How HSBC is Using Data to Detect Money Laundering and Fraud
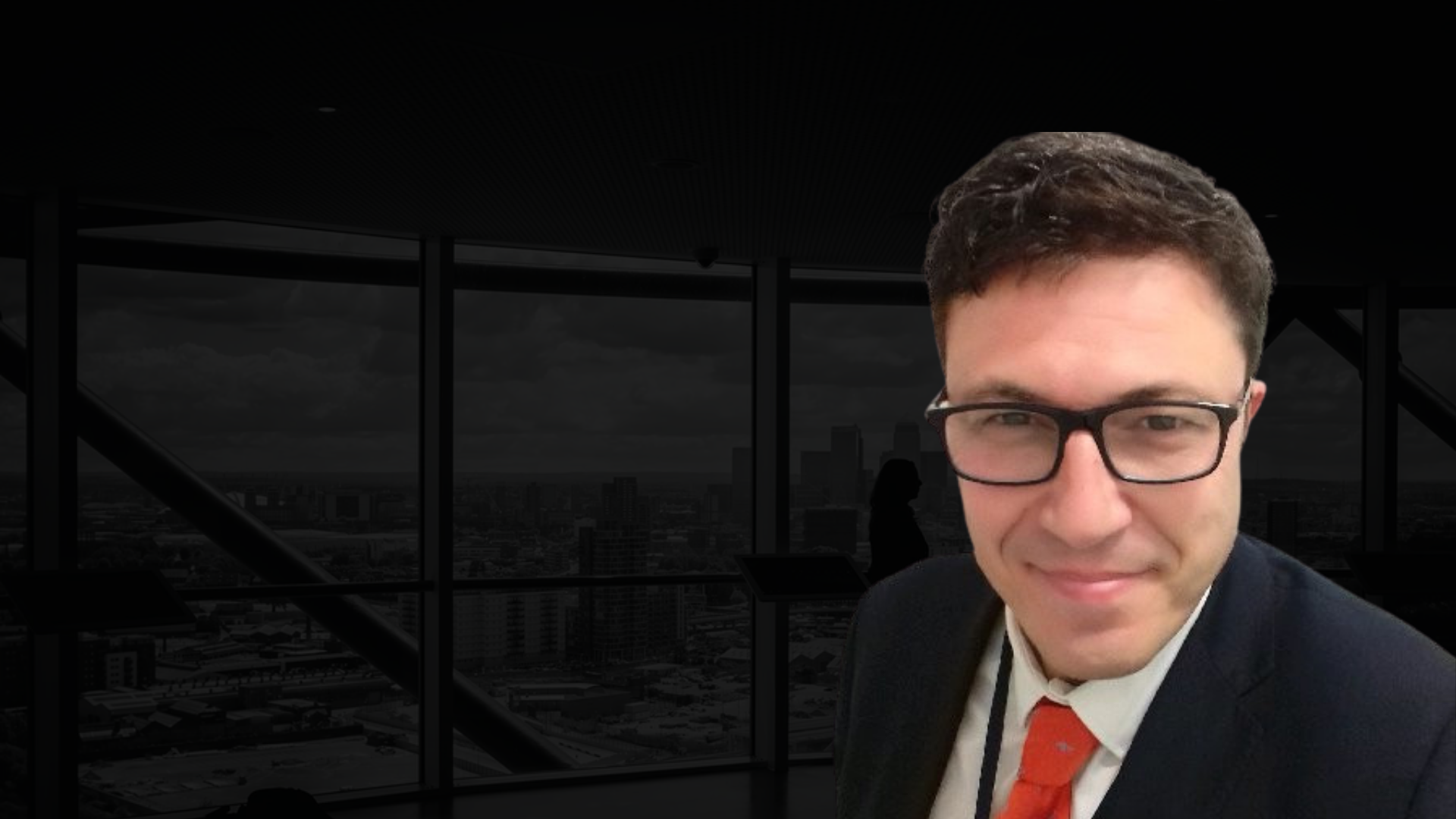
HSBC is modernizing its fraud and money laundering detection capabilities by rolling out algorithms designed to identify ‘bad apples’ in the most efficient way possible
Digital transformation is not unique to businesses. Since the onset of the COVID-19 pandemic, criminals have also moved their activities online.
Now, HSBC is using data to fight back. In this week’s episode of the Business of Data Podcast, HSBC’s Head of Data and Analytics and Business Financial Crime Risk Francisco Mainez explains the motivations behind this global initiative.
“We need to find something that will divert our attention to the customers that we really wanted to analyze. The ones that have the potential [to be] the bad apples in your basket,” says Mainez. “And also, that's going to have a knock-on effect on cost efficiency.”
Using Data to Identify High-Risk Customers
Historically, customer-focused assessments looking for fraud and money laundering might involve the manual review of thousands, if not tens of thousands of people and accounts. This process was costly, inefficient and time-consuming.
By employing an algorithm to give individual customers a personal score based on predetermined risk factors, HSBC can quickly identify high-risk accounts.
“What the algorithm does is embed different key risk indicators,” says Mainez. “Are you moving countries? Are you transacting with virtual currencies? Are you over a certain age?”
He continues: “In the old world, we will be looking for things that we know for a fact from previous experience that could be suspicious. With this [algorithm], you're scoring customers because you're actually measuring customer behavior.”
Improving the Efficiency of Fraud Investigations
By using data to identify the high-risk accounts, HSBC is making sure that their investigative resources are being used as efficiently as possible.
“You don't want to spend 80% of your time, energy, budget and resources, especially on the operation segment, checking a false positive. Then 20% of the time rushing to find out if those customers are the ones that you're looking for,” Mainez says. “We wanted to reverse that.”
He continues: We're going to spend minimal time because the machine is going to help us make a decision on which customers we need to review. And then we're going to spend the rest of the time properly analyzing the customers.”
Mainez points out that this initiative is designed to assist human decision-making, not replace it. The human element of fraud detection is still essential, especially when it comes to adapting a global initiative to local realities.
Taking Stock of Cultural Factors
Big financial institutions typically work in a very decentralized way. To make this global initiative successful, Mainez knew that the algorithm would have to take account of local and cultural factors.
“You also need to take into consideration cultural factors, he says. “Every country is going to have to worry about their own typologies, not the ones from [any other] country because that's going to produce false positives that they'll have to review”.
By asking individual regions to specify the cultural or regional typographies that best indicate risk, Mainez can tailor the algorithm to that region.
“You're going to tell me which are the typologies that are keeping you awake at night,” he says. We want to help you by configuring the system in a way that can detect those types of behaviors.”
The new initiative has already been rolled out in several regions, but the future has plenty in store for Mainez and his team.
“Over the next few months, we'll be deploying in more markets and continually tweaking those typologies,” Mainez says. “Because of all possible times, we started to roll this out in the middle of COVID.”
He concludes: “[Criminals] are adapting to a more digital and remote environment. That’s reflected in the data, and we need to be able to figure out how those typologies, and how they are evolving.”
Key Findings
- Algorithms are helping in the fight against fraud at HSBC. Customers are given a score based on their unique risk profile.
- It’s all about making fraud investigations more efficient. More efficiency means high-value people can focus on high-value tasks – it also may lead to cost savings.
- Understanding regional factors is key. In a decentralized environment like banking, it's crucial to factor in region-specific risks.