Business of Data Meets: Duke University Health System CAO Stephen Blackwelder
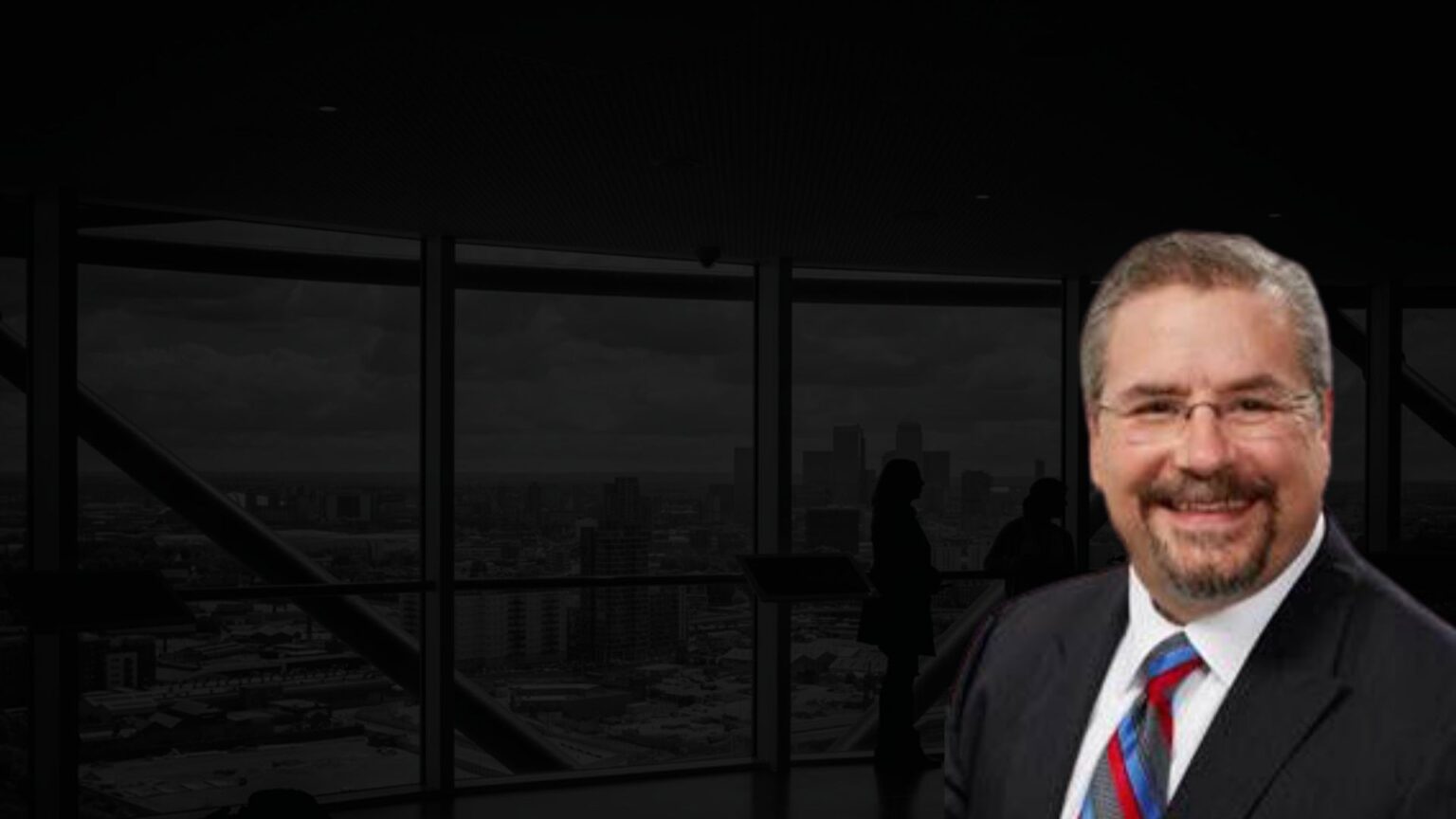
To celebrate being named one of the world’s top analytics leaders, Duke University Health System CAO Stephen Blackwelder talks about his professional achievements, his plans for 2020 and the future of data science in the healthcare industry
What were your greatest professional achievements in 2019?
