Gen AI's Transformative Impact in the Financial Sector with ASX's Dan Chesterman
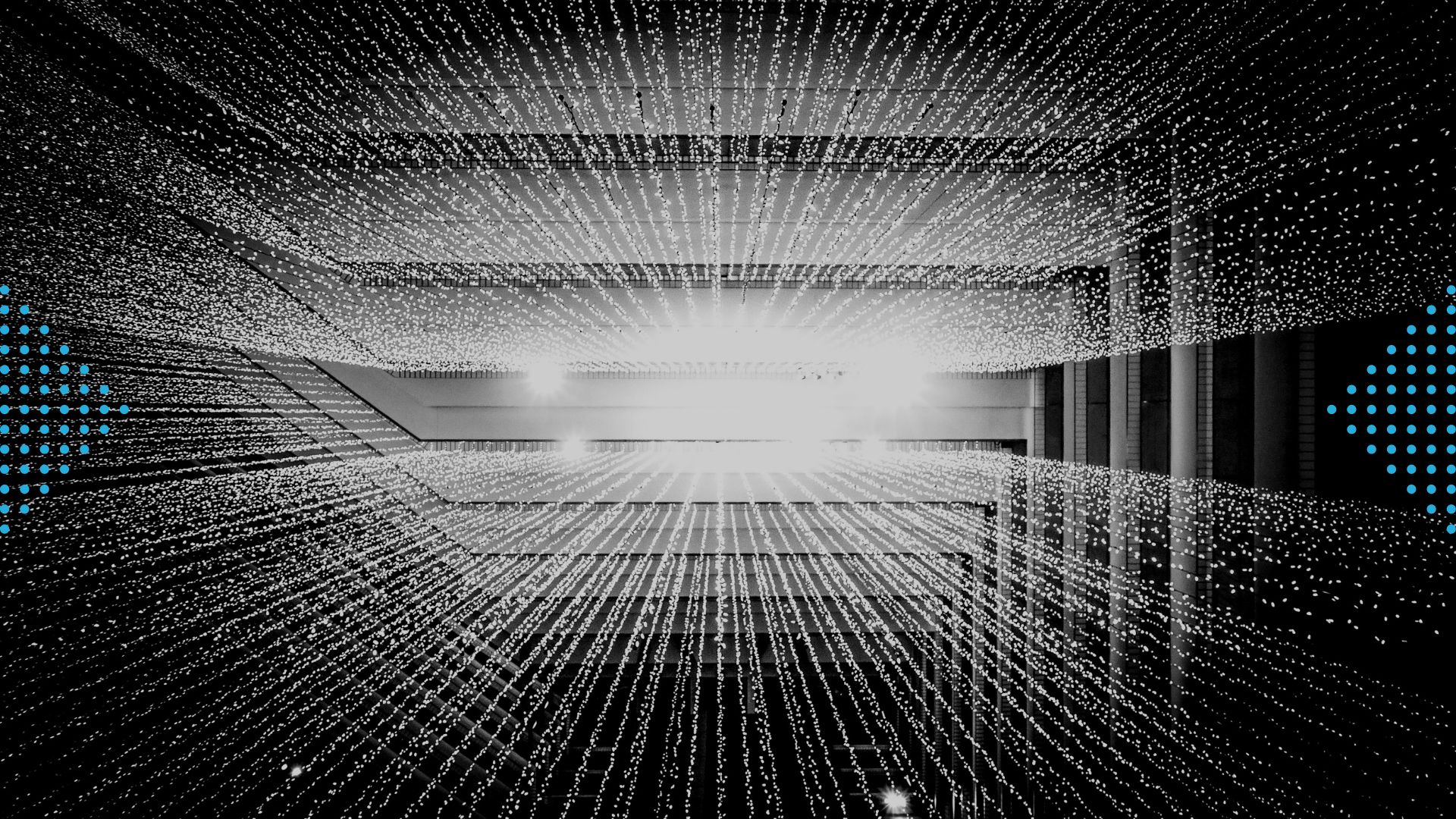
Corinium's Vanessa Jalleh interviewed Dan Chesterman, Group Executive, Technology and Data at ASX, on how advancements in AI are changing the Financial Sector, touching upon both growth opportunities as well as the security and ethical risks
How do you see AI transforming the financial sector and what initiatives could help organisations become AI-savvy?
