Three Ways 2020 Transformed How Investors Use AI
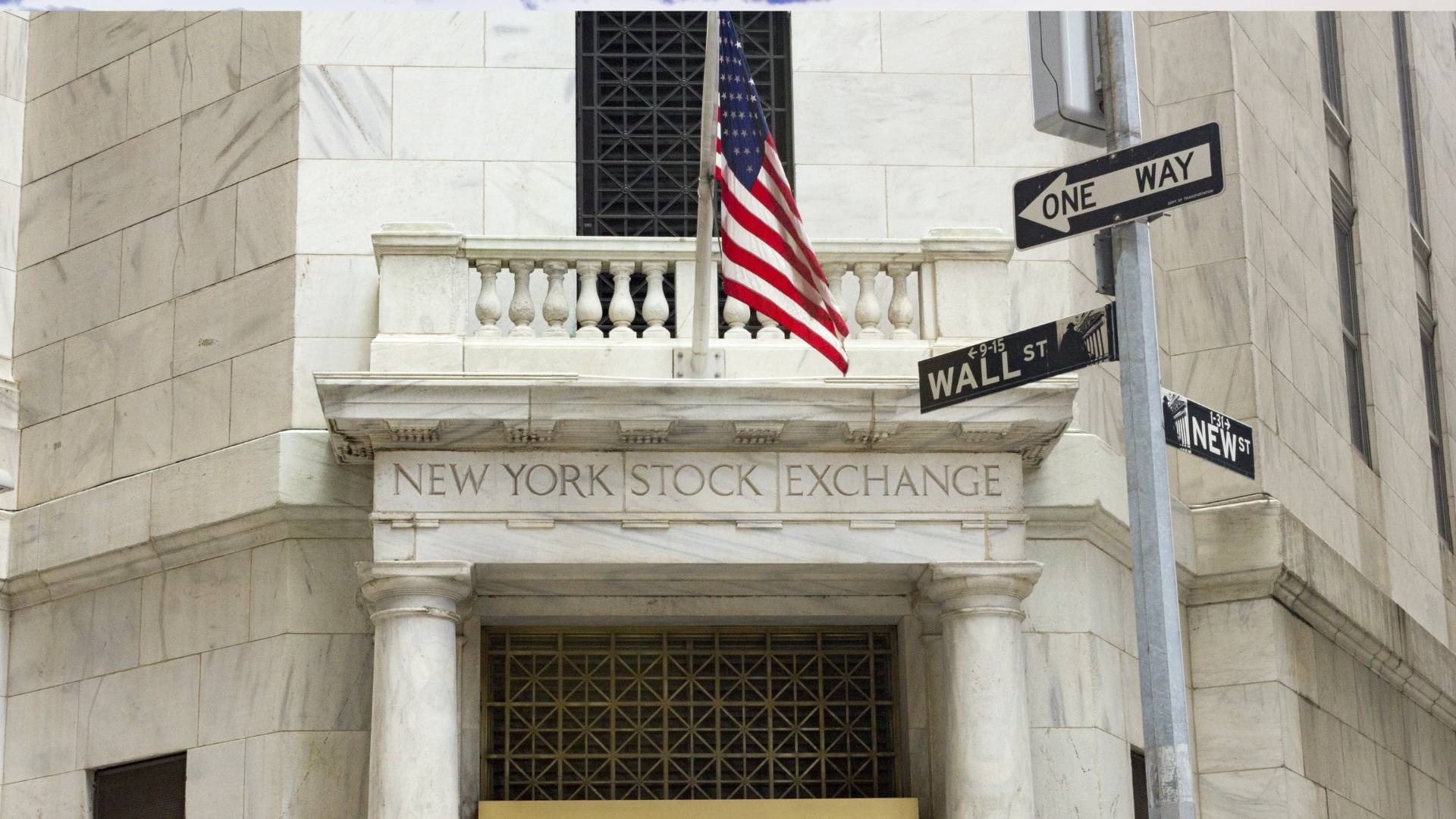
From the limitations of ‘quantamental’ investing to the rise of explainable AI and alternative data, Corinium’s AI Champions, Online: Trading & Investment event highlighted this year’s top trends in data-driven asset management
AI leaders from top American financial services and investment firms gathered to share ideas at Corinium’s AI Champions, Online: Trading & Investment virtual summit this month.
