Value vs. Risk in an AI-Driven Business Environment: Dr. Ram Singh
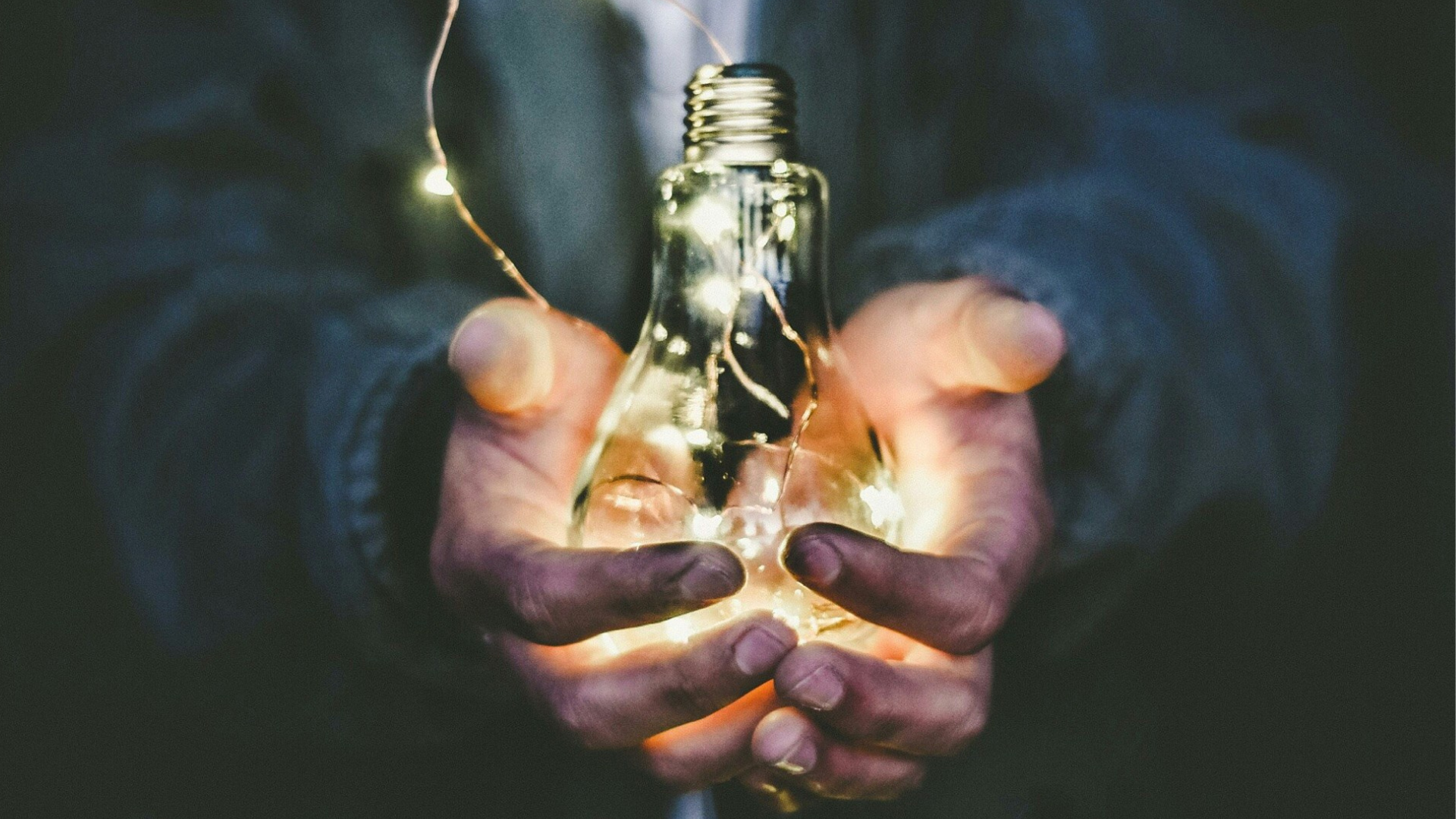
Dr. Ram Singh is the Chief Data Officer at Night Market, a Horizon Media company focusing on innovative, data-driven solutions to drive performance and digital transformation in the rapidly expanding commerce landscape. In his upcoming keynote at CDAO Chicago, Dr. Singh will explore how AI is changing the relationship between data and business and what it takes for CDOs and CDAOs to lead this transition effectively
As the Chief Data Officer at Night Market, Dr. Ram Singh spearheads initiatives to harness AI's full potential, ensuring data-driven insights lead to informed decision-making. His strategies emphasize the importance of data democratization, stakeholder involvement, and balancing value with risk.
