DataOps and the Industrialization of AI
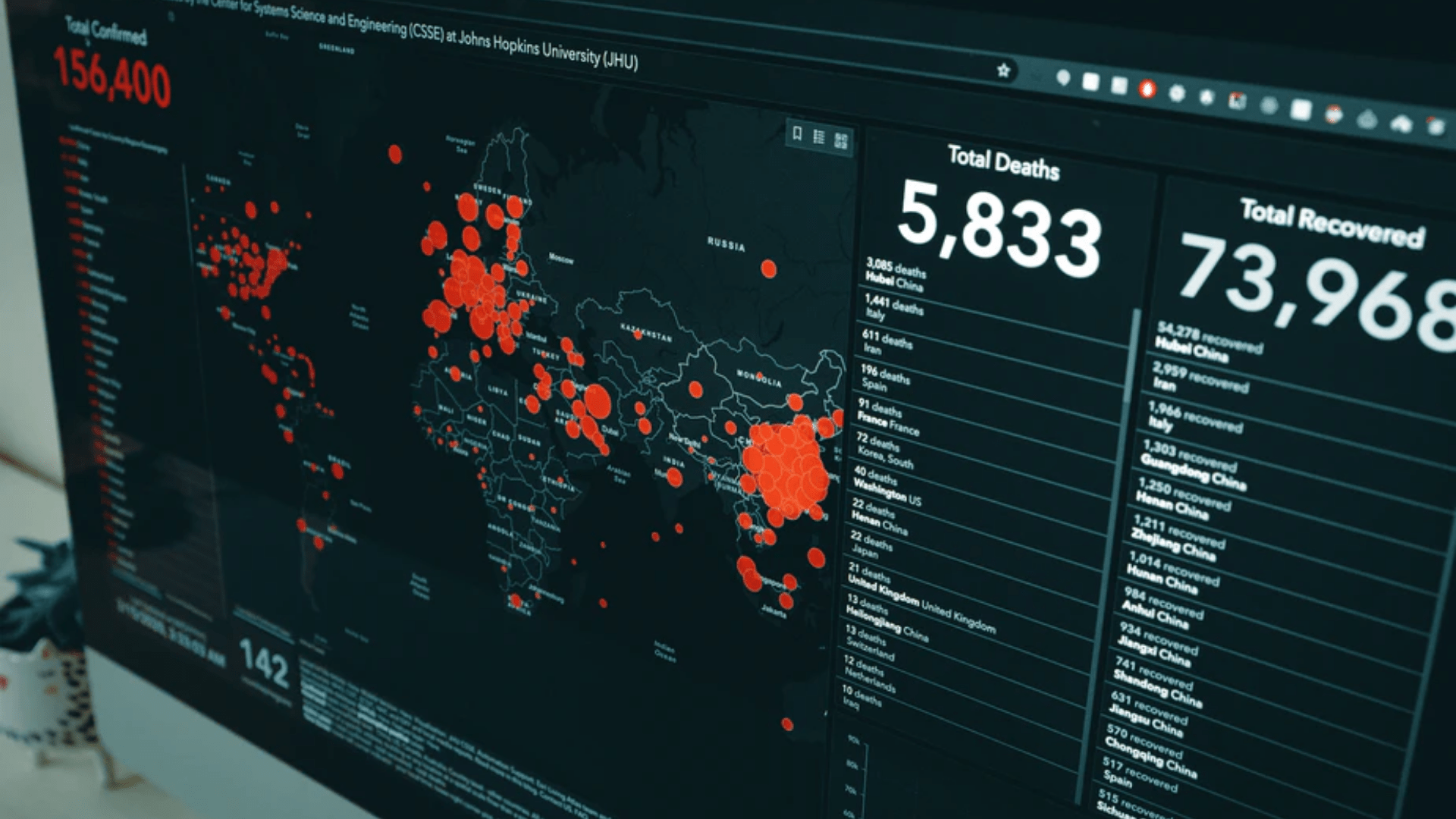
HPE Global Field CTO Matt Maccaux explored why so many enterprises struggle to operationalize AI in his 2020 Data Champions Online US keynote presentation
Why Enterprises Struggle to Industrialize AI
Industrialization is the process of expanding the application of a technology so that a business can benefit from it at scale.
Enterprises must take this leap to truly realize the benefits of AI capabilities. But as HPE Global Field CTO Matt Maccaux noted in his recent keynote presentation at Data Champions Online, US, most struggle to reach this stage of AI maturity. As Gartner reports, 60% of the AI models that are developed never make it into production.
“It’s a sort of ‘last mile’ problem,” Maccaux quipped. “They go through the entire data science life cycle but aren’t able to actually operationalize their models.”
To help data and analytics leaders overcome this hurdle, he identified three common obstacles that prevent AI industrialization and explored how they can be removed by optimizing the data life cycle.
Three Stumbling Blocks for AI Industrialization
Maccaux argued that organizational friction, legacy infrastructure and outdated business processes are the three most common challenges enterprises face when industrializing AI.
The first of these obstacles stems from the presence of organizational silos that stifle cross functional collaboration. Data scientists must work with business units to understand the problems they must solve, while also collaborating with software developers, data engineers, operations specialists and cyber security experts to deliver solutions efficiently.
“The industrialization process isn’t just about the data scientists themselves,” Maccaux said. “It encompasses a broader network of players that have got to work together.”
At the same time, many IT departments make the mistake of treating data scientists like software developers. Maccaux argued that data scientists need access to the latest tools and the freedom to embrace experimentation if they are to solve business problems successfully.
“When we talk about data scientists, they are developers, but they’re not software developers like the organization is comfortable with,” he remarked. “The traditional tooling and infrastructure are not designed to support [experimentation] at any enterprise scale.”
Finally, even the most accurate data models will fall flat if the organization using them fails to update its business processes to act on the new data-driven insights they generate.
Maccaux concluded: “Just because a data scientist can build a working model using the latest and greatest open source tools, and even if we can get that model to run in an operational environment, it doesn’t mean the organization is going to change around that.”
The Role of CICD in Industrializing AI
A key step when addressing these hurdles is to establish a data science center of excellence to work alongside the data science team and determine what tools, models and best practices are right for an organization’s needs.
But Maccaux argued that it’s equally important to build a ‘data pipeline’ that fosters collaboration and supports a cycle of continuous improvement and development. In the world of DataOps, this is commonly abbreviated as ‘CICD’.
To achieve this, data scientists must work closely with software engineers to ensure the code they write will run properly in their companies’ production environments.
“They need to operate in parallel in this shared environment,” he said. “So, as the data scientist is iterating, so does the software engineer.”
At the same time, they will need to partner with the BI analysts who will build reports or dashboards to monitor how effective the model in question is and spot when it starts to decay.
When a model’s accuracy starts to fall or the business’ needs shift, they will need to go back and update it. As such, having processes in place that record crucial metadata about how an algorithm works each time it’s deployed will save a great deal of time in the long run.
“What this requires is all of these different departments working together in a virtuous lifecycle,” Maccaux concluded. “If we treat them as independent, disconnected groups, we’re never going to get to that level of industrialization.”