There are already many examples of AI being used in financial services, for example to support faster product launches and innovation, to automate and execute trading strategies, to tailor a customer’s engagement with their bank, and to enhance fraud detection. In the future it is reasonable to expect that AI will continue to have a significant impact on the industry driving faster processes, hyper-personalised experiences, and enhancements to risk management and anomaly detection.
To realise the benefits of AI, organisations need to become AI-savvy. This will vary across sectors and but there’s a real risk that organisations risk falling behind without developing an AI capability. Some key ways organisations can become AI-savvy include:
- Implementing strategic frameworks: Develop robust AI implementation plans that prioritise opportunities within legal and ethical boundaries.
- Developing a data-centric culture: Ensure high-quality data with a clear understanding of its limitations.
- Ensure you are building for AI: Design solutions with modularity and easy data access to facilitate future AI integration.
- Invest in people: Cultivate an "AI-savvy" workforce by fostering interest in AI solutions.
By adopting these initiatives, financial institutions can not only leverage AI's transformative power but also redefine industry standards and customer expectations.
What are some of the ethical considerations that have come to light in the last few months or last year around the usage of generative AI?
The rapid rise of generative AI has highlighted a range of ethical concerns, some of which exist in traditional AI or machine learning and some of which are novel. There are some key issues that have emerged or have been reinforced in recent months including:
- Data Privacy: Oversharing personal information with AI systems raises concerns about misuse and unauthorised access. There have been a number of significant breaches globally which underscore the vulnerability of personal information. Additionally, individuals may unknowingly consent to data use that goes beyond their expectations, potentially leading to privacy violations.
- Copyright Infringement: Generative AI's ability to learn from existing content raises copyright concerns. There have already been lawsuits against AI models accused of "systematic theft" by using copyrighted material for training, illustrate this growing tension.
- Misinformation Warfare: Deepfakes, highly realistic AI-generated videos, threaten to erode trust in what we see and hear. Recent deepfake robocalls impersonating political figures like Joe Biden or public company CEOs showcase the potential for manipulating public opinion and influencing decisions.
To navigate these complexities, we need comprehensive ethical frameworks, transparency in AI development, and inclusive discussions. Equipping employees with data literacy, ethical reasoning, and critical thinking skills is crucial. This multifaceted approach will ensure responsible AI development while unlocking its notable potential.
What is your approach to AI governance and what strategies can data leaders implement to deliver results?
At ASX, our approach to AI governance centres on our existing Data Governance mechanisms and forums. For example, we have a Data Governance Group which oversees a whitelisting process, ensuring strong alignment between the potential business benefits of AI initiatives and the associated risks. This includes careful consideration of data privacy, regulatory compliance, and legal requirements.
To achieve success with any new technology, including AI, we ensure alignment with the group’s strategic plan, and focus on a measured and cautious approach. We begin with well-defined Proof-of-Concepts that target specific business benefits and have clear metrics for measuring outcomes. Additionally, recognising the rapid advancements in AI, we seek to leverage partnerships with organisations that have a proven track record and depth of capability in AI and ML.
AI Metrics: How can organisations benchmark AI success? What is the value system that they can use to show AI is effective?
In my view, the measurement of success for each individual use case is likely to be agnostic to the technology or approach that is used, rather it would depend on the nature of the benefit targeted by the initiative. AI should be viewed as an enabler - the fact AI was used, or not used may well be immaterial to the success of the initiative. A counterpoint to this argument is that it is possible, and in some cases advantageous, to measure an organisation’s AI savviness and data proficiency through measuring employee capabilities (including credentials and/or skills assessments, use of data in decision making and product development, revenue from data products, data quality and accessibility and customer satisfaction.
Have end-user-vendor relationships and partnerships changed since ChatGPT entered the market? Is there greater collaboration, transparency and communication between the two parties?
The launch of ChatGPT created significant interest in generative AI specifically and AI/ML more broadly. This triggered a meaningful increase in the demand for AI-related services and technologies as industries grappled with working out how it could be applied in their context.
As has been the case in other technology cycles this can lead to an initial overestimation of the potential use cases and benefits, followed by a trough of disillusionment where the hard work associated with wrangling data, determining appropriate policies, controls and addressing inevitable risks is conducted. This cycle is not unique to AI, and truly valuable end-user-vendor partnerships are those based on pragmatic and informed advice, mutual trust and aligned values.
Dan Chesterman was a speaker at our highly successful CDAO Sydney event. If you want to find out more about how businesses are evolving with the advancements in AI, we have some amazing sessions coming up at CDAO Melbourne happening 2-4 September 2024, check out the agenda here.