Dr. Singh’s presentation at CDAO Chicago will delve into the critical responsibilities of CDOs and CDAOs in leading enterprise AI initiatives. He will highlight the importance of robust governance, comprehensive stakeholder engagement, and the strategic shift from cost efficiency to revenue generation.
Check out the full agenda and register for the event here.
What's your take on how AI is changing the relationship between data and business decision-making?
Dr. Ram Singh: As you know, data fuels AI, and we've all discussed the power of data in decision-making. However, until AI adoption became widespread, certain topics remained in the background.
The first thing that comes to mind is the scope of analytics we used to perform. Previously, we used smaller subsets of data due to the challenge of handling enterprise data and numerous descriptors.
Many of us have used ML models for a while, but the amount of data we utilized for decision-making was often limited by our resources and convenience. For instance, we might have access to 2,000 to 10,000 descriptors but end up using only a small, limited subset, or data that has been summarized already. The adoption of AI has empowered us to consider the full breadth of data. With the increased compute power, we can now process vast amounts of data that were previously discarded or unused.
For the first time, we are using the full potential of data, which is fundamental to good decision-making. The more data points we have, the richer the decision-making process becomes.
Another point is the confidence AI brings to decision-making. Previously, there was often a disconnect between decision-making and data. Decisions were based on model suggestions but still required a significant level of judgment.
With AI, especially complex models like neural networks with multiple layers, it becomes nearly impossible to manually analyze all possible combinations. AI processes the data for us, extracting learnings directly and dynamically, thereby reducing the need for manual summarization and judgment layers (not to be confused with governance, which is still required). This builds confidence in the actual findings derived from the data, which is beneficial for decision-making.
Moreover, the adoption of learning systems is increasing, further connecting data and decision-making. This is transforming how we think about and use data in business decisions.
What are some of the key challenges for organizations as they move from a more traditional data visualization approach to decision-making to automation and AI models?
Dr. Ram Singh: One of the big changes happening now is the shift from looking backward to looking forward. Traditional visualization relied on summarizing past information, extracting insights, and using that as the basis for future decisions. Now, we can adopt a go-forward approach. We no longer need to rely on data summaries. By feeding large volumes of data into machines, we can make real-time decisions without waiting for a stepwise approach to running past analysis and then dictating future actions. This forward-looking approach is exciting because it truly transforms our industry.
As I mentioned earlier, this shift also allows us to utilize data descriptors that we previously discarded. The ability to analyze a lot more data that wasn't used earlier is significant. With deep learning models, generative models, or computer vision models, we're not looking at months or weeks of analysis. We can feed the data, get findings, and move to the next step much quicker, even if we need to test the results. The pace of decision-making is much faster with these advanced solutions, which is a big advantage.
Modern AI tools seem to significantly amplify potential value, but they also introduce amplified risks. How do you think organizations can effectively manage these heightened benefits and risks?
Dr. Ram Singh: At the heart of it, I don't think this is a new problem. This is essentially a new way of posing the cost-benefit analysis question, which all of us have dealt with for a long time. In my view, it’s fundamentally a cost-benefit analysis. The application of AI has to be tailored to the right use case.
Let's say you’re using a generative AI model to sift through a lot of information. The most obvious and common application is customer service, such as analyzing conversation logs and chat logs to create more informed chatbots. They can directly leverage the power of generative AI.
However, even within this broad use case, there can be variations in risk levels. For instance, handling quick transaction-related conversations might be low risk. Conversely, using the same chatbot for high-stakes interactions, like pre-qualifying prospective students for educational programs, involves higher risk. A small bias in this context could turn potential customers away, and each lost enrollment could be worth tens of thousands of dollars.
The point here is that while the general use case might be the same, the risk level varies. When using AI, it’s crucial to have a robust list of use cases and perform a diligent analysis to identify where the risks might outweigh the benefits. This is why it's still a balancing exercise. Business owners must evaluate each use case, determining which has the highest potential for increasing revenue or lowering costs without introducing disproportionate risk. That’s the approach I advocate for managing these amplified value and risk factors.
