What Happened When MoneySupermarket Embraced DataOps
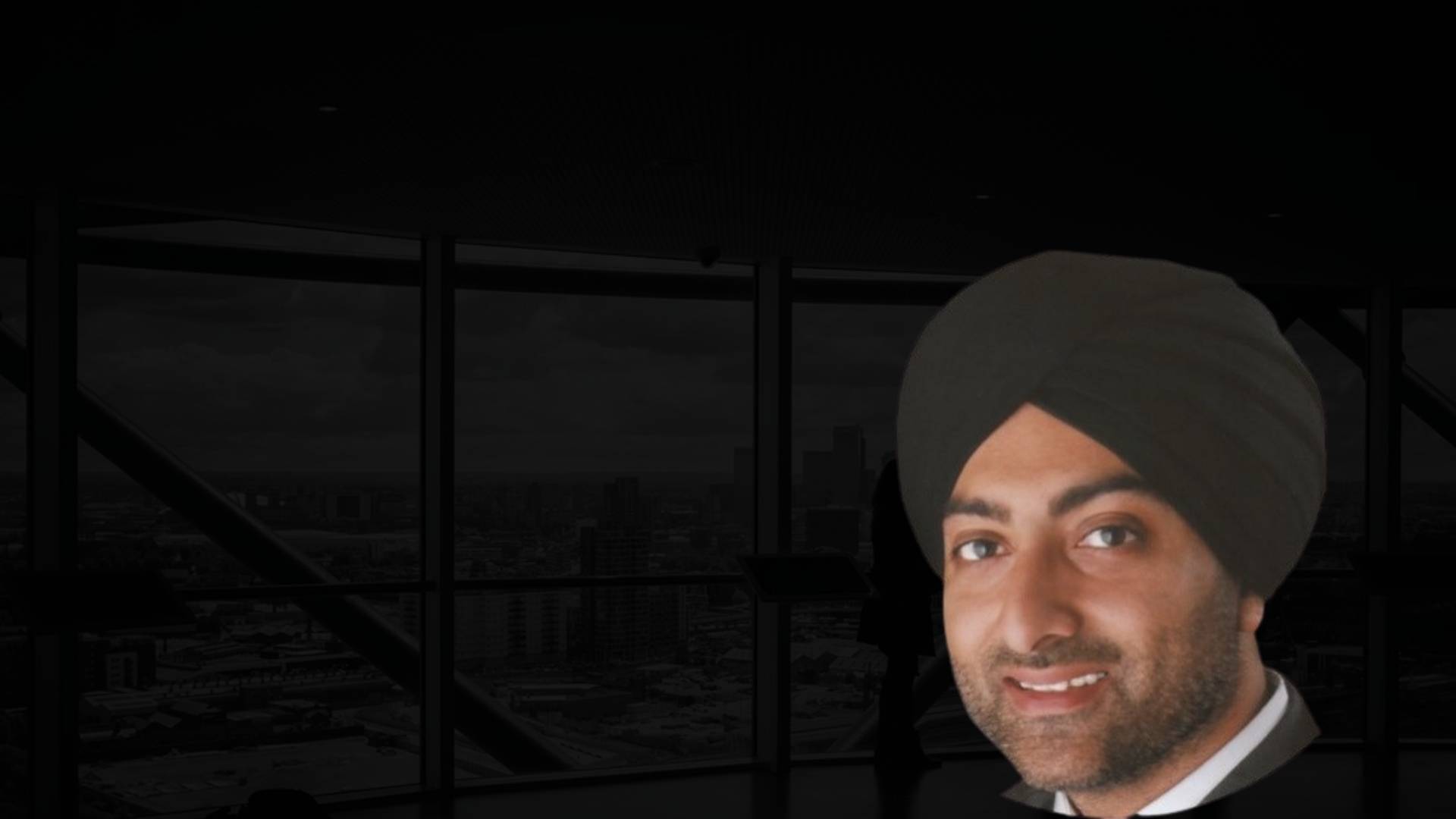
Harvinder Atwal, Group Data Director at MoneySupermarket, shares how DataOps principles have dramatically enhanced the productivity of the group’s data function
Adopting DataOps practices has helped MoneySupermarket’s data function drive significant productivity gains in recent years. The results have been so good, MoneySupermarket Group Data Director Harvinder Atwal decided to chronicle his experiences in his book, Practical DataOps: Delivering Agile Data Science at Scale.
In this episode of the Business of Data podcast, he outlines the key principles that define DataOps and shares how adopting a ‘data products’ mindset is helping his team drive business results more effectively.
“For us, DataOps is data analytics – in its broadest sense, including data science and AI – combined with ‘lean thinking’,” he explains. “The creation of data products is key."
What DataOps and DevOps Have in Common
There are two strands to the DataOps concept of ‘lean thinking’. One is about looking at processes, making them more efficient and adapting to change. The other is DevOps.
Atwal explains that DevOps has its roots in the historic tension between software developers and operations professionals. While developers want to innovate and improve applications, this can create challenges for operations people, who need to make sure things run in production reliably.
“The challenge was that you get to a place where the operations people are maintaining a really brittle product in production,” he explains. “DevOps is there to make sure that these things [don't] happen.”
Instead of developing apps as giant ‘monoliths’, DevOps breaks them down into independent constituent parts. These can then be iterated rapidly to incrementally improve their performance.
Harvinder notes that a key step in applying ‘lean thinking’ principles to data and analytics is making the switch from a ‘project’ to a ‘product’ mindset. Rather than starting with data and trawling it for insights, data teams should start with a ‘desired outcome’ and go from there.
“Traditionally, the way people have approached using data is to think about actionable insights,” he says. “So, ‘What can we find in the data that will produce some insights and create a recommendation?’”
“It’s about flipping everything on its head,” he continues. “We’ll take an outcome and say, ‘What kind of data product can we build that will deliver that outcome?’”
How to Apply DataOps to Model Development
To illustrate how DataOps can improve data team efficiency, Atwal outlines how MoneySupermarket applied these principles to streamline marketing model development and reduce the latency of its model scoring.
“We used to have long data and development cycles around models,” he recalls. “We looked at that end-to-end to say, ‘Where are the bottlenecks?’”
He continues: “We broke up the entire model scoring pipeline into lots of different steps and the model training pipeline into lots of different steps, and then introduced automation and reproducibility.”
“What reproducibility allows your to do is to be able to test things in a development environment and then, if you pass the test, you deploy it in the production environment,” he notes. “That can lead to quite a high level of automation.”
“You can also work on different parts of the pipeline in parallel,” he adds. “So, rather than working on one giant code base, you’re able to split it up and just make changes in one place, without having to affect others.
In this way, DataOps has helped Atwal's team create faster, more robust processes for marketing model development. But the story doesn’t end there. One final difference between a product and a project is that a product has no ‘end’.
His team will continue to improve the data products in their portfolio, continuously iterating and integrating new capabilities to drive better outcomes for the business in the months ahead.
Key Takeaways
- Adopt a ‘data products’ mindset. Data teams should start with a business challenge and design a data product that achieves a predefined desired outcome
- Streamline the data product pipeline. Use ‘lean thinking’ principles to find bottlenecks in existing business processes and find ways to make the data pipeline more efficient
- Continuously integrate; continuously develop. Rapidly iterate data products to add in new features, reduce model scoring latency and drive better business outcomes