The three-day event provided a forum for AI leaders in this space to evaluate what’s been successful and how they’ve overcome the unique challenges they’re facing today.
“We’ve always had statistical models,” said Aishwarya Venkatesan, Data Science and Analytics Lead for Global Trading at BlackRock. “But now, with data-driven AI models, those are augmenting the experience more. So, even with the same problems, you’re able to glean more insights.”
COVID-19 Exposed the Limitations of ‘Quantamental’ AI
As AI methodology has evolved, quantamental investing has been at the forefront of the industry. This hybrid of quantitative and fundamental investing involves using mathematics, finance or trading experience to develop ideas about exploitable market anomalies.
However, the recent market fluctuations COVID-19 triggered have shown that this may not always be the best approach. As Adrian de Valois-Franklin, CEO of Castle Ridge Asset Management observed, the past few months have shown that machine learning (ML) based on historical data can be inaccurate in unforeseen circumstances.
“A lot of people are talking about buzzword machine learning techniques like neural networks and deep learning in this space,” he said. “Our feeling is that these are generally ‘black box’ strategies that are too static.”
He added: “[They’re] great for teaching your cell phone how to recognize your face and unlocking itself or a computer to play chess. But the problem becomes, what if the rules of chess change at every turn?”
To be truly predictive, AI systems need to adapt to unknown environments and constantly evolve. Castle Ridge Asset Management has developed one such self-evolving AI system, which enabled them to come through the market crash not just unscathed but thriving.
“Where most strategies tend to be ‘book smart’, they’re trying to learn from the past and assuming that those types of behaviors are going to continue,” he said. “We had to develop an AI methodology that was actually ‘street smart’, able to enter an unknown environment and quickly adapt to it.”
Alternative Data has been Crucial in 2020
Once the quality of the data ML models are ingesting has been ensured, alternative data is a key resource to improve predictions. Recent market uncertainty has really brought this to the forefront of strategy for many firms. It’s increasingly viewed as a necessity to keep pace with the competition.
Mackenzie Hargrave, VP, Product Strategist at FactSet, shared that while the software company has always been interested in alternative data, she has seen the attitudes of its clients shift in the past 6-9 months.
“We’ve seen a little bit more openness to it,” she said. “I think this is out of a need to navigate the uncertainty around the pandemic and respond to increased adoption of alternative data across financial firms and their competitors.”
One of her fellow panelists, George Mylnikov, VP, Head of Quantitative Research at Charles Schwab, acknowledged the challenges involved with integrating these new data sources across the organization.
“What’s applicable to bonds is not necessarily applicable to stocks or commodities or currencies, yet you have to model them all together,” he said. “You need to pick your poison. Either stay with old data, old standard methodologies or, if you want to go with some of the new data, new methodologies, that might become a bit less intuitive.”
Hargrave added: “The alternative data needs to still deliver value in the long-term and be informative in different investment environments, in part because there’s still that high cost of ownership [and] acquisition of new content.”
Finance Professionals Want Explainable AI
Explainable AI remains of the utmost importance in the industry. AI-focused executives must be transparent with internal and external stakeholders about the possible impacts or risks associated with new technologies.
In fact, our own research shows that ‘explainability’ is the characteristic executives value most when developing AI systems, ahead of ‘predictiveness’, ‘transparency’ and ‘accuracy’.
“The explainability required by those novel markets is the top challenge for AI models,” Sophie Chen, Senior Data Scientist at Nasdaq observed. “It’s really challenging for us to balance the model-driven decision making versus the expert-driven decision making and we need to find the sweet spot. I’m pretty sure going forward, as more and more of those types of markets are emerging, we will see this problem get resolved.”
“I think when we say ‘better performing’ [models], that term needs some thought,” echoed Venkatesan. “Is it better performing in every scenario? Because when you’re asked about a scenario where it did not perform well, how are you going to explain it?”
“Explainability is so key to how we get adoption of AI in finance,” Venkatesan concluded. “I think that motivation also comes from the clients, and rightly so. They want to know what’s affecting their portfolios.”