competitive and manage their data effectively.
Another significant trend is the integration of AI into existing platforms. AI and machine learning are increasingly being embedded into data engineering workflows, automating complex tasks and enabling smarter data management. This trend also makes data more accessible to non-technical employees.
For example, AI tools like Genie in Databricks allow anyone within a company to retrieve market insights without needing coding skills. This democratization of data is transformative, empowering all employees to utilize data more effectively, driving faster decision-making, and enhancing business agility.
C: What advice would you give to data engineers and business leaders looking to stay ahead of industry trends and effectively transform their data practices?
TQ: Continuous learning is essential for both data professionals and business leaders. Keeping up with rapidly evolving technologies and methodologies in data engineering and AI is crucial, not just for personal growth but for fostering a culture of learning within the organization.
Investing in AI and automation tools is also vital. Embracing these technologies can streamline data management processes, enhance operational efficiency, and drive value across the organization. This investment should be complemented by a strong focus on data governance. Implementing robust data governance frameworks ensures data quality, compliance, and security, which are increasingly critical from both legal and operational perspectives.
Promoting collaboration between data teams and business units is key. Encourage alignment between data initiatives and business goals to maximize value. It's important to address any misconceptions about data teams being just IT support—they play a strategic role in business success.
Finally, be open to experimentation and innovation. Allow teams the freedom to explore and run proof-of-concept (POC) projects. These initiatives, whether successful or not, are valuable learning experiences that can lead to adopting new technologies and approaches, ultimately providing a competitive edge.
C: For organizations that are still early in developing a data-driven culture, what strategies can help elevate awareness and education among stakeholders within the business?
TQ: To successfully build a data-driven culture, strong top-down support is critical. Relying solely on bottom-up initiatives can be challenging. Senior leadership, such as the Chief Data Officer (CDO) or Directors, must recognize the value of AI and data. Their endorsement helps shape the organization’s strategic direction and establish core values that align with a data-driven mindset.
Another important strategy is to lead by example and encourage experimentation and innovation within teams. Demonstrations are particularly effective in this regard, as they provide a platform for communicating with various stakeholders, showcasing ongoing projects, and highlighting potential improvements.
This approach fosters a collaborative environment where ideas are openly shared, solutions are developed collectively, and a positive, inclusive culture extends beyond the core tech or data teams to the entire organization.
C: For organizations looking to build or enhance their data-driven culture, what are some effective strategies to ensure alignment and foster collaboration across different teams?
TQ: To build a strong data-driven culture, it's essential to start with strategic alignment by designing a data architecture that directly supports the organization's key business objectives. This requires close collaboration between data engineering teams and business leaders to ensure a deep understanding of strategic goals. Such alignment fosters ongoing collaboration and encourages regular interactions across teams, ensuring that data initiatives are consistently aligned with business priorities.
Another crucial aspect is the scalability and flexibility of your data architecture. It needs to be adaptable and capable of managing the growing volume and speed of streaming data, enabling real-time processing and analytics. In my experience, we initially encountered issues with architectures that lacked the necessary scalability and flexibility, which pushed us to continually refine and enhance our frameworks.
Moreover, building integrated data pipelines that can efficiently ingest, process, and deliver streaming data to the right stakeholders is critical to meeting business requirements. A well-integrated pipeline ensures smooth data flow and aligns with business objectives. We implement continuous integration and deployment (CI/CD) practices to keep our development environment up-to-date and running efficiently.
Lastly, continuous monitoring and optimization of the data architecture are essential. Regular performance evaluations and adjustments ensure the system adapts to changing business needs and stays aligned with our strategic goals. This ongoing cycle of assessment and improvement is crucial for maintaining an agile and effective data-driven environment.
As technology and methodologies evolve, what are the risks for organizations, particularly mid-sized ones, as they work to catch up with larger companies already proficient in advanced tech and data practices?
TQ: One major risk is the potential loss of talent. Today’s tech professionals, such as engineers and data architects, actively seek workplaces that leverage modern tech stacks. For example, they are drawn to companies using cutting-edge cloud solutions like Microsoft Azure or platforms like Databricks, which are leading the way in current industry trends.
When a company relies on outdated technology, it not only struggles to attract new talent but also faces difficulties in maintaining its existing systems. From my experience in the banking sector, which is the banking sector has historically been slower to adopt new technologies compared to other industries, the shift to cloud-based solutions and business intelligence tools has dramatically sped up processes that once took months.
Organizations that stick with obsolete systems risk entering a downward spiral: as skilled professionals become harder to retain or hire, maintaining those aging systems becomes even more challenging, further compounding the issue of technological obsolescence.
_____
Register now for Data Architecture London to hear directly from Tiffany Qiao and other top professionals about the future of data engineering and AI.