Do you see most AI opportunities being in internal efficiency use cases, or are there broader applications?
Dr. Ram Singh: I'm actually a big fan of shifting use cases from cost efficiency to revenue generation. Your audience may have heard this from me before. While efficiency measures are important, they have a floor.
Over the last decade or so, even with the use of ML and advanced analytics, we have made many processes very efficient. By no means are we at the end of this. You can still automate, increase pace, and gain efficiency, but there's a limit to how much more you can squeeze out.
On the other hand, revenue-generation opportunities don’t have this constraint. AI applications that increase revenue, attract more customers, enhance transaction experiences, foster customer loyalty, or solve customer problems fall into this category.
These outward-facing business improvements offer significant potential. I'm a big fan of moving from cost containment use cases to business-enhancing use cases. Many of my colleagues believe in this approach as well.
That’s not to say there isn’t opportunity in efficiency gains, but those gains are becoming increasingly limited. If we can use AI, generative AI, computer vision, ML, or any intelligent application to drive business forward, that's where the real potential lies. Of course, this should always be done with an eye on managing risk.
With the advancement of AI models and increased AI usage, there's a growing need for robust AI governance frameworks within enterprises. What strategies or approaches do you recommend for organizations in this regard?
Dr. Ram Singh: It ties into the previous discussion about data democratization across organizations. We now have a powerful business proposition that necessitates democratizing and utilizing data organization-wide. I understand many organizations struggle with this due to siloed departments, legal constraints, budget issues, and competing priorities. However, the robust need to access and utilize all available data is more compelling than ever
One thing I'm observing from many partners, clients, and even within our organization is people voluntarily raising their hands to collaborate on building enterprise-level AI applications. Instead of each department using its own data silos, there's a shift towards developing applications that benefit the entire enterprise. This requires having a comprehensive list of use cases, some of which may be specific to a department while others are enterprise wide.
Data owners are now more willing to contribute to enterprise AI initiatives because everyone benefits from a successful application. It’s no longer a one-person or one-team show. An enterprise AI application motivates stakeholders to contribute their data, knowing that their department will share in the benefits.
To wrap up, it's crucial to have multiple stakeholders in an enterprise AI application. If you're working on a small-scale application, that’s one thing. But for enterprise-level initiatives, involving multiple stakeholders pays off. These stakeholders may be more open to contributing because they see the mutual benefits. These are the two main factors to consider: involve multiple stakeholders and recognize the shared benefits across the organization. Other reasons exist, but these are the key factors for robust AI governance.
As a leader, specifically a CDO or CDAO, what do you see as the most critical responsibilities in leading an enterprise AI initiative?
Dr. Ram Singh: Identifying all the different stakeholders in your organization is crucial because enterprise AI will require data from all parts of the organization. Having a comprehensive list of stakeholders who can contribute to the success of the initiative is essential.
I also highly recommend analyzing what data you own versus what you procure from other sources, whether it's purchased, third-party, or syndicated data. Owning a large part of the data that supports decision-making with your enterprise AI application puts you in a much better position. Using procured or syndicated data, without having your own significant data to stitch to it or complement it, can limit the customization and effectiveness of your AI solution in terms of highly tailored decisions specifically for your business needs.
Understanding the talent profile within your organization is also important because every setup is different. Not everyone on your team needs to be a data scientist, but you can't do this without having some data science experts actively working on the problem.
At the enterprise level, you'll need data detectives (analysts), data engineers, infrastructure people, and system integrators. You will need GTM strategists to help built practical use cases that have a high demand in the marketplace. You will need data scientists to build out the complex models with all the inputs listed earlier. Furthermore, you will need team members who can help identify the pros and cons of use cases and assess the value versus the risk.
Considering the scrutiny in this area, it's important to involve the right compliance person to ensure all regulatory and ethical standards are met. So, to summarize, the critical responsibilities include:
1. Having a comprehensive list of stakeholders.
2. Understanding what data you own versus what you acquire.
3. Assessing the talent profile within your organization.
4. Involving compliance personnel.
5. Differentiating between efficiency-driven and revenue-driven use cases.
These are the top considerations that come to mind for leading a successful enterprise AI initiative.
Want to learn more?
Dr. Ram Singh is speaking at CDAO Chicago, kicking off on August 7th, 2024. Join him and many more world-class data and analytics leaders to learn more about the data and analytics trends driving value creation. Register to attend here.