Data Architecture Expert Nilabh Sahai: What AI Demands of Data
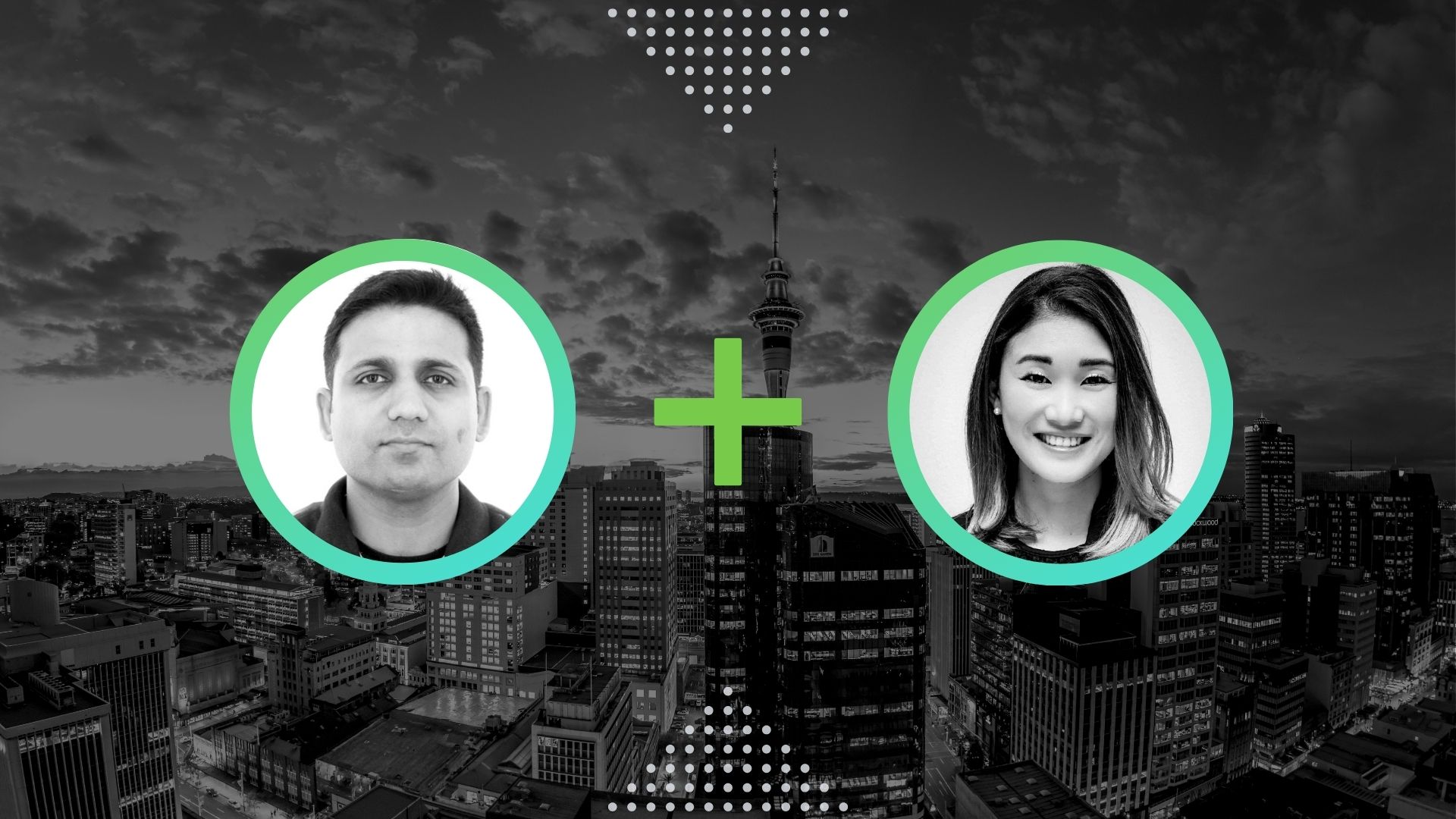
Data architecture expert, Nilabh Sahai, spoke to Corinium APAC Conference Director Maddie Abe about the intersection of data architecture and AI and ML workflows
In the dynamic landscape of artificial intelligence (AI) and machine learning (ML), the significance of robust data architecture cannot be overstated.
In order to produce reliable insights and forecasts, ML models are dependent on significant volumes of quality data, and that data needs to be managed and delivered.
This pivotal role of data architecture in facilitating AI and ML applications is well understood by Nilabh Sahai, data architecture expert.
“Data architecture-defined standards and governance approaches can be extended to the AI/ML initiatives,” Sahai says.
“The standards defining data cleansing, normalisation, and validation processes ensure the data used for training AI/ML models is accurate, consistent, and reliable.
“Governance helps maintain data integrity, privacy, and compliance with regulations, which are critical in the AI/ML space both from the legal and compliance perspective.”
Discussing the foundational components of robust data architecture for AI and ML workflows, Sahai underscores the importance of a well-architected data platform.
"One of the core foundations of any AI/ML is to have a well-architected data platform that can deliver the data needs of the AI/ML models,” he says.
“This will often include some key components such as scalable storage, for data lakes, purpose-built services, and controls for seamless data ingestion, preparation, and movement."
Scalability and Performance
"One of the components of the data architecture is to have a robust data processing capability," Sahai emphasises.
"AI/ML requires clean and accurate data to deliver a better result. Feature engineering can be defined as a process to manipulate the dataset to improve machine learning model training to get better performance and accuracy."
Addressing strategies and technologies for ensuring scalability and performance, Sahai shared some crucial points.
“The architecture can adopt strategies like the use of distributed computing, running workloads in parallel (parallelisation), using case-based efficient data ingestion (batch/streaming), and scalable and efficient storage capabilities (including distributed storage and cloud storage services), data compression, partitioning, and monitoring,” he says.
Sahai also elaborates on how real-time data processing fits into data architecture for AI/ML applications.
"To enable real-time decision-making, AI/ML requires data to be available and accessible in the data platform for the model’s uses in real-time," he says.
Data Storage and Retrieval
Similar to other data-driven activities, the success of AI and ML hinges on efficiently ingesting and storing data for various purposes, including model training and feature engineering.
This necessitates the ability to swiftly onboard data and manage it in cost-effective and optimised manners to support future use cases.
“The overall performance of AI/ML models can be influenced by the way data is accessed or made available, the data access speed and the storage type/format. Faster data retrieval enables faster model training and deduction,” Sahai says.
“Columnar storage formats like Parquet and ORC (Optimized Row Columnar) are optimised for analytical queries and improve data retrieval speed by reading only the required columns."
Data Quality and Governance
Regarding data quality maintenance and governance measures, Sahai emphasises the importance of clean and accurate data for reliable AI/ML outcomes.
"Any analytical or AI/ML model requires clean and accurate data in volume to publish a trusted result," he says.
Sahai underscores the role of data quality checks, model validation techniques, and governance frameworks in ensuring data reliability and transparency.
“The governance framework can be used to implement the data quality checks validating and monitoring AI/ML models to ensure their reliability, fairness, and transparency,” he says.
“The framework includes capabilities such as model validation techniques, model performance monitoring, bias detection, and model explainability methods to understand and mitigate potential risks and biases in model predictions.”
Adapting to Evolving Models and Technologies
Regarding adapting data architectures to evolving AI/ML models and emerging technologies, Sahai emphasises the importance of flexibility, scalability, and modular design.
"Data architecture plays an important part in building a modern-day platform using cutting-edge technologies that can help deliver and handle the data requirements of analytics, data science and AI in a more efficient way with cost-effectiveness,” he says.
“To run AI/ML initiatives requires a flexible and scalable approach and a strategy that can help build a design that can be easily scaled up/down and work with modular design.”
Challenges and Lessons Learned:
In reflecting on common challenges and valuable lessons learned in implementing data architectures for AI/ML, Sahai emphasises the multifaceted nature of building platforms to support these initiatives, including data quality, scalability and performance, organisational mindsets and governance.
"Issues with the quality of data impact the model’s results significantly," Sahai mentions first and foremost.
“When it comes to scalability and performance, platforms that are not scalable and performant struggle to support the performance requirements of AI/ML workloads.”
As for organisational mindsets, Sahai warns that a lack of literacy in the AI/ML space stops organisations from comprehending the value of such applications. He emphasises the need for organisations to foster a culture of understanding and appreciation for AI/ML to fully realise its potential.
Lastly, a lack of governance can put an initiatives into cold storage," Sahai says.
"Comprehensive governance, both in terms of data and AI/ML, is absolutely necessary to ensure the success and sustainability of initiatives in this rapidly evolving landscape.”
Sahai's insights underscore the pivotal role of data architecture in advancing AI and ML initiatives. By tackling challenges like data quality, scalability, organizational mindset, and governance, businesses can effectively leverage AI technologies to address complex problems and drive innovation.
With a robust data architecture in place, organisations can unlock the full potential of AI and ML, paving the way for transformative solutions and sustainable growth in the digital age.
Nilabh Sahai will be speaking at Data Architecture New Zealand this April. To learn more and register your place, visit this link!