The thing that I’m most proud of over the past year has been the establishment of a holistic training program for folks that are distributed throughout the organization.
As an academic medical center, we don’t have all the analysts neatly cordoned off in a little unit with a VP over them. They’re in departments and academic settings. They’re in different elements of the health system reporting up to different VPs and different siloed aspects of the organization.
Despite this, we endeavor to support all our analytics efforts with a consistent data and metrics base. We refer to this as ‘enterprisifying’ the independent projects – providing consistent data and model curation and deployment that maximizes model effectiveness while limiting accumulation of technical debt.
For example, we support our data science development with a data science lifecycle platform that allows containerized models to interact with a robust microservices infrastructure. That is still maturing, but it has already made a huge difference in terms of reliability and repeatability, allowing people to scale their data science efforts throughout the organization.
How will you build on those foundations through 2020?
What I was just describing is very foundational. At the ‘rubber meets the road’ end of things, we’ve had some consolidation of the leadership around the actual implementation of data science.
So, in place of the ‘beauty contest’ approach we started out with years ago – where if somebody could find a sexy clinical problem to pursue, they went off and started modeling it – we’re now seeing more rigorous alignment to the strategy of the organization.
We’ll see this play out over the next year, reflected in the organization around the way new projects get prioritized, the way they get onboarded, the way resources are assigned and with further maturation of our lifecycle platform.
Just like everybody else, we have limited resources and making the best use of them is important. What we’re now beginning to see, through ‘enterprisifying’ our data, our governance and through some other organizational changes, is the ability to consistently apply those resources in alignment with our organizational strategy to advance health and healthcare.
How you think data leadership roles are changing? And how you think they should change over the months and years ahead?
I’m beginning to see non-data science folks realizing that, if we’re going to be successful in data science, we need curation of the data.
They’re saying, ‘Let me step into this project and I’ll bring some analytical support to it, we’ll work with the clinicians, the researchers or the SMEs and we’ll curate some data for the project.’
One of the things that we’re focused on here at Duke is, how do we allow that kind of work to proceed? Because it’s obviously got to be done by many hands. So, how do we bring all these projects together such that, if we’re doing curation in project A, then projects B and C can also benefit from it where it makes sense.
I think CDAOs are increasingly finding that that’s a challenge they need to pursue, so that the organization doesn’t end up with that horrible cliché of ‘multiple sources of the truth’.
So, what should CDAOs be doing to facilitate these projects and the curation of these datasets?
Well, nor do we want to waste all our energies building subtly different versions of exactly the same thing. So, there’s definitely that data governance component here.
I’m not talking about what we saw a few years ago, where everybody in the marketplace was giddy for data dictionaries or cataloging. Here, I’m talking more about a methodology for data engineering through pipelines allowing for some reusability.
There’s this concept from software engineering called CICD [continuous integration, continuous development] that we’re seeing being applied in data management. It’s where you build your methodological approach in a coded way so that it’s reproducible. You can go in and make a change to the code and you don’t have to go back in and make a change to the table.
Data management is in flux, and if we can harness some of these capabilities to make it more transparent, reproducible and reliable, then we have a real opportunity to be able to deliver information into the organization at scale in a way that would really be unprecedented.
If you were to imagine how the data and analytics space will look like at the very end of 2020, what do you think will have changed compared to how things are today?
Right now, we’ve got a host of folks who are willing to come and do data science for you, essentially as hired guns. I believe that’s going to thin. Many of those organizations are relatively small. They’ve got some venture backing, they’re looking to find a niche and I believe there’ll be some shakeout over the course of 2020.
There’s been an initial flush on the part of health systems, with people saying, ‘Hey, we’ve got to get in on data science and AI. We need to spend some money on it.’ I believe that flush is beginning to pass and we’re not going to see health systems, by and large, willing to invest large proportions of their budget on vendor data science.
So, folks out in the marketplace are going to have to figure out ways to apply value into health